Rapid fault extraction from seismic images via deep learning
Journal of Electronic Imaging(2022)
摘要
Using deep learning to automatically and quickly extract faults from seismic images is of practical significance. An improved U-Net algorithm is proposed by reducing convolutional layers, designing skip connections, enforcing deep supervision, and improving the loss function and learning rate to build a new model. In the operation, the feature map parameters in the network are further revised, the number of training iterations is increased, a callback function is added, and the parameter adjustment training consumes less time and space and has higher accuracy. Experiments on real public datasets show that the improved network can limit the time required to extract a 128 x 128 x 128 three-dimensional image within 200 ms, which not only requires less time and computing power than existing methods but also has an extraction accuracy as high as 97.6%. (c) 2022 SPIE and IS&T
更多查看译文
关键词
fault extraction,seismic images,three-dimensional U-Net,deep supervision,deep learning
AI 理解论文
溯源树
样例
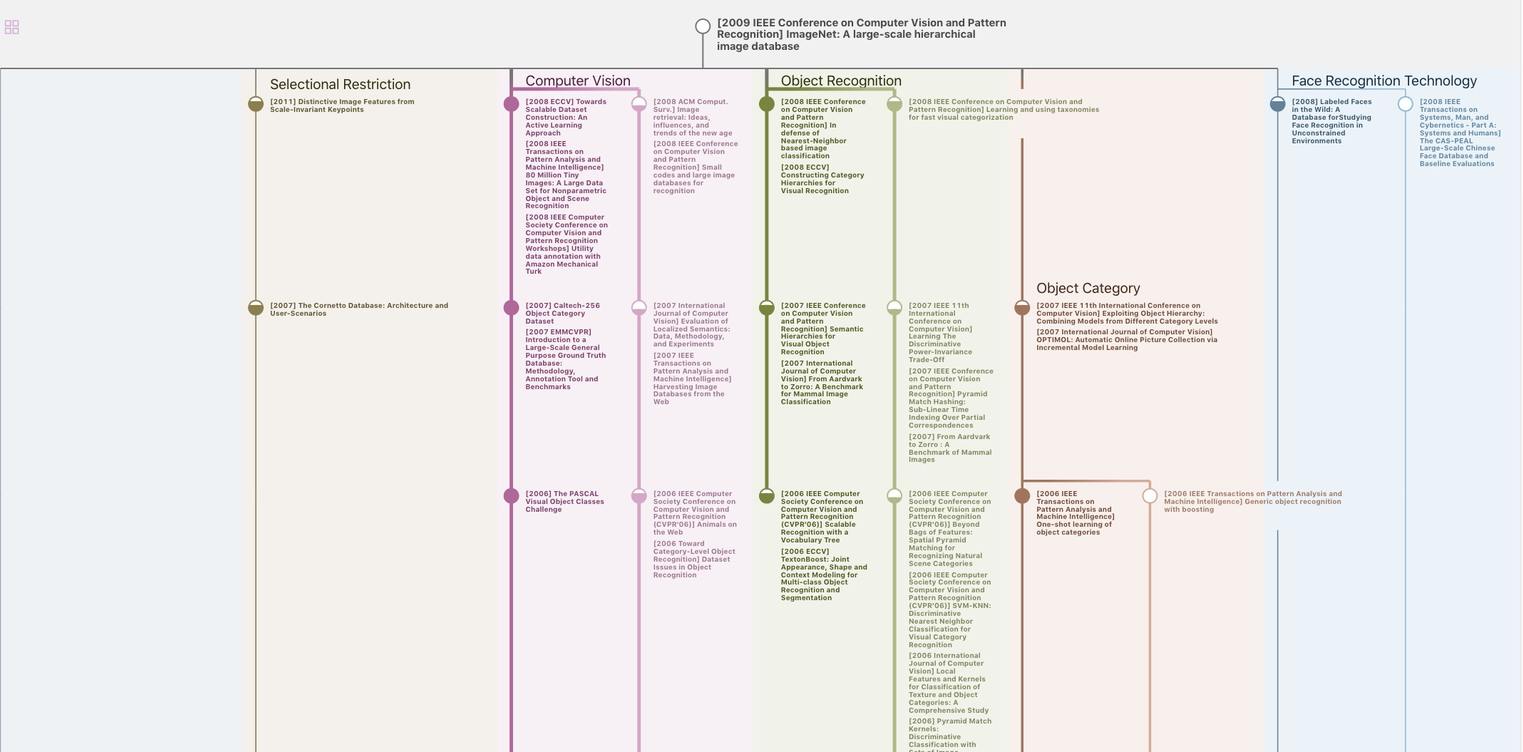
生成溯源树,研究论文发展脉络
Chat Paper
正在生成论文摘要