Application of artificial neural networks in horizontal luminous efficacy modeling
Renewable Energy(2022)
Abstract
Harnessing daylight for energy-efficient designs requires the availability of daylight illuminance data. In the absence of measured daylight data, deriving daylight using the luminous efficacy model is an alternative. This paper presents an approach for modeling diffuse and global luminous efficacies using accessible measured climatic data. The methodology explored machine learning, sensitivity analysis and empirical modeling approaches. Twelve (12) luminous efficacy models were proposed. These models consisted of six artificial neural networks (ANN) and six empirical models. All models also cover the all-sky, overcast and non-overcast sky conditions. The intent of using ANN lies in the need for more accurate daylight predictions and its ease in explaining complex relationships between complex atmospheric variables. Findings from the study show that diffuse fraction is crucial in global and diffuse luminous efficacy modeling. Furthermore, the performance of all models was statistically assessed, and the results show that all twelve proposed models could offer acceptable predictions of daylight. In particular, the ANN models outperformed the empirical models.
MoreTranslated text
Key words
Daylighting,Luminous efficacy,Machine learning,Empirical model,Sensitivity analysis
AI Read Science
Must-Reading Tree
Example
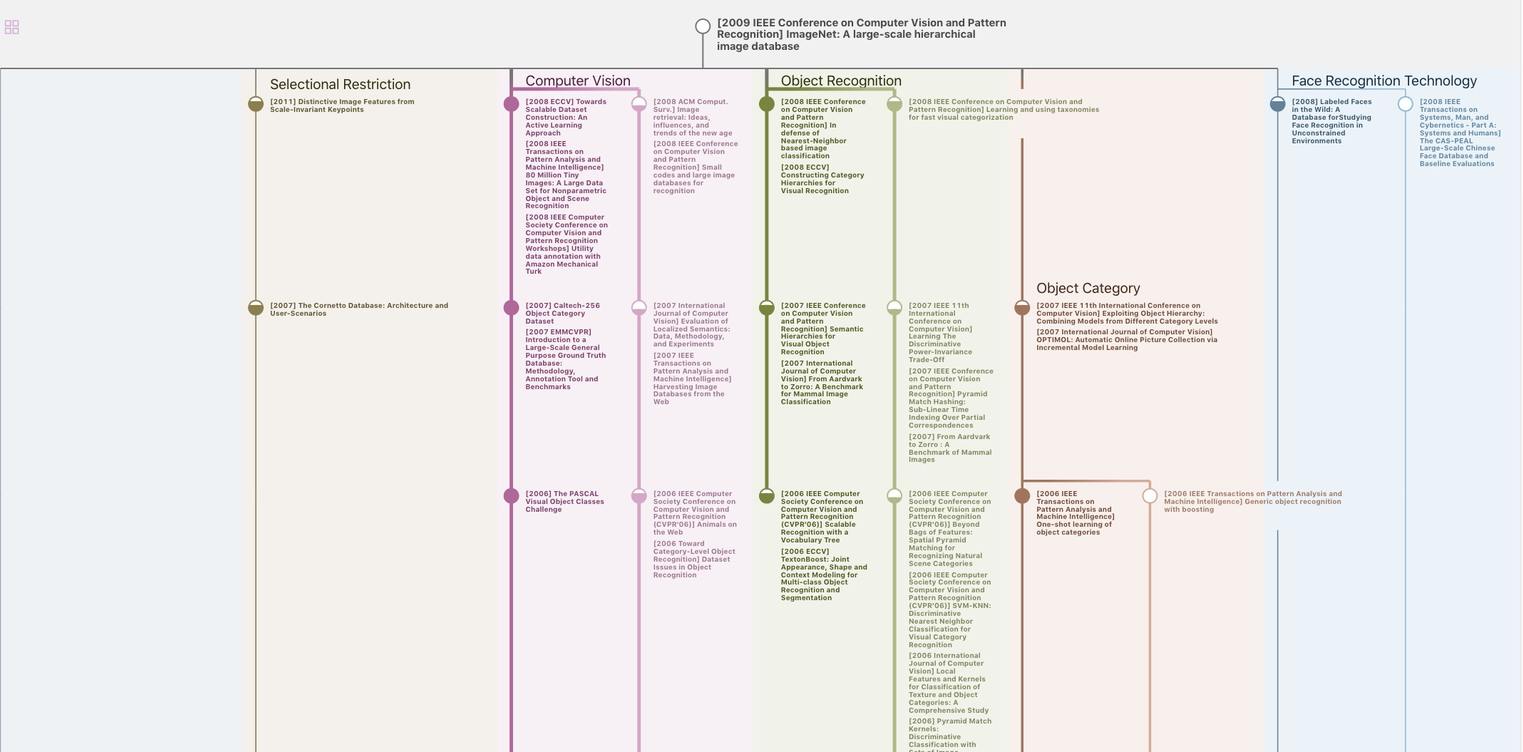
Generate MRT to find the research sequence of this paper
Chat Paper
Summary is being generated by the instructions you defined