Recursive Network Estimation for a Model With Binary-Valued States
IEEE Transactions on Automatic Control(2022)
摘要
This article studies how to estimate the weighted adjacency matrix of a network out of the state sequence of a model with binary-valued states, by using a recursive algorithm. In the considered system, agents display and exchange these binary-valued states generated from intrinsic quantizers. It is shown that stability of the model and identifiability of the system parameters can be guaranteed under continuous random noise. Under standard Gaussian noise, the problem of estimating the real-valued weighted adjacency matrix and the unknown quantization threshold is transformed to an optimization problem via a maximum likelihood approach. It is further verified that the unique solution of the optimization problem is the true parameter vector. A recursive algorithm for the estimation problem is then proposed based on stochastic approximation techniques. Its strong consistency is established and convergence rate analyzed. Numerical simulations are provided to illustrate developed results.
更多查看译文
关键词
Binary-valued states, identifiability, network estimation, quantized identification, stochastic approximation (SA)
AI 理解论文
溯源树
样例
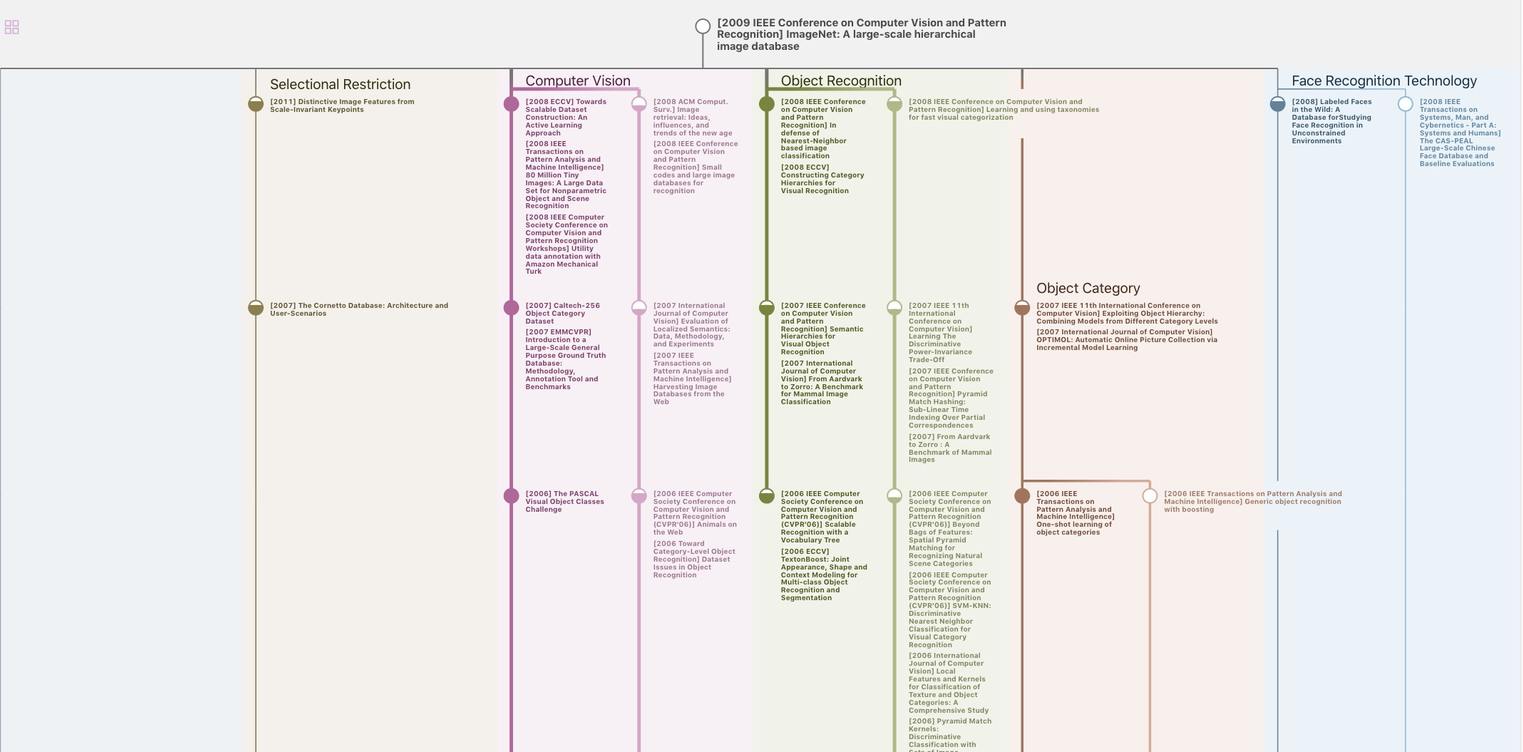
生成溯源树,研究论文发展脉络
Chat Paper
正在生成论文摘要