Machine Learning-Based Fragment Selection Improves the Performance of Qualitative PRM Assays
JOURNAL OF PROTEOME RESEARCH(2022)
摘要
Targeted mass spectrometry-based platforms have become a valuable tool for the sensitive and specific detection of protein biomarkers in clinical and research settings. Traditionally, developing a targeted assay for peptide quantification has involved manually preselecting several fragment ions and establishing a limit of detection (LOD) and a lower limit of quantitation (LLOQ) for confident detection of the target. Established thresholds such as LOD and LLOQ, however, inherently sacrifice sensitivity to afford specificity. Here, we demonstrate that machine learning can be applied to qualitative PRM assays to discriminate positive from negative samples more effectively than a traditional approach utilizing conventional methods. To demonstrate the utility of this method, we trained an ensemble machine learning model using 282 SARS-CoV-2 positive and 994 SARS-CoV-2 negative nasopharyngeal swabs (NP swab) analyzed using a targeted PRM method. This model was then validated using an independent set of 200 positive and 150 negative samples and achieved a sensitivity of 92% relative to results obtained by RT-PCR, which was superior to a traditional approach that resulted in 86.5% sensitivity when analyzing the same data. These results demonstrate that machine learning can be applied to qualitative PRM assays and results in superior performance relative to traditional methods.
更多查看译文
关键词
machine learning (ML), parallel reaction monitoring (PRM), COVID-19, sensitivity, limit of detection (LOD), antigen detection
AI 理解论文
溯源树
样例
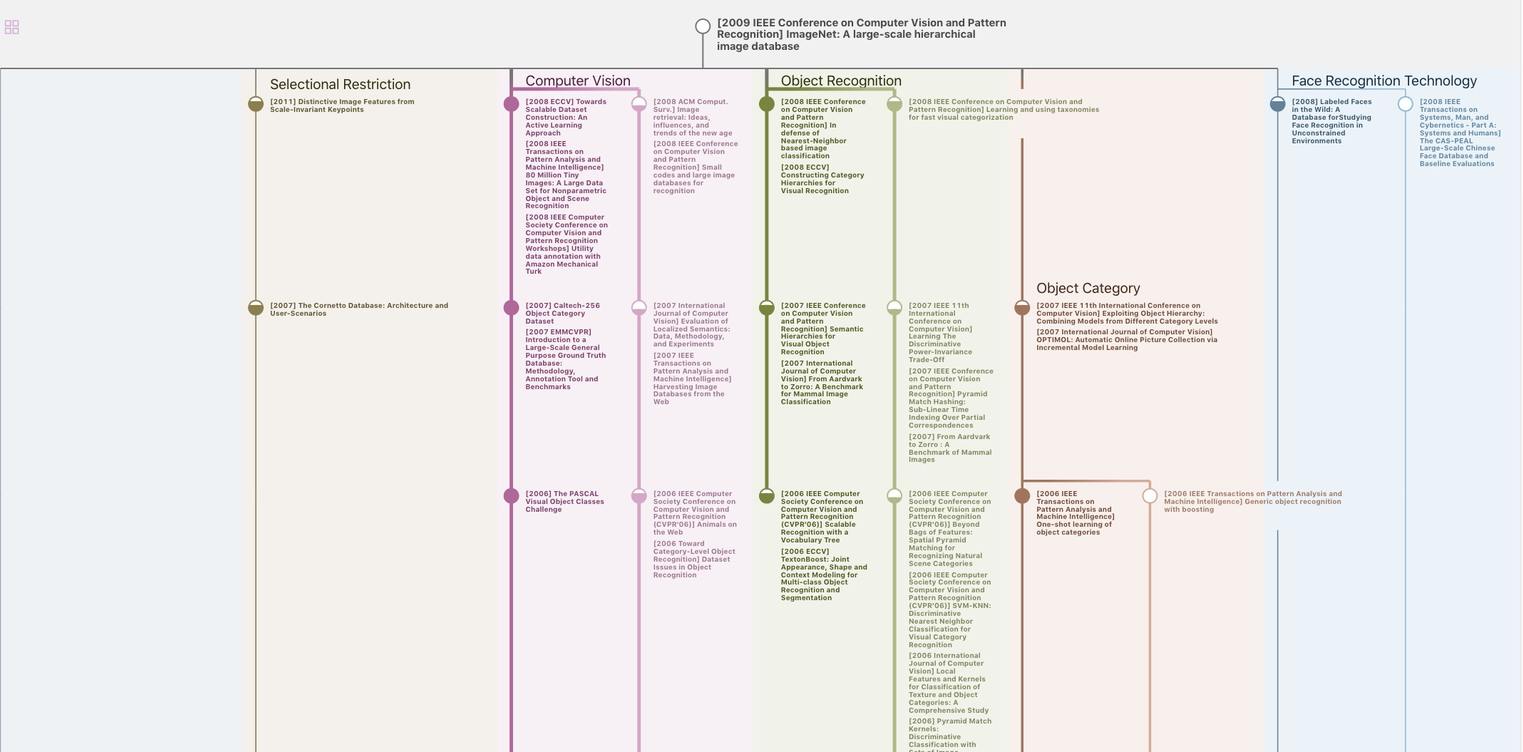
生成溯源树,研究论文发展脉络
Chat Paper
正在生成论文摘要