Multicommunity Graph Convolution Networks with Decision Fusion for Personalized Recommendation
ADVANCES IN KNOWLEDGE DISCOVERY AND DATA MINING, PAKDD 2022, PT III(2022)
Abstract
Most graph-based recommendation approaches actually have made an implicit assumption about that representations of all users and all items can be learned in a single latent space. However, this assumption may be too strong to well describe a single use's multifaceted preferences each probably dominated by some latent type of motivations. This paper challenges this assumption and proposes a MultiGCN model (Multicommunity Graph Convolution Networks with Decision Fusion) to leverage multiple latent spaces for capturing multiple types of motivation. Specifically, we first design a community exploration module to construct multiple communities so as to explore different latent types of motivation. We next design a local recommendation module which maps the representations of entities in each community into one latent space and outputs a local recommendation list. A decision fusion module reranks the items of local lists to obtain the final recommendation list. Experiment results on three real-world datasets demonstrate that our MultiGCN outperforms the state-of-the-art algorithms.
MoreTranslated text
Key words
Recommender system, Graph convolution network, Community exploration, Random walk
AI Read Science
Must-Reading Tree
Example
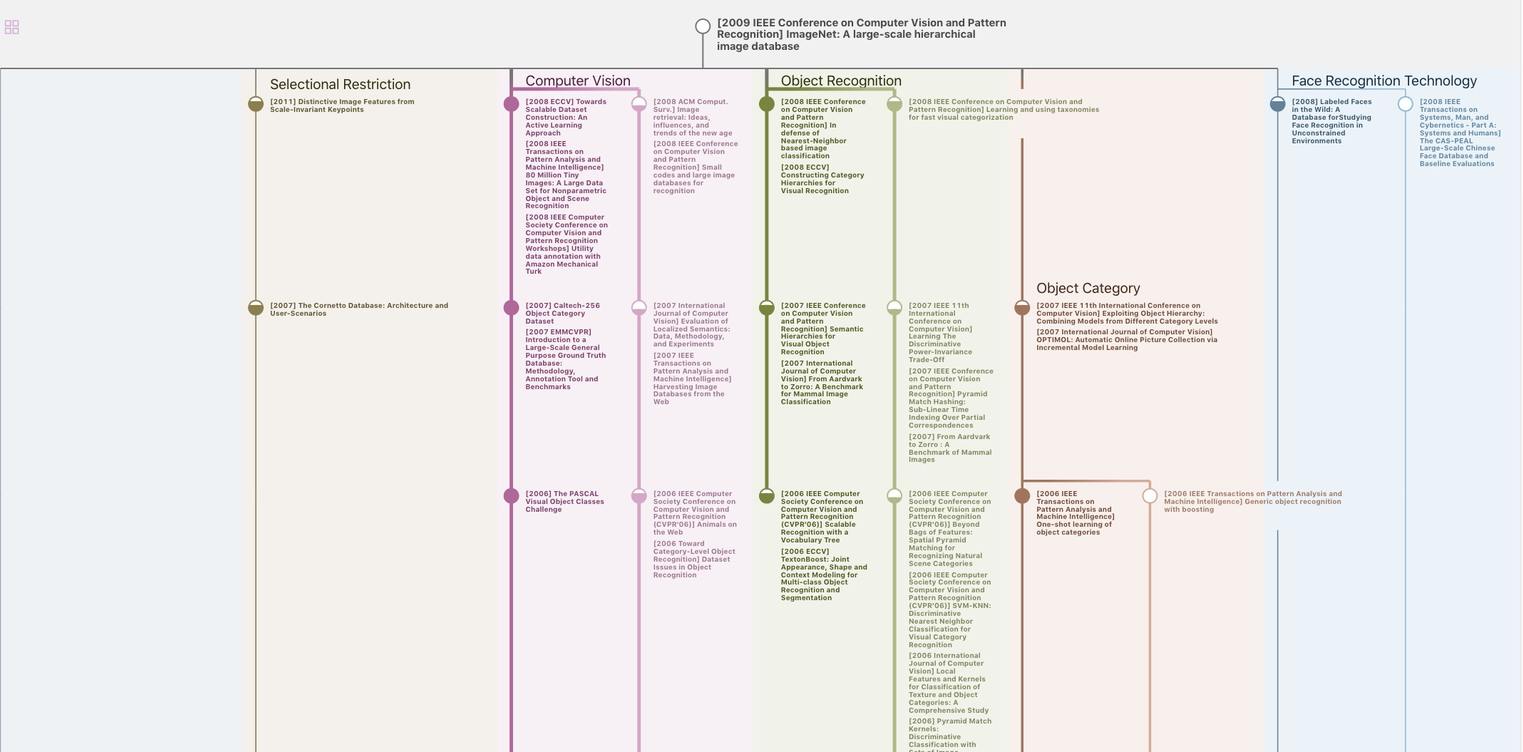
Generate MRT to find the research sequence of this paper
Chat Paper
Summary is being generated by the instructions you defined