Retrospective motion correction for preclinical/clinical magnetic resonance imaging based on a conditional generative adversarial network with entropy loss
NMR in biomedicine(2022)
摘要
Multishot scan magnetic resonance imaging (MRI) acquisition is inherently sensitive to motion, and motion artifact reduction is essential for improving the image quality in MRI. This work proposes and validates a new end-to-end motion-correction method for the multishot sequence that incorporates a conditional generative adversarial network with minimum entropy (cGANME) of MR images. The cGANME contains an encoder-decoder generator to obtain motion-corrected images and a PatchGAN discriminator to classify the image as either real (motion-free) or fake (motion-corrected). The entropy of the images is set as one loss item in the cGAN's loss as the entropy increases monotonically with the motion artifacts. An ablation experiment of the different weights of entropy loss was performed to evaluate the function of entropy loss. The preclinical dataset was acquired with a fast spin echo pulse sequence on a 7.0-T scanner. After the simulation, we had 10,080/2880/1440 slices for training, testing, and validating, respectively. The clinical dataset was downloaded from the Human Connection Project website, and 11,300/3500/2000 slices were used for training, testing, and validating after simulation, respectively. Extensive experiments for different motion patterns, motion levels, and protocol parameters demonstrate that cGANME outperforms traditional and some state-of-the-art, deep learning-based methods. In addition, we tested cGANME on in vivo awake rats and mitigated the motion artifacts, indicating that the model has some generalizability.
更多查看译文
关键词
conditional GAN,deep learning,entropy loss,fast spin echo,motion correction
AI 理解论文
溯源树
样例
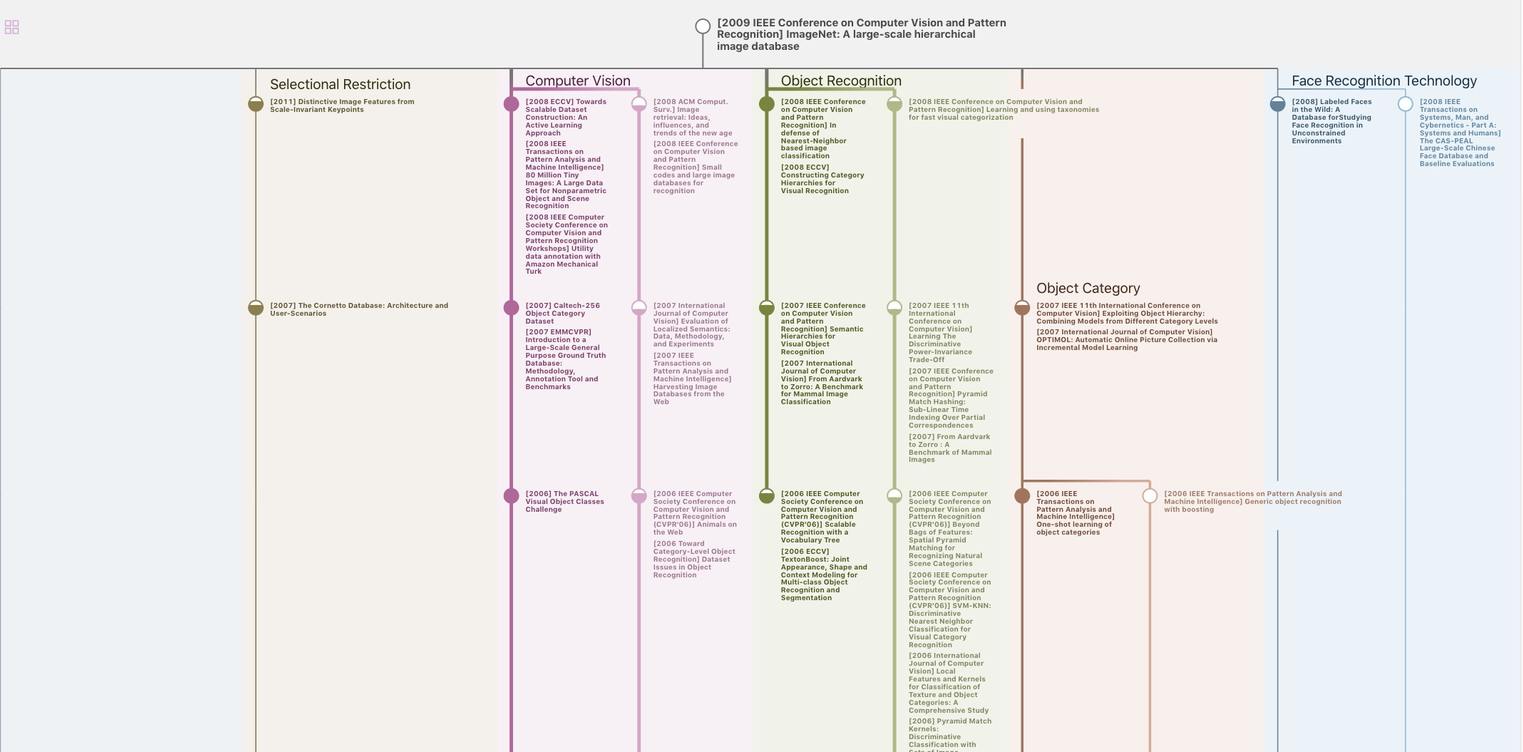
生成溯源树,研究论文发展脉络
Chat Paper
正在生成论文摘要