Analysis and Detection against Network Attacks in the Overlapping Phenomenon of Behavior Attribute
Computers & Security(2022)
摘要
The proliferation of network attacks poses a significant threat. Researchers propose datasets for network attacks to support research in related fields. Then, many attack detection methods based on these datasets are proposed. These detection methods, whether two-classification or multi-classification, belong to single-label learning, i.e., only one label is given to each sample. However, we discover that there is a noteworthy phenomenon of behavior attribute overlap between attacks, The presentation of this phenomenon in a dataset is that there are multiple samples with the same features but different labels. In this paper, we verify the phenomenon in well-known datasets(UNSW-NB15, CCCS-CIC-AndMal-2020) and re-label these data. In addition, detecting network attacks in a multi-label manner can obtain more information, providing support for tracing the attack source and building IDS. Therefore, we propose a multi-label detection model based on deep learning, MLD-Model, in which Wasserstein-Generative-Adversarial-Network-with-Gradient-Penalty (WGAN-GP) with improved loss performs data enhancement to alleviate the class imbalance problem, and Auto-Encoder (AE) performs classifier parameter pre-training. Experimental results demonstrate that MLD-Model can achieve excellent classification performance. It can achieve F1=80.06% in UNSW-NB15 and F1=83.63% in CCCS-CIC-AndMal-2020. Especially, MLD-Model is 5.99%∼7.97% higher in F1 compared with the related single-label methods.
更多查看译文
关键词
Overlapping attribute,Multi-label,Network attack detection,Data enhancement,Pre-training
AI 理解论文
溯源树
样例
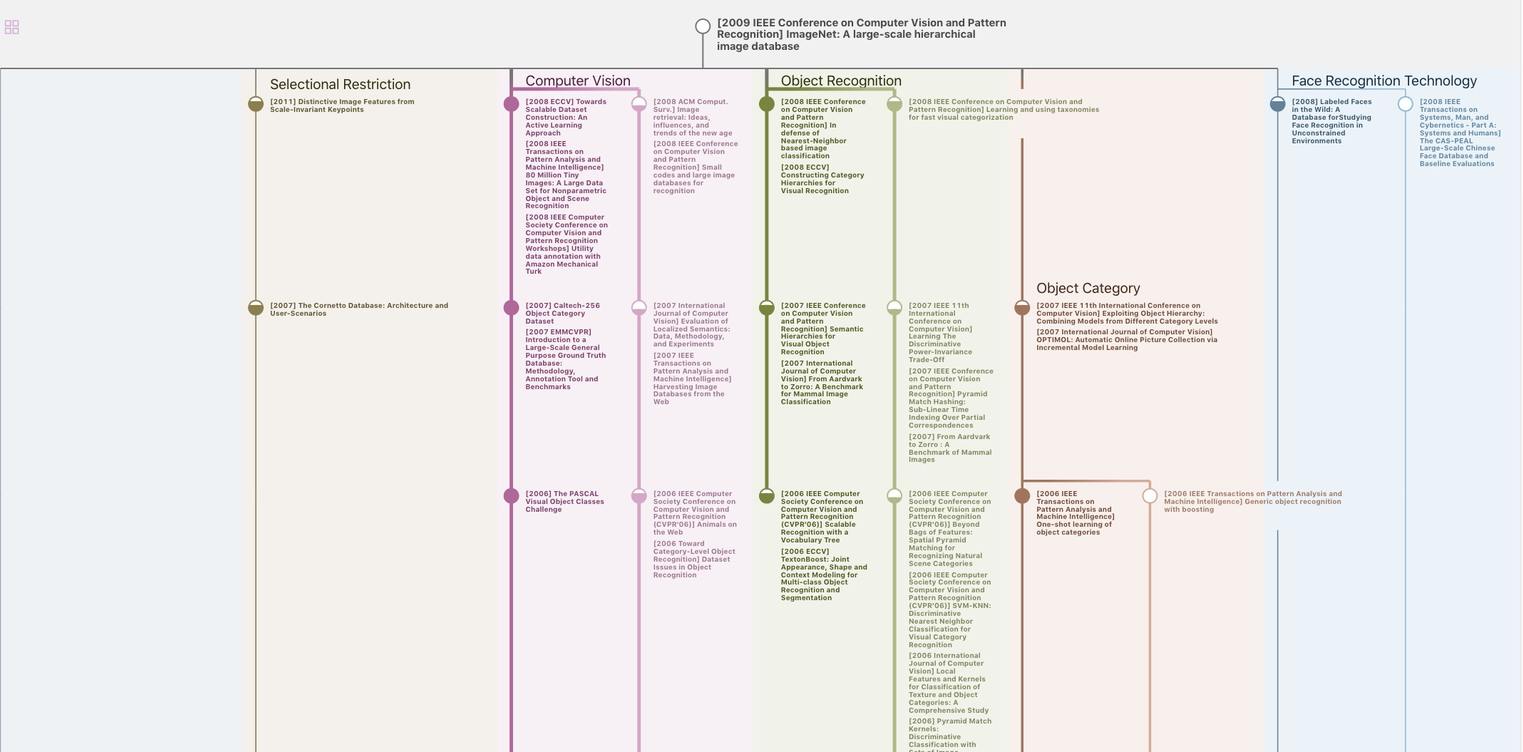
生成溯源树,研究论文发展脉络
Chat Paper
正在生成论文摘要