Sampling QCD field configurations with gauge-equivariant flow models
Proceedings of The 39th International Symposium on Lattice Field Theory — PoS(LATTICE2022)(2022)
Abstract
Machine learning methods based on normalizing flows have been shown to address important challenges, such as critical slowing-down and topological freezing, in the sampling of gauge field configurations in simple lattice field theories. A critical question is whether this success will translate to studies of QCD. This Proceedings presents a status update on advances in this area. In particular, it is illustrated how recently developed algorithmic components may be combined to construct flow-based sampling algorithms for QCD in four dimensions. The prospects and challenges for future use of this approach in at-scale applications are summarized.
MoreTranslated text
Key words
qcd field configurations,flow,gauge-equivariant
AI Read Science
Must-Reading Tree
Example
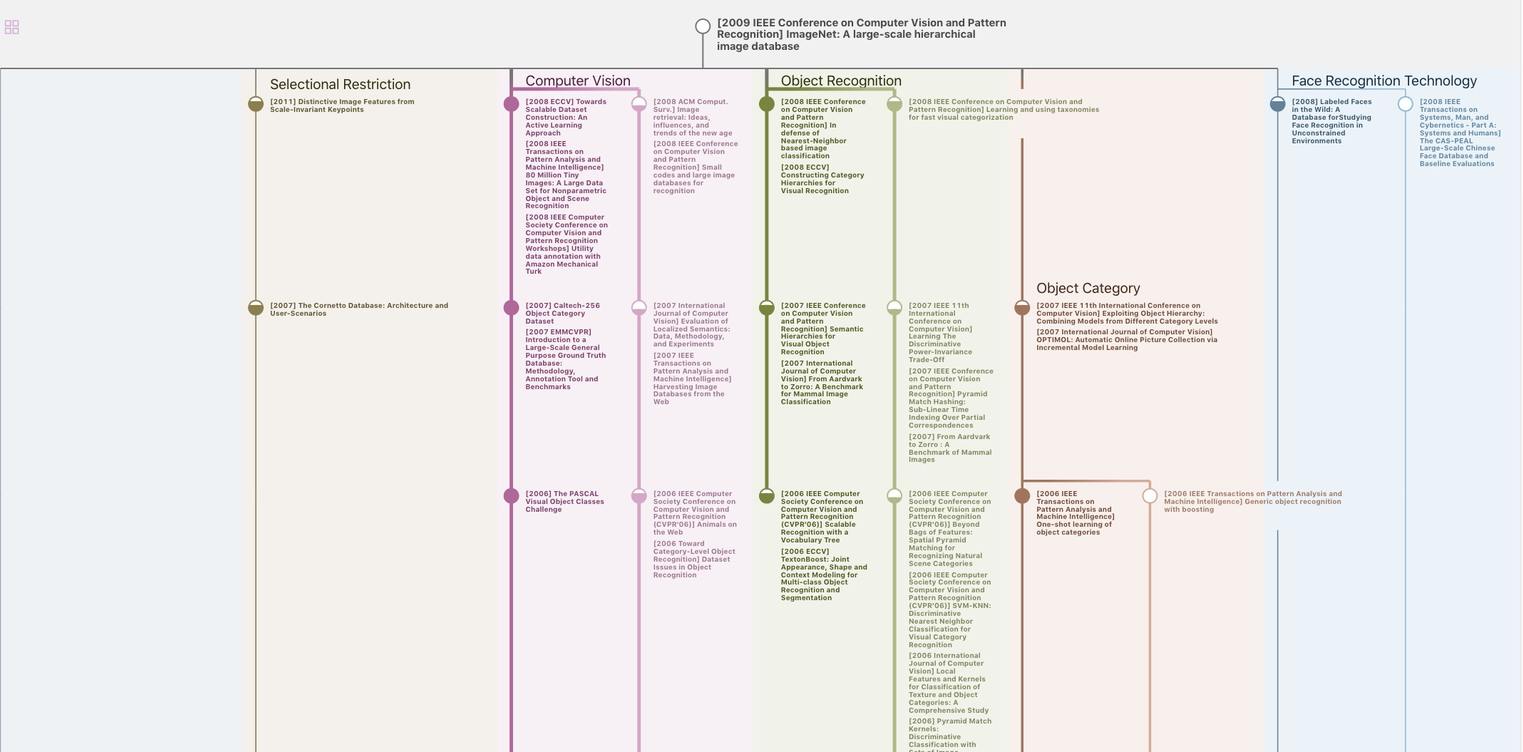
Generate MRT to find the research sequence of this paper
Chat Paper
Summary is being generated by the instructions you defined