Learning New Skills after Deployment: Improving open-domain internet-driven dialogue with human feedback
PROCEEDINGS OF THE 61ST ANNUAL MEETING OF THE ASSOCIATION FOR COMPUTATIONAL LINGUISTICS (ACL 2023): LONG PAPERS, VOL 1(2022)
Abstract
Frozen models trained to mimic static datasets can never improve their performance. Models that can employ internet-retrieval for up-to-date information and obtain feedback from humans during deployment provide the promise of both adapting to new information, and improving their performance. In this work we study how to improve internet-driven conversational skills in such a learning framework. We collect deployment data, which we make publicly available, of human interactions, and collect various types of human feedback -- including binary quality measurements, free-form text feedback, and fine-grained reasons for failure. We then study various algorithms for improving from such feedback, including standard supervised learning, rejection sampling, model-guiding and reward-based learning, in order to make recommendations on which type of feedback and algorithms work best. We find the recently introduced Director model (Arora et al., '22) shows significant improvements over other existing approaches.
MoreTranslated text
AI Read Science
Must-Reading Tree
Example
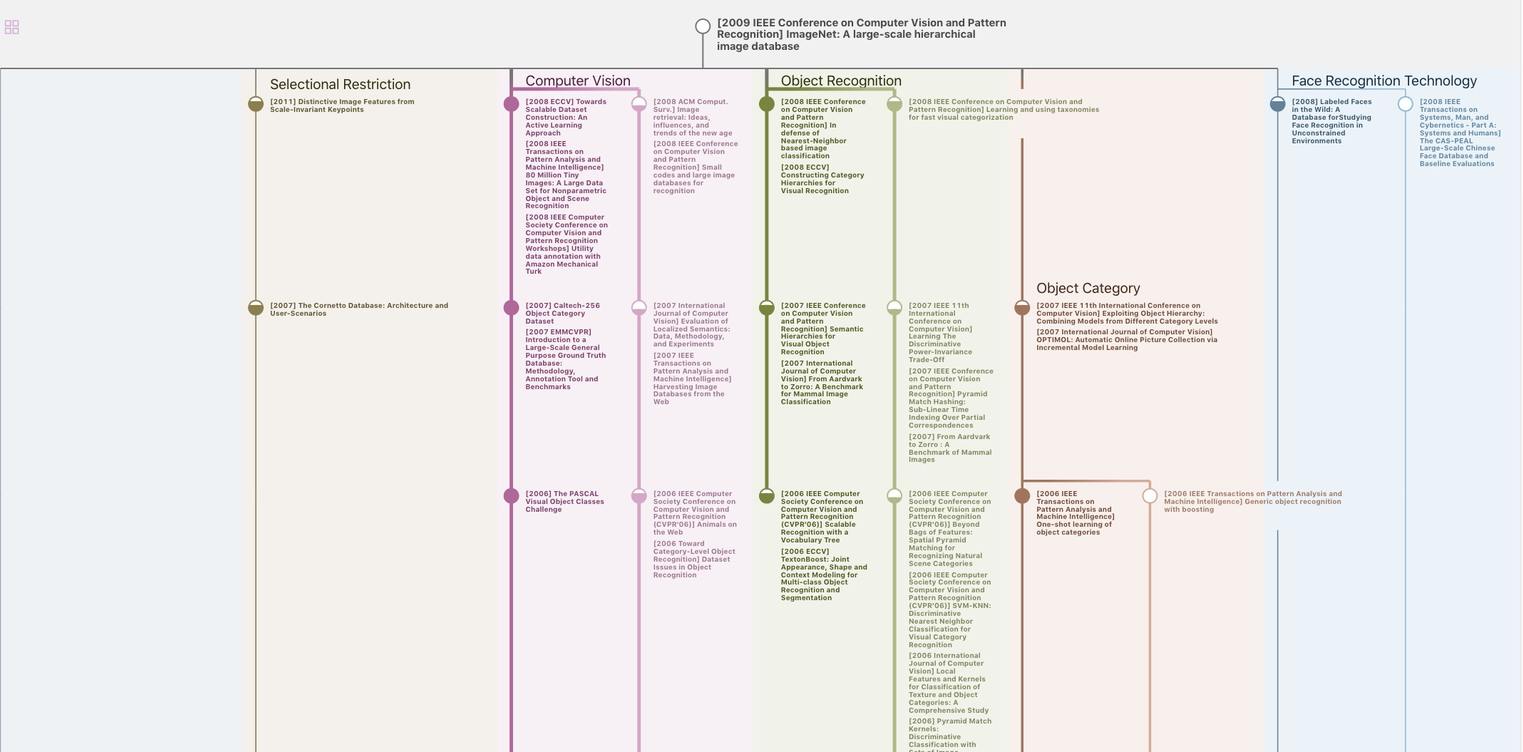
Generate MRT to find the research sequence of this paper
Chat Paper
Summary is being generated by the instructions you defined