Tpm: transition probability matrix-graph structural feature based embedding
Kybernetika(2023)
Abstract
In this work, Transition Probability Matrix (TPM) is proposed as a new method for extract-ing the features of nodes in the graph. The proposed method uses random walks to capture the connectivity structure of a node's close neighborhood. The information obtained from random walks is converted to anonymous walks to extract the topological features of nodes. In the embedding process of nodes, anonymous walks are used since they capture the topological sim-ilarities of connectivities better than random walks. Therefore the obtained embedding vectors have richer information about the underlying connectivity structure. The method is applied to node classification and link prediction tasks. The performance of the proposed algorithm is superior to the state-of-the-art algorithms in the recent literature. Moreover, the extracted information about the connectivity structure of similar networks is used to link prediction and node classification tasks for a completely new graph.
MoreTranslated text
Key words
graph representation learning,feature learning,link prediction,node classification,anonymous random walk
AI Read Science
Must-Reading Tree
Example
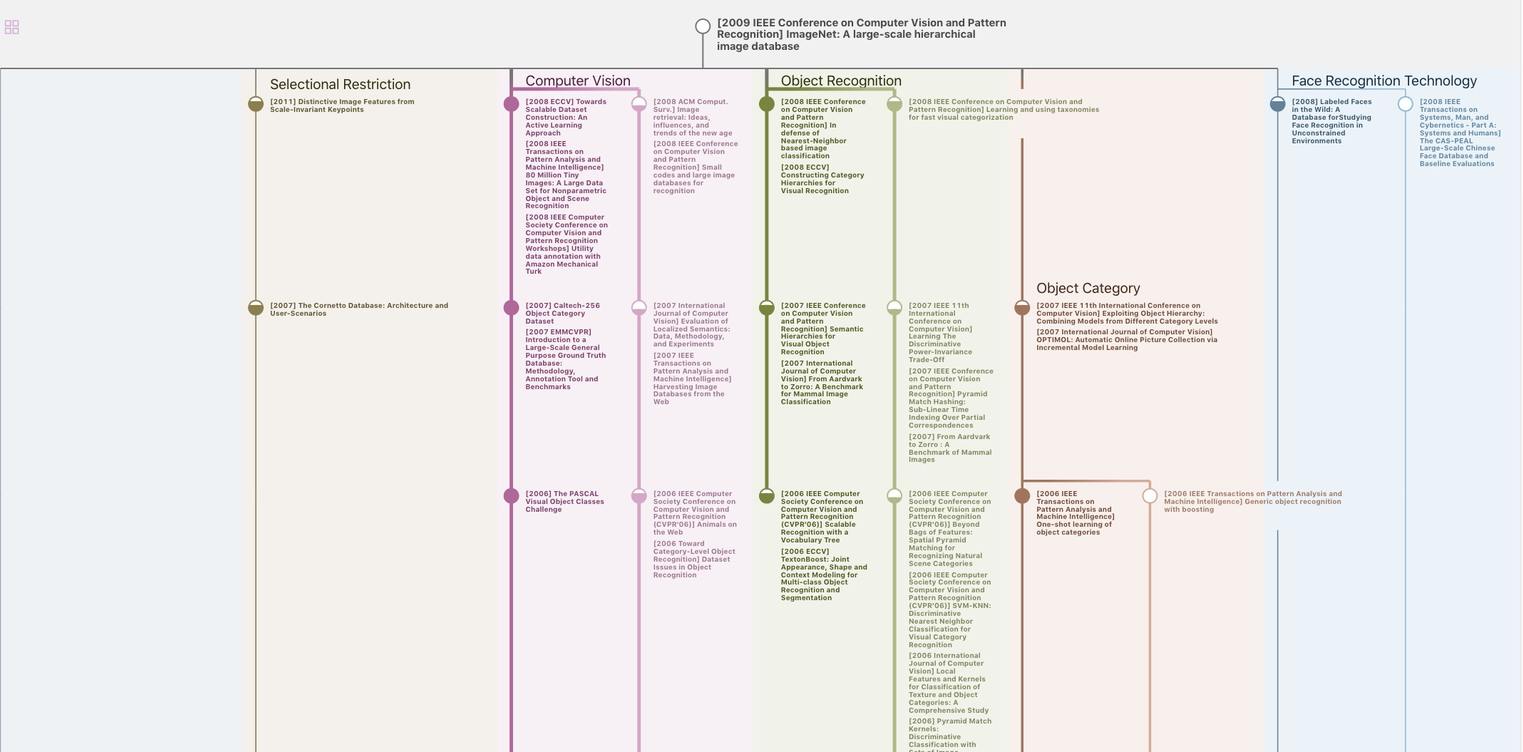
Generate MRT to find the research sequence of this paper
Chat Paper
Summary is being generated by the instructions you defined