Deep Learning-Based Segmentation of Pleural Effusion from Ultrasound Using Coordinate Convolutions.
International Conference on Medical Image Computing and Computer-Assisted Intervention (MICCAI)(2022)
摘要
In many low-to-middle income (LMIC) countries, ultrasound is used for assessment of pleural effusion. Typically, the extent of the effusion is manually measured by a sonographer, leading to significant intra-/inter-observer variability. In this work, we investigate the use of deep learning (DL) to automate the process of pleural effusion segmentation from ultrasound images. On two datasets acquired in a LMIC setting, we achieve median Dice Similarity Coefficients (DSCs) of 0.82 and 0.74 respectively using the nnU-net DL model. We also investigate the use of coordinate convolutions in the DL model and find that this results in a statistically significant improvement in the median DSC on the first dataset to 0.85, with no significant change on the second dataset. This work showcases, for the first time, the potential of DL in automating the process of effusion assessment from ultrasound in LMIC settings where there is often a lack of experienced radiologists to perform such tasks.
更多查看译文
关键词
pleural effusion,ultrasound,coordinate convolutions,deep,learning-based
AI 理解论文
溯源树
样例
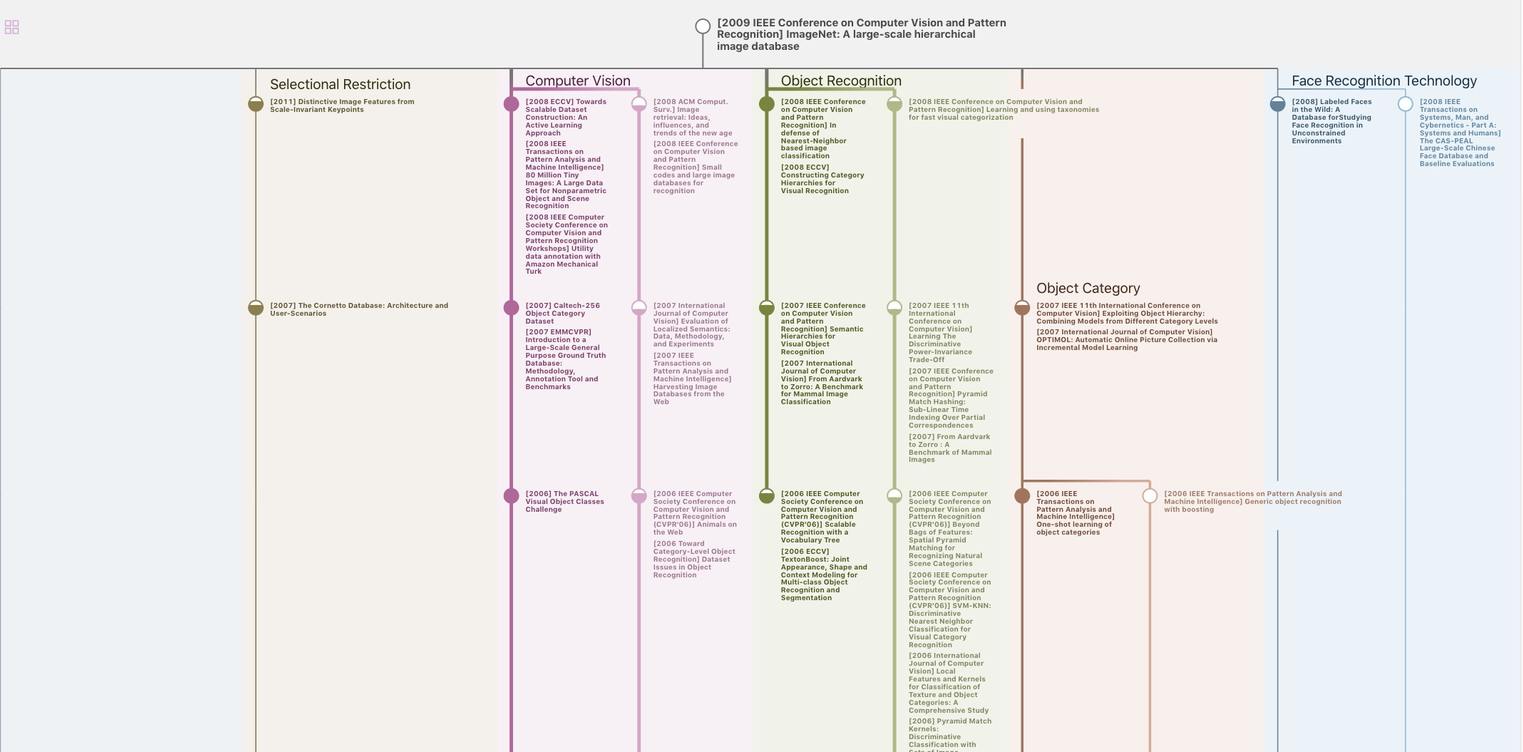
生成溯源树,研究论文发展脉络
Chat Paper
正在生成论文摘要