Small Is Beautiful: Compressing Deep Neural Networks for Partial Domain Adaptation
IEEE TRANSACTIONS ON NEURAL NETWORKS AND LEARNING SYSTEMS(2024)
Abstract
Domain adaptation is a promising way to ease the costly data labeling process in the era of deep learning (DL). A practical situation is partial domain adaptation (PDA), where the label space of the target domain is a subset of that in the source domain. Although existing methods yield appealing performance in PDA tasks, it is highly presumable that computation overhead exists in deep PDA models since the target is only a subtask of the original problem. In this work, PDA and model compression are seamlessly integrated into a unified training process. The cross-domain distribution divergence is reduced by minimizing a soft-weighted maximum mean discrepancy (SWMMD), which is differentiable and functions as regularization during network training. We use gradient statistics to compress the overparameterized model to identify and prune redundant channels based on the corresponding scaling factors in batch normalization (BN) layers. The experimental results demonstrate that our method can achieve comparable classification performance to state-of-the-art methods on various PDA tasks, with a significant reduction in model size and computation overhead.
MoreTranslated text
Key words
Training,Computational modeling,Task analysis,Adaptation models,Deep learning,Taylor series,Supervised learning,Deep learning,neural network compression,transfer learning
AI Read Science
Must-Reading Tree
Example
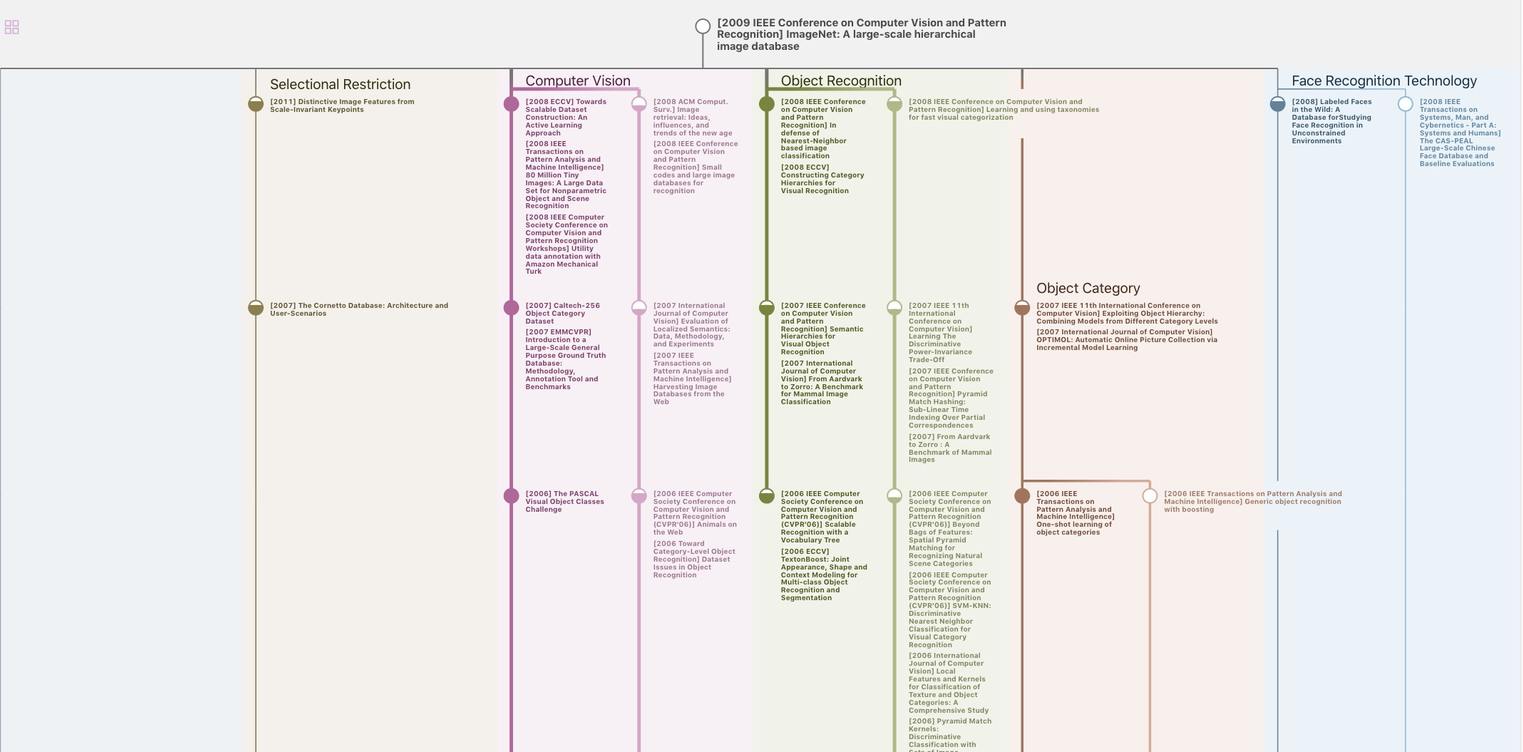
Generate MRT to find the research sequence of this paper
Chat Paper
Summary is being generated by the instructions you defined