ACE: Adaptive Constraint-aware Early Stopping in Hyperparameter Optimization
CoRR(2022)
Abstract
Deploying machine learning models requires high model quality and needs to comply with application constraints. That motivates hyperparameter optimization (HPO) to tune model configurations under deployment constraints. The constraints often require additional computation cost to evaluate, and training ineligible configurations can waste a large amount of tuning cost. In this work, we propose an Adaptive Constraint-aware Early stopping (ACE) method to incorporate constraint evaluation into trial pruning during HPO. To minimize the overall optimization cost, ACE estimates the cost-effective constraint evaluation interval based on a theoretical analysis of the expected evaluation cost. Meanwhile, we propose a stratum early stopping criterion in ACE, which considers both optimization and constraint metrics in pruning and does not require regularization hyperparameters. Our experiments demonstrate superior performance of ACE in hyperparameter tuning of classification tasks under fairness or robustness constraints.
MoreTranslated text
Key words
hyperparameter
AI Read Science
Must-Reading Tree
Example
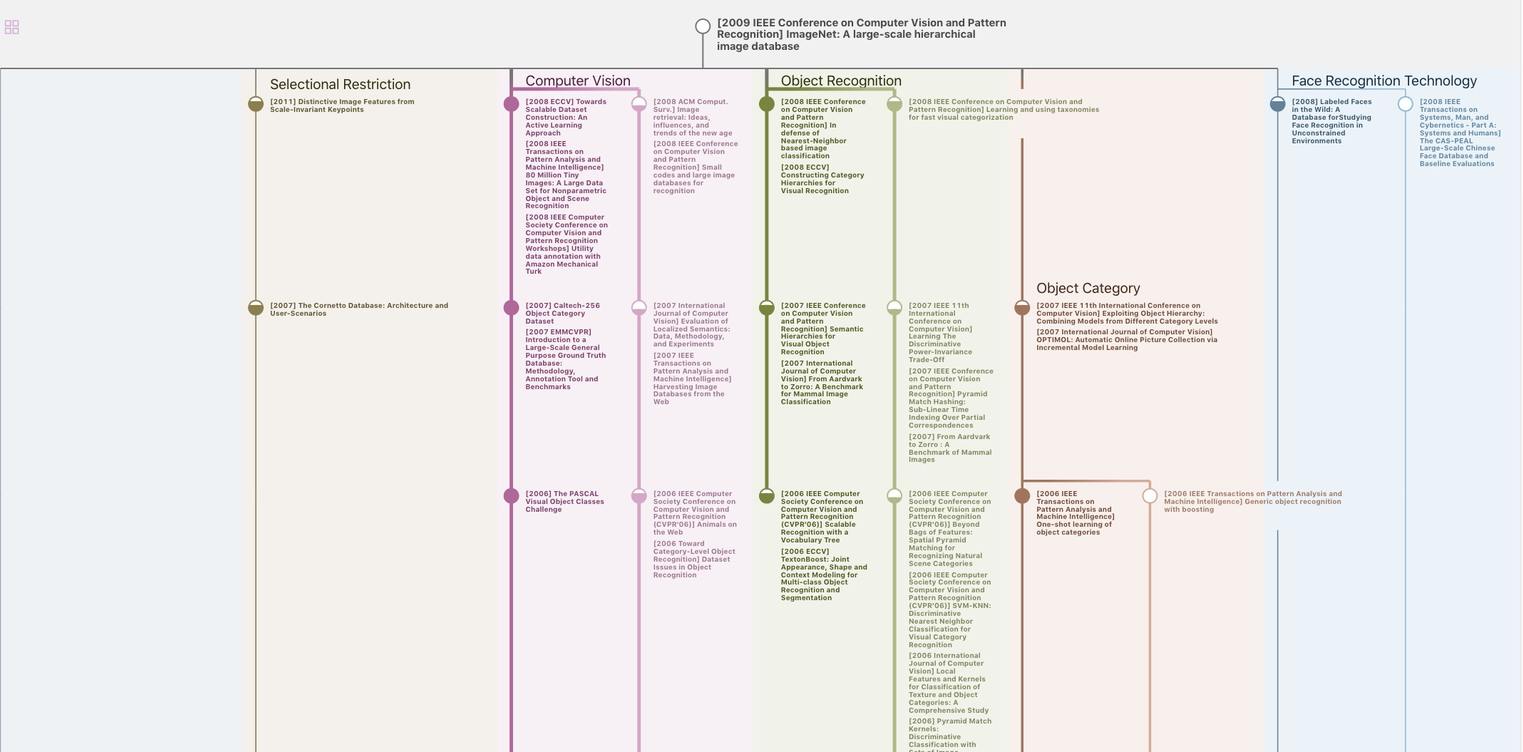
Generate MRT to find the research sequence of this paper
Chat Paper
Summary is being generated by the instructions you defined