What Do Deep Neural Networks Find in Disordered Structures of Glasses?
arxiv(2022)
摘要
Glass transitions are widely observed in various types of soft matter systems. However, the physical mechanism of these transitions remains {elusive}, despite years of ambitious research. In particular, an important unanswered question is whether the glass transition is accompanied by a divergence of the correlation lengths of the characteristic static structures. In this study, we develop a deep-neural-network-based method that is used to extract the characteristic local meso-structures solely from instantaneous {particle} configurations without any {information} about the dynamics. We first train a neural network to classify configurations of liquids and glasses correctly. Then, we obtain the characteristic structures by quantifying the grounds for the decisions made by the network using Gradient-weighted Class Activation Mapping (Grad-CAM). We considered two qualitatively different glass-forming binary systems, and through comparisons with several established structural indicators, we demonstrate that our system can be used to identify characteristic structures that depend on the details of the systems. Moreover, the extracted structures are remarkably correlated with the nonequilibrium aging dynamics in thermal fluctuations.
更多查看译文
关键词
deep neural networks,machine learning,molecular dynamics simulations,glass transition,dynamical heterogeneity of liquid state
AI 理解论文
溯源树
样例
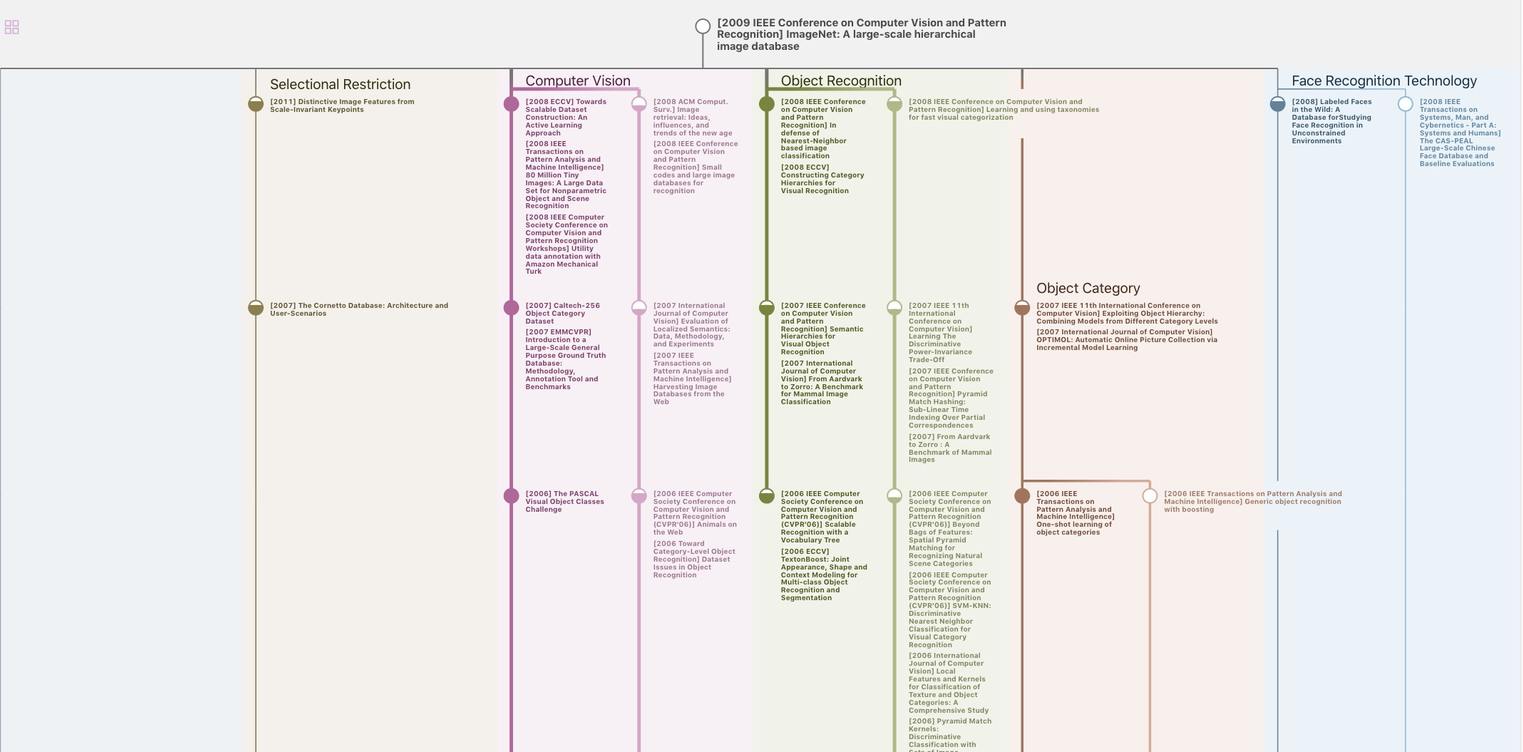
生成溯源树,研究论文发展脉络
Chat Paper
正在生成论文摘要