A Gaussian RBM with binary auxiliary units
International Journal of Machine Learning and Cybernetics(2022)
摘要
Restricted Boltzmann Machines (RBM) have been widely applied in image processing. For RBM-based models on image recognition and image generation tasks, extracting expressive real-valued features and alleviating the overfitting problem are extremely important. In this paper, we propose a Gaussian Restricted Boltzmann Machine with binary Auxiliary units (GARBM), which designs binary auxiliary units in its visible layer and constructs parameterized real-valued features in its hidden layer. Specifically, based on the designed energy function in GARBM, activated auxiliary units are directly used to control probabilities of visible units and hidden units to extract real-valued features. Moreover, auxiliary units and their resulting feature selection mechanism not only alleviate the “gradient-variance” problem, but also provide certain randomness to other units to alleviate overfitting without introducing more hyperparameters. To build more effective deep models, we propose GARBM-based deep neural networks, and the effectiveness of proposed neural networks is verified in experiments.
更多查看译文
关键词
Restricted Boltzmann machine, Truncated Gaussian distribution, Deep belief net, Variational AutoEncoder, Auxiliary unit
AI 理解论文
溯源树
样例
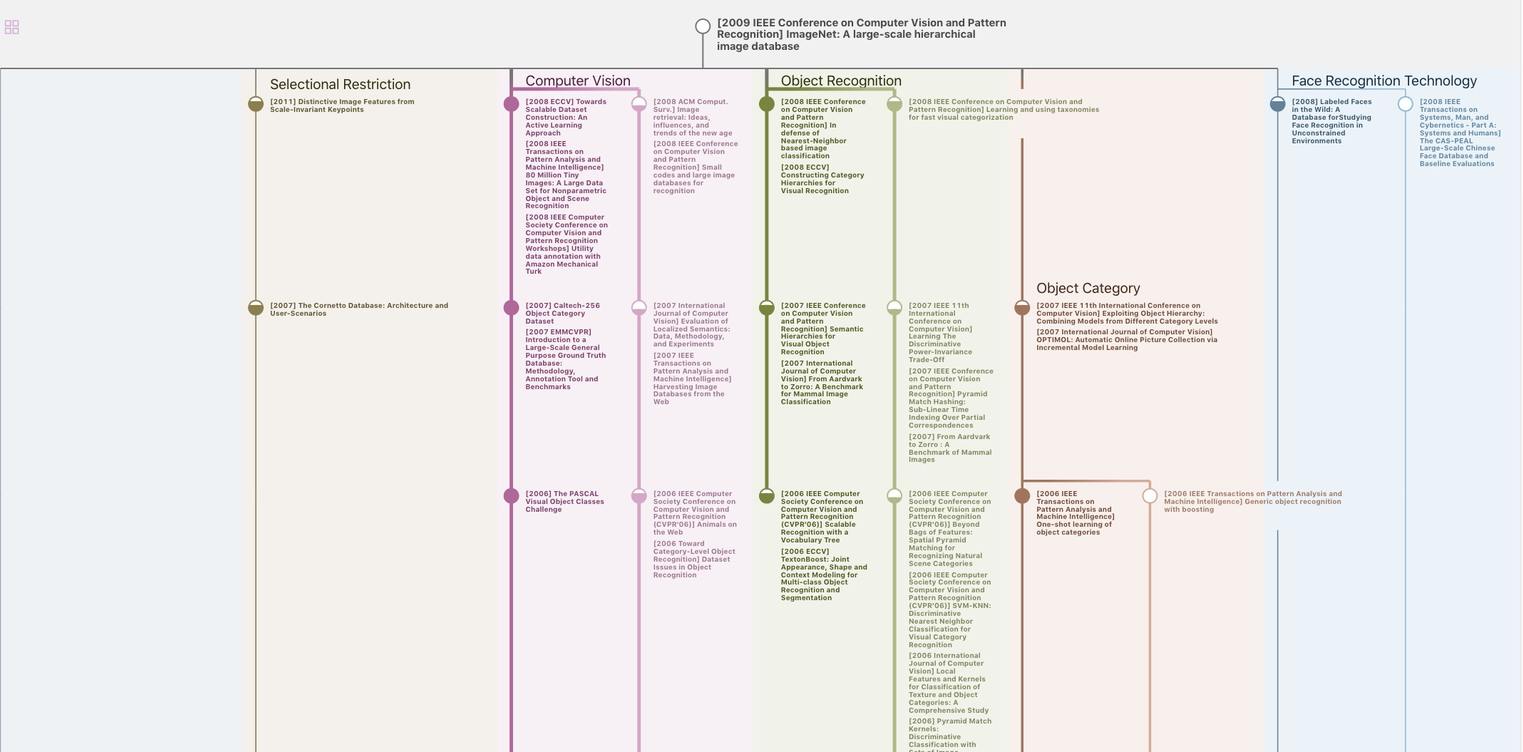
生成溯源树,研究论文发展脉络
Chat Paper
正在生成论文摘要