Piecewise forecasting of nonlinear time series with model tree dynamic Bayesian networks
INTERNATIONAL JOURNAL OF INTELLIGENT SYSTEMS(2022)
摘要
When modelling multivariate continuous time series, a common issue is to find that the original processes that generated the data are nonlinear or that they drift away from the original distribution as the system evolves over time. In these scenarios, using a linear model such as a Gaussian dynamic Bayesian network (DBN) can result in severe forecasting inaccuracies due to the structure and parameters of the model remaining constant without considering either the elapsed time or the state of the system. To approach this problem, we propose a hybrid model that combines a model tree with DBNs. The model first divides the original data set into different scenarios based on the splits made by the tree over the system variables and then performs a piecewise regression in each branch of the tree to obtain nonlinear forecasts. The experimental results on three different datasets show that our model outperforms standard DBN models when dealing with nonlinear processes and is competitive with state-of-the-art time series forecasting methods.
更多查看译文
关键词
dynamic Bayesian networks,forecasting,piecewise regression,regression trees,time series
AI 理解论文
溯源树
样例
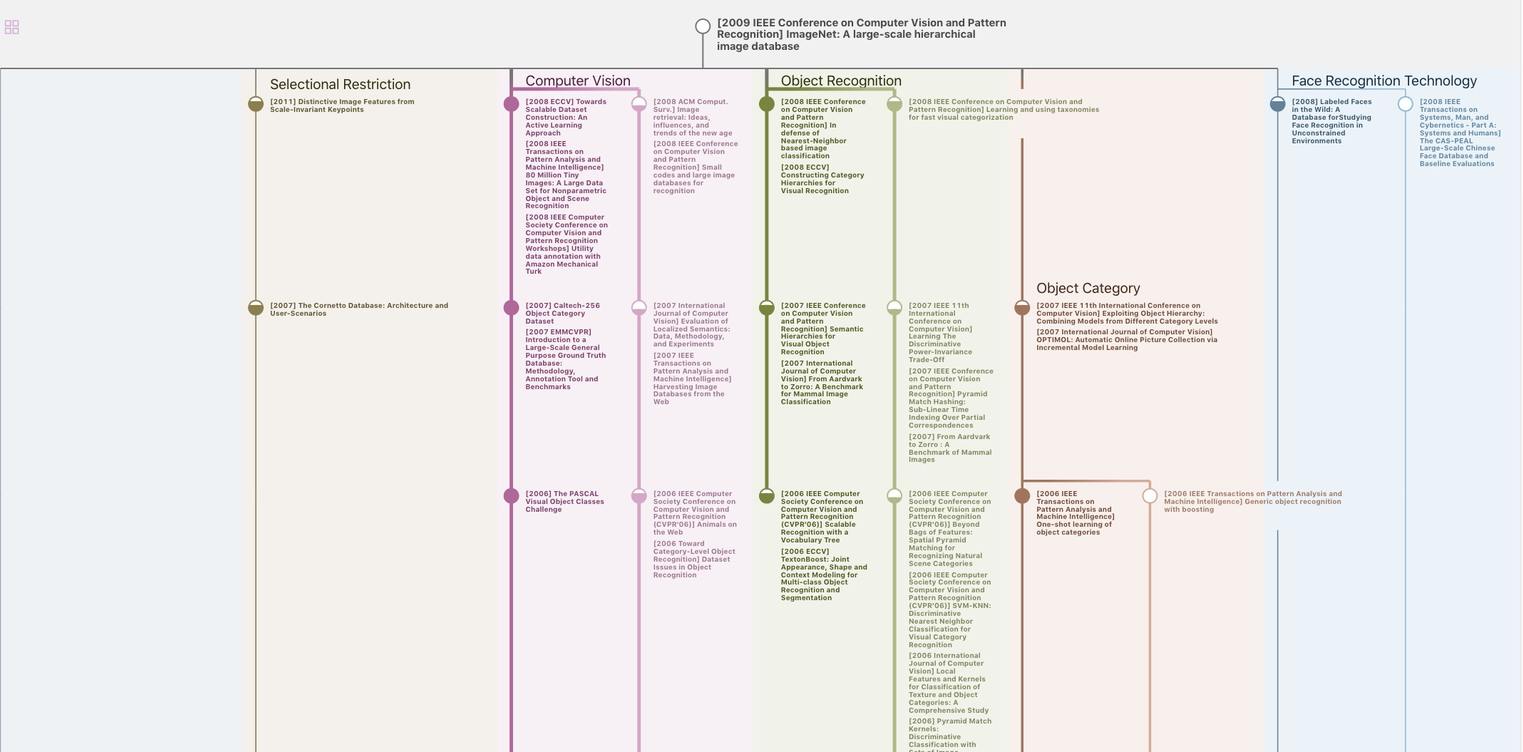
生成溯源树,研究论文发展脉络
Chat Paper
正在生成论文摘要