A correlatively sparse lagrange multiplier expression relaxation for polynomial optimization
arXiv (Cornell University)(2024)
Abstract
In this paper, we consider polynomial optimization with correlative sparsity. We formulations for polynomial optimization problems using the Karush-Kuhn-Tucker optimality conditions. Correlatively sparse sum-of-squares (CS-SOS) relaxations are applied to solve the CS-LME reformulation. We show that the CS-LME reformulation inherits the original correlative sparsity pattern, and the CS-SOS relaxation provides sharper lower bounds when applied to the CS-LME reformulation, compared with when it is applied to the original problem. Moreover, the convergence of our approach is guaranteed under mild conditions. In numerical experiments, our new approach usually finds the global optimal value (up to a negligible error) with a low relaxation order for cases where directly solving the problem fails to get an accurate approximation. Also, by properly exploiting the correlative sparsity, our CS-LME approach requires less computational time than the original LME approach to reach the same accuracy level.
MoreTranslated text
Key words
polynomial optimization,correlative sparsity,Lagrange multiplier expressions,Moment-SOS relaxations
AI Read Science
Must-Reading Tree
Example
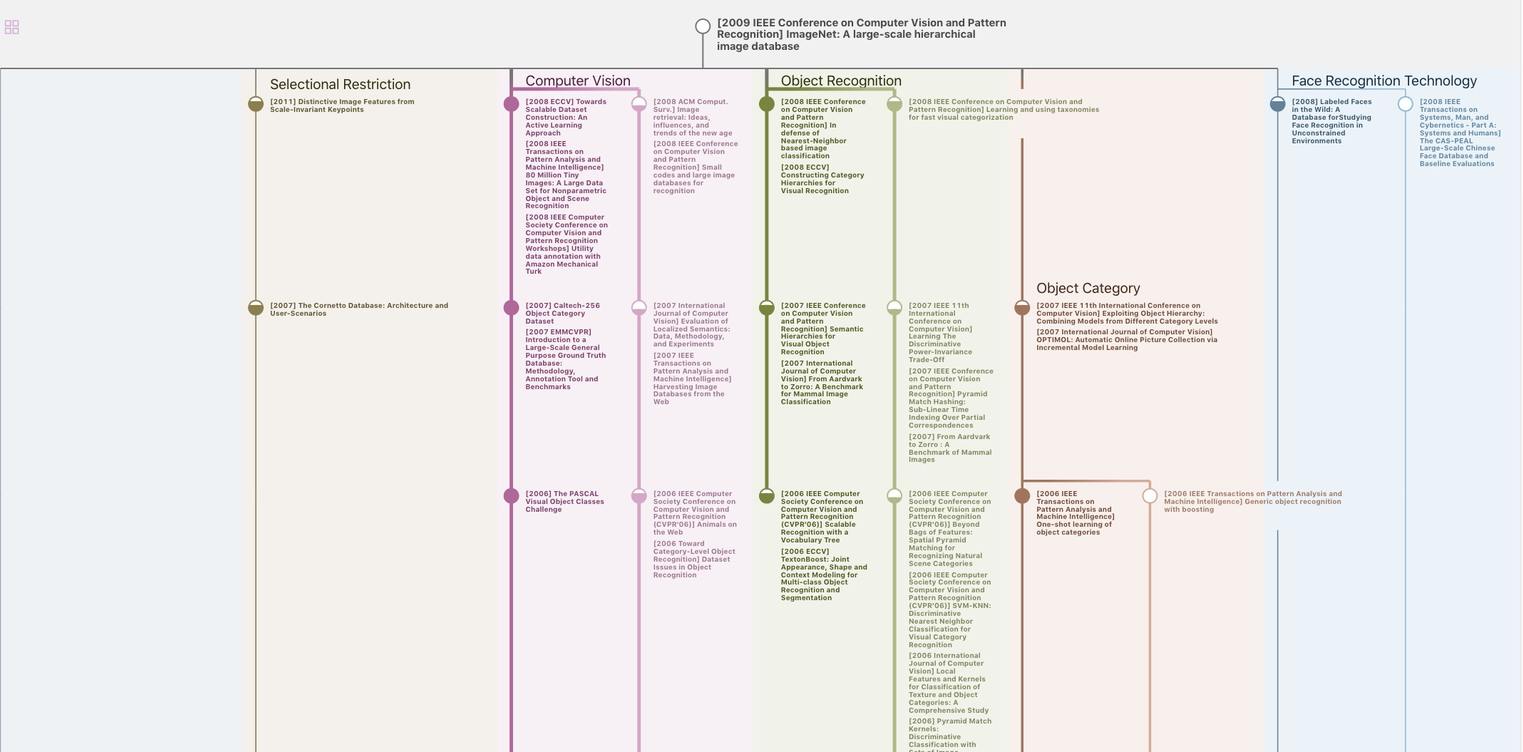
Generate MRT to find the research sequence of this paper
Chat Paper
Summary is being generated by the instructions you defined