Non-invasive imaging through scattering media with unaligned data using dual-cycle GANs
Optics Communications(2022)
Abstract
Benefiting from deep learning (DL), great progress has been made in the research of imaging through scattering media. Most DL methods use a training set of aligned image pairs to learn the mapping between the speckle image and the object image. However, the alignment process of the dataset is invasive, which will be greatly limited in actual scenes. Here, we propose a method for non-invasive imaging through scattering media with unaligned data using dual-cycle Generative Adversarial Networks (GANs). In this method, we preprocess the unaligned speckle images and object images to obtain their respective autocorrelation images. Then, the autocorrelation images of the speckle and the object are fed into the dual-cycle GANs for label-free training to realize the image translation between them. Finally, a pre-trained convolutional neural network (CNN) is used to reconstruct the translated autocorrelation image of the object into the clear object. Experimental results show that our method can accurately reconstruct the unknown objects with different complexity through scattering media with different statistical properties. Our method has great potential in solving the problem of scattering imaging in actual scenes.
MoreTranslated text
Key words
Image reconstruction,Non-invasive,Scattering media,Deep learning
AI Read Science
Must-Reading Tree
Example
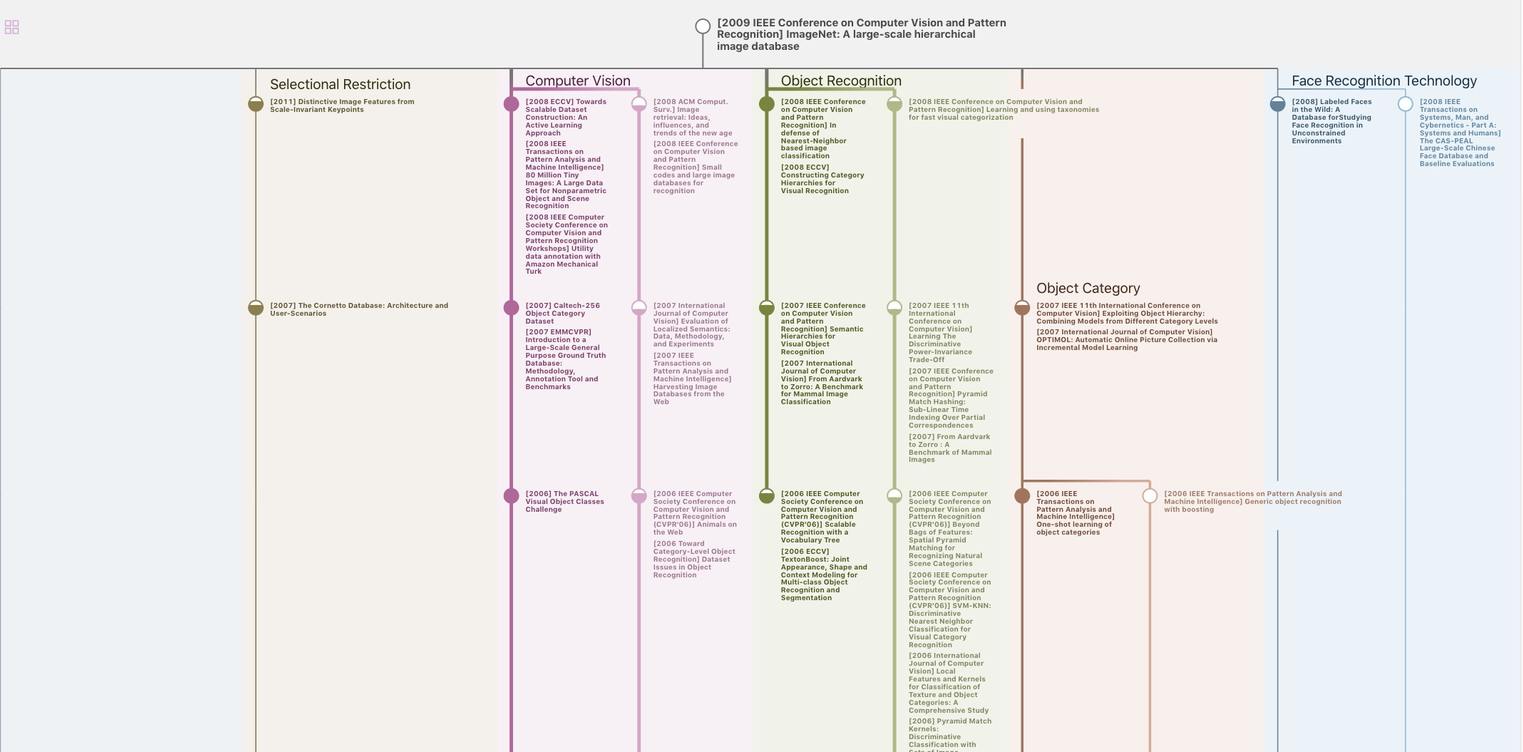
Generate MRT to find the research sequence of this paper
Chat Paper
Summary is being generated by the instructions you defined