Dataset Obfuscation: Its Applications to and Impacts on Edge Machine Learning
arxiv(2022)
摘要
Obfuscating a dataset by adding random noises to protect the privacy of sensitive samples in the training dataset is crucial to prevent data leakage to untrusted parties for edge applications. We conduct comprehensive experiments to investigate how the dataset obfuscation can affect the resultant model weights - in terms of the model accuracy, Frobenius-norm (F-norm)-based model distance, and level of data privacy - and discuss the potential applications with the proposed Privacy, Utility, and Distinguishability (PUD)-triangle diagram to visualize the requirement preferences. Our experiments are based on the popular MNIST and CIFAR-10 datasets under both independent and identically distributed (IID) and non-IID settings. Significant results include a trade-off between the model accuracy and privacy level and a trade-off between the model difference and privacy level. The results indicate broad application prospects for training outsourcing in edge computing and guarding against attacks in Federated Learning among edge devices.
更多查看译文
关键词
dataset,edge machine
AI 理解论文
溯源树
样例
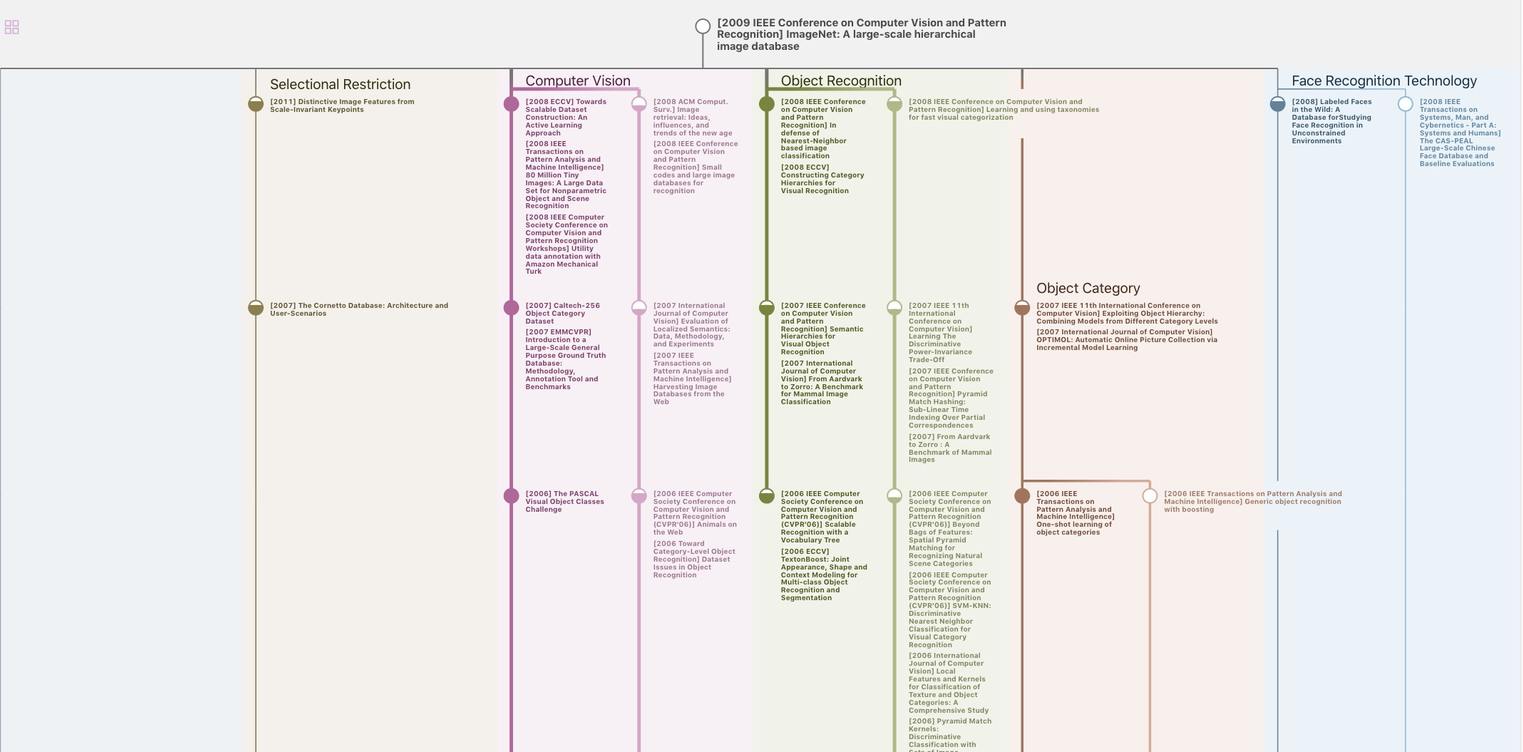
生成溯源树,研究论文发展脉络
Chat Paper
正在生成论文摘要