Generalized estimators, slope, efficiency, and fisher information bounds
Information Geometry(2024)
摘要
Point estimators may not exist, need not be unique, and their distributions are not parameter invariant. Generalized estimators provide distributions that are parameter invariant, unique, and exist when point estimates do not. Comparing point estimators using variance is less useful when estimators are biased. A squared slope Λ is defined that can be used to compare both point and generalized estimators and is unaffected by bias. Fisher information I and variance are fundamentally different quantities: the latter is defined at a distribution that need not belong to a family, while the former cannot be defined without a family of distributions, M . Fisher information and Λ are similar quantities as both are defined on the tangent bundle TM and I provides an upper bound, Λ≤ I , that holds for all sample sizes—asymptotics are not required. Comparing estimators using Λ rather than variance supports Fisher’s claim that I provides a bound even in small samples. Λ -efficiency is defined that extends the efficiency of unbiased estimators based on variance. While defined by the slope, Λ -efficiency is simply ρ ^2 , the square of the correlation between estimator and score function.
更多查看译文
AI 理解论文
溯源树
样例
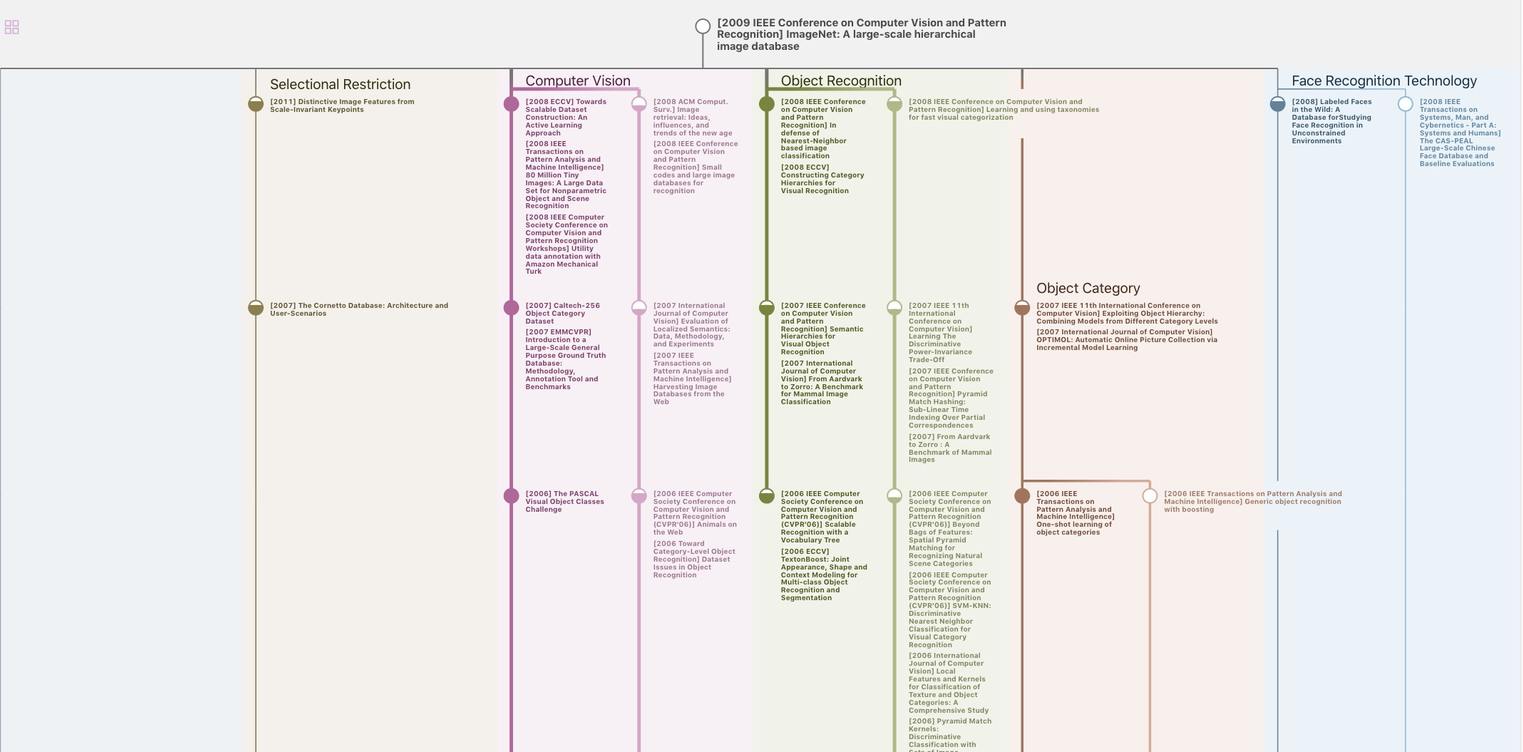
生成溯源树,研究论文发展脉络
Chat Paper
正在生成论文摘要