Wangiri Fraud: Pattern Analysis and Machine-Learning-Based Detection
user-61447a76e55422cecdaf7d19(2023)
摘要
The rapid growth of the telecommunication landscape leads to a rapid rise of frauds in such networks. In this article, Wangiri fraud in which users are deceived by being charged for services without their knowledge during a call is tackled. In fact, Wangiri fraud has significant negative financial and reputation consequences for the mobile service providers and also has a bad psychological impact on the victims. In order to identify this fraudulent behavior, three Wangiri fraud patterns are defined by analyzing call records of over a year. Then, the security and performance of unsupervised and supervised machine learning (ML) methods in detecting one Wangiri pattern are evaluated using a large real-world Call Detail Records (CDRs) data set. In the context of Wangiri fraud detection, classification algorithms outperformed the others based on the chosen security and performance metrics. Finally, the performance evaluation of these algorithms is extended in detecting the other two real-world Wangiri fraud patterns. This article provides a detailed definition of the Wangiri fraud patterns and outlines the implementation and evaluation of ML algorithms in the context of detecting Wangiri fraud. The security analysis and experimental results demonstrate that depending on fraud patterns the best ML algorithm to detect Wangiri fraud may also vary.
更多查看译文
关键词
Communications technology,Oral communication,Costs,Classification algorithms,Internet of Things,Security,Machine learning algorithms,Machine learning (ML),pattern analysis,telecommunication fraud,Wangiri fraud
AI 理解论文
溯源树
样例
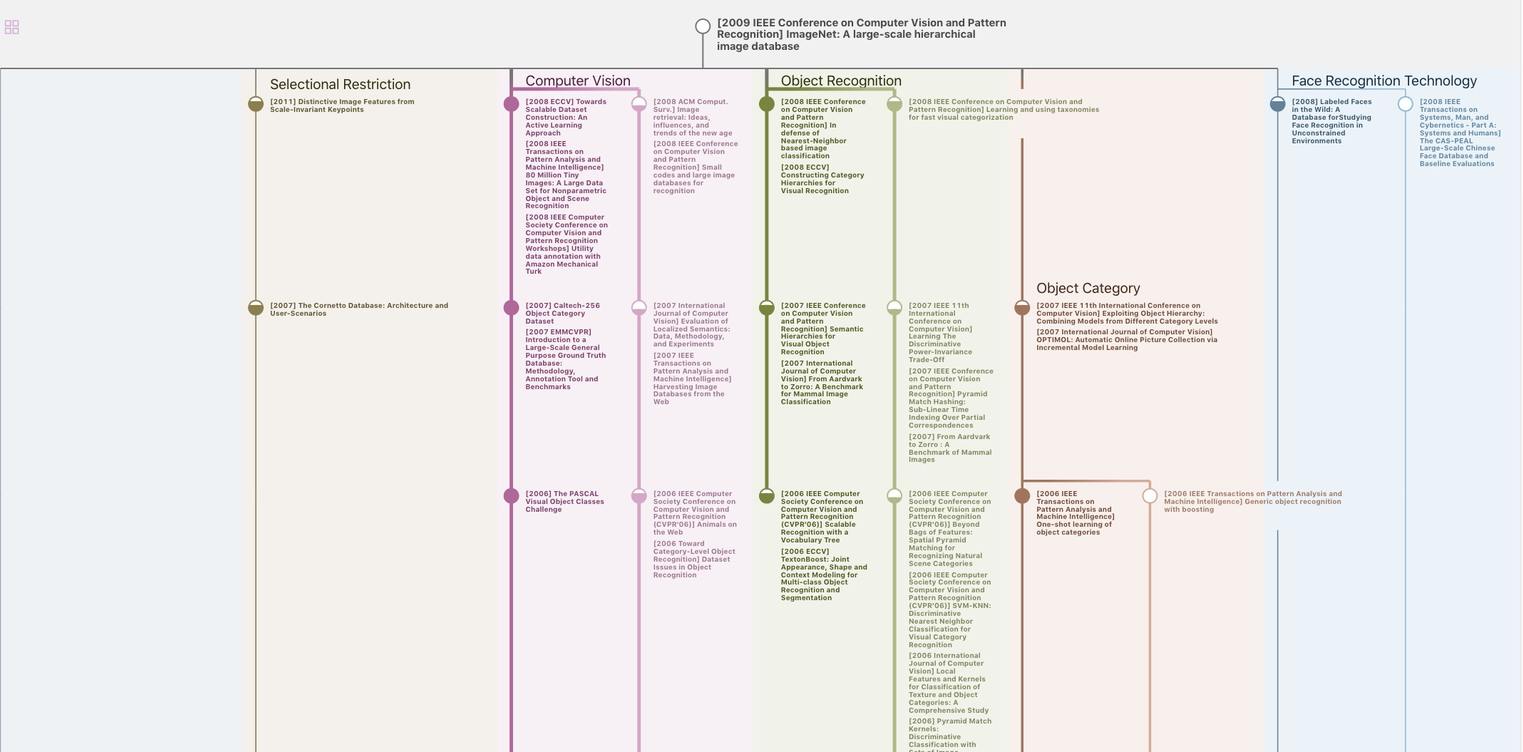
生成溯源树,研究论文发展脉络
Chat Paper
正在生成论文摘要