Multi-agent reinforcement learning for long-term network resource allocation through auction: A V2X application
Computer Communications(2022)
摘要
We formulate offloading of computational tasks from a dynamic group of mobile agents (e.g., cars) as decentralized decision making among autonomous agents. We design an interaction mechanism that incentivizes such agents to align private and system goals by balancing between competition and cooperation. In the static case, the mechanism provably has Nash equilibria with optimal resource allocation. In a dynamic environment, this mechanism’s requirement of complete information is impossible to achieve. For such environments, we propose a novel multi-agent online learning algorithm that learns with partial, delayed and noisy state information, thus greatly reducing information need. Our algorithm is also capable of learning from long-term and sparse reward signals with varying delay. Empirical results from the simulation of a V2X application confirm that through learning, agents with the learning algorithm significantly improve both system and individual performance, reducing up to 30% of offloading failure rate, communication overhead and load variation, increasing computation resource utilization and fairness. Results also confirm the algorithm’s good convergence and generalization property in different environments.
更多查看译文
关键词
Offloading,Distributed systems,Reinforcement learning,Decentralized decision-making
AI 理解论文
溯源树
样例
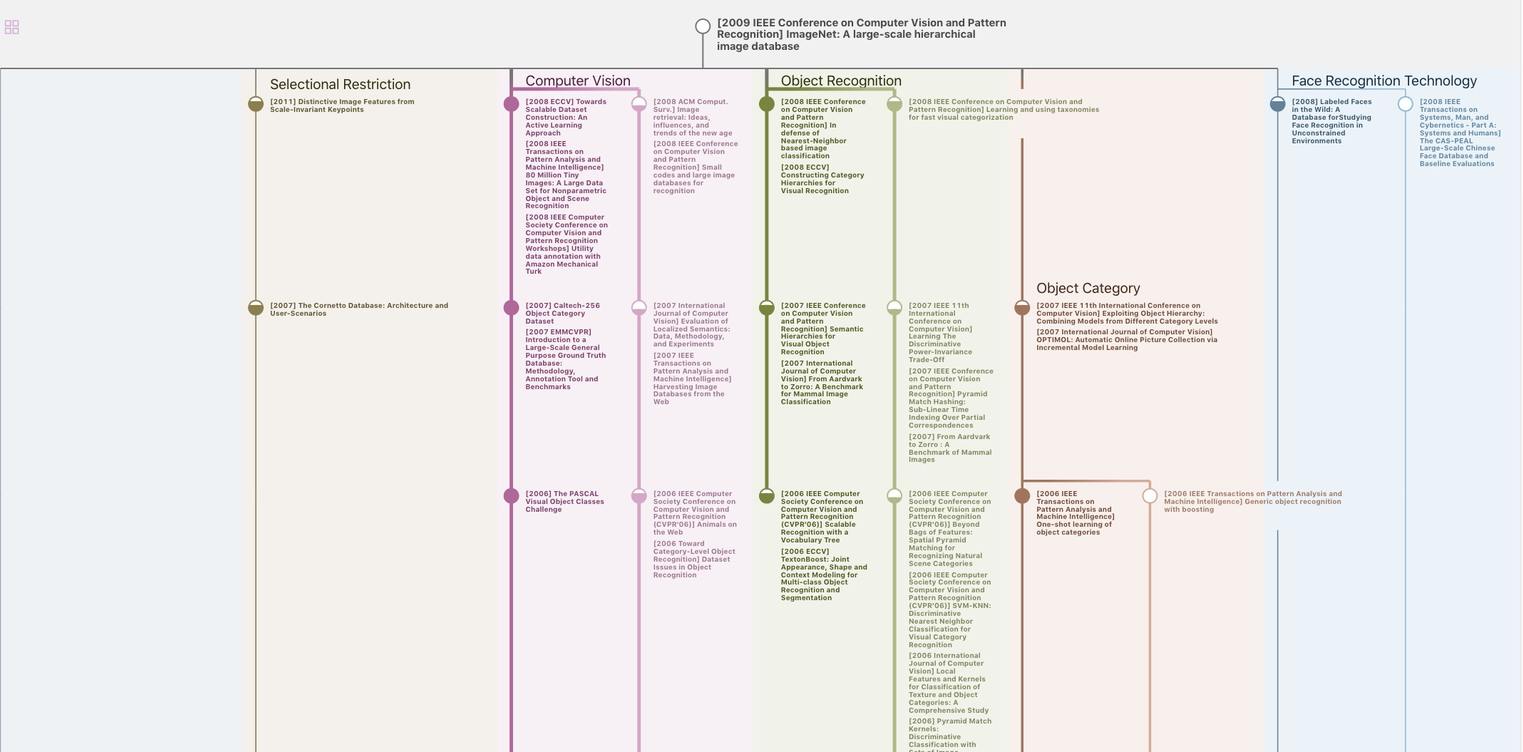
生成溯源树,研究论文发展脉络
Chat Paper
正在生成论文摘要