Linear Combinatorial Semi-Bandit with Causally Related Rewards.
International Joint Conference on Artificial Intelligence(2022)
Abstract
In a sequential decision-making problem, having a structural dependency amongst the reward distributions associated with the arms makes it challenging to identify a subset of alternatives that guarantees the optimal collective outcome. Thus, besides individual actions' reward, learning the causal relations is essential to improve the decision-making strategy. To solve the two-fold learning problem described above, we develop the 'combinatorial semi-bandit framework with causally related rewards', where we model the causal relations by a directed graph in a stationary structural equation model. The nodal observation in the graph signal comprises the corresponding base arm's instantaneous reward and an additional term resulting from the causal influences of other base arms' rewards. The objective is to maximize the long-term average payoff, which is a linear function of the base arms' rewards and depends strongly on the network topology. To achieve this objective, we propose a policy that determines the causal relations by learning the network's topology and simultaneously exploits this knowledge to optimize the decision-making process. We establish a sublinear regret bound for the proposed algorithm. Numerical experiments using synthetic and real-world datasets demonstrate the superior performance of our proposed method compared to several benchmarks.
MoreTranslated text
Key words
Uncertainty in AI: Sequential Decision Making,Machine Learning: Online Learning,Machine Learning: Sequence and Graph Learning
AI Read Science
Must-Reading Tree
Example
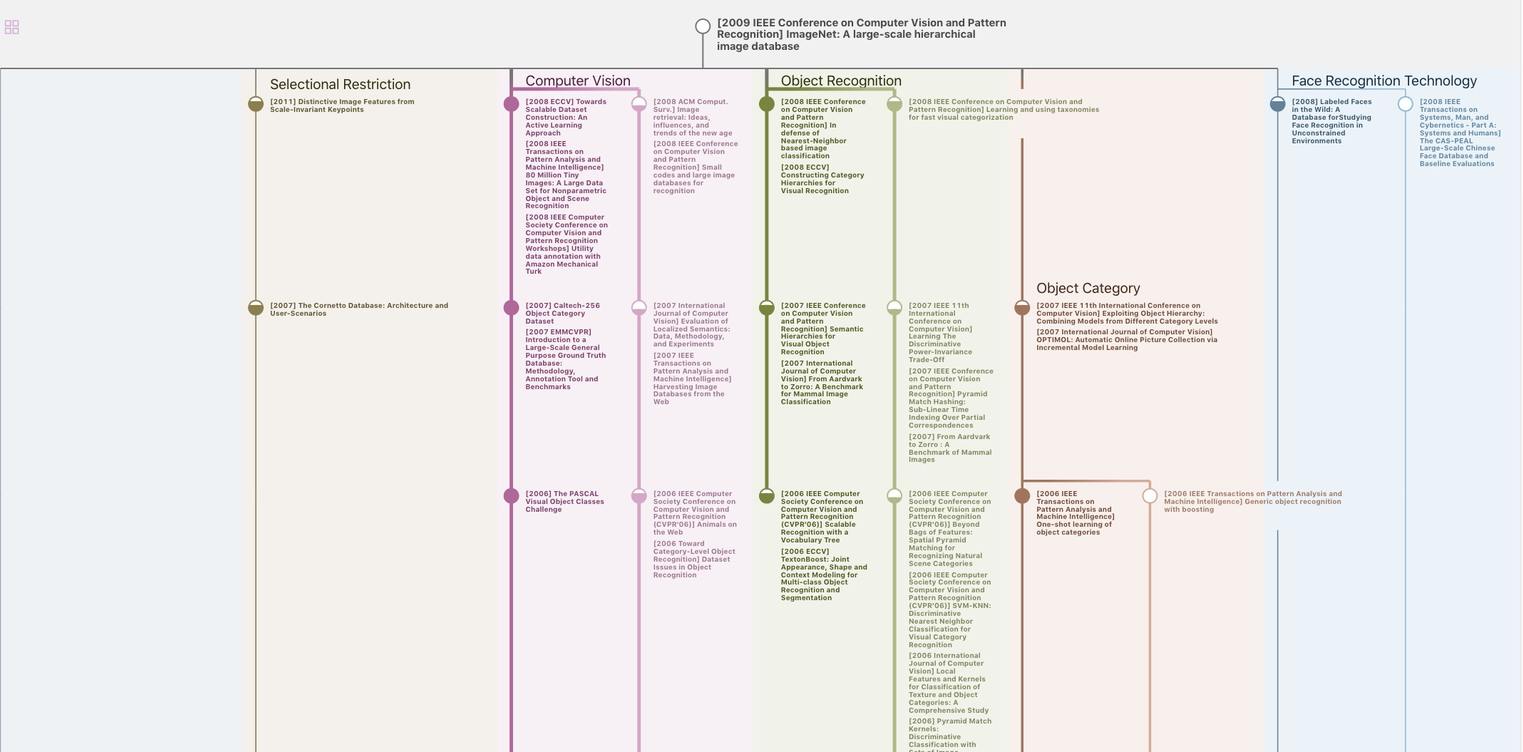
Generate MRT to find the research sequence of this paper
Chat Paper
Summary is being generated by the instructions you defined