Convolutional neural network with a hybrid loss function for fully automated segmentation of lymphoma lesions in FDG PET images
MEDICAL IMAGING 2022: IMAGE PROCESSING(2022)
摘要
Segmentation of lymphoma lesions is challenging due to their varied sizes and locations in whole-body PET scans. In this work, we present a fully-automated segmentation technique using a multi-center dataset of diffuse large B-cell lymphoma (DLBCL) with heterogeneous characteristics. We utilized a dataset of [18F]FDG-PET scans (n=194) from two different imaging centers including cases with primary mediastinal large B-cell lymphoma (PMBCL) (n=104). Automated brain and bladder removal approaches were utilized as preprocessing steps, to tackle false positives caused by normal hypermetabolic uptake in these organs. Our segmentation model is a convolutional neural network (CNN), based on a 3D U-Net architecture that includes squeeze and excitation (SE) modules. Hybrid distribution, region, and boundary-based losses (Unified Focal and Mumford-Shah (MS)) were utilized that showed the best performance compared to other combinations (p<0.05). Cross-validation between different centers, DLBCL and PMBCL cases, and three random splits were applied on train/validation data. The ensemble of these six models achieved a Dice similarity coefficient (DSC) of 0.77 +/- 0.08 and Hausdorff distance (HD) of 16.5 +/- 12.5. Our 3D U-net model with SE modules for segmentation with hybrid loss performed significantly better (p<0.05) as compared to the 3D U-Net (without SE modules) using the same loss function (Unified Focal and MS loss) (DSC= 0.64 +/- 0.21 and HD= 26.3 +/- 18.7). Our model can facilitate a fully automated quantification pipeline in a multi-center context that opens the possibility for routine reporting of total metabolic tumor volume (TMTV) and other metrics shown useful for the management of lymphoma.
更多查看译文
关键词
Lymphoma,Convolutional neural network,PET,Segmentation,U-Net,Focal loss,hybrid loss
AI 理解论文
溯源树
样例
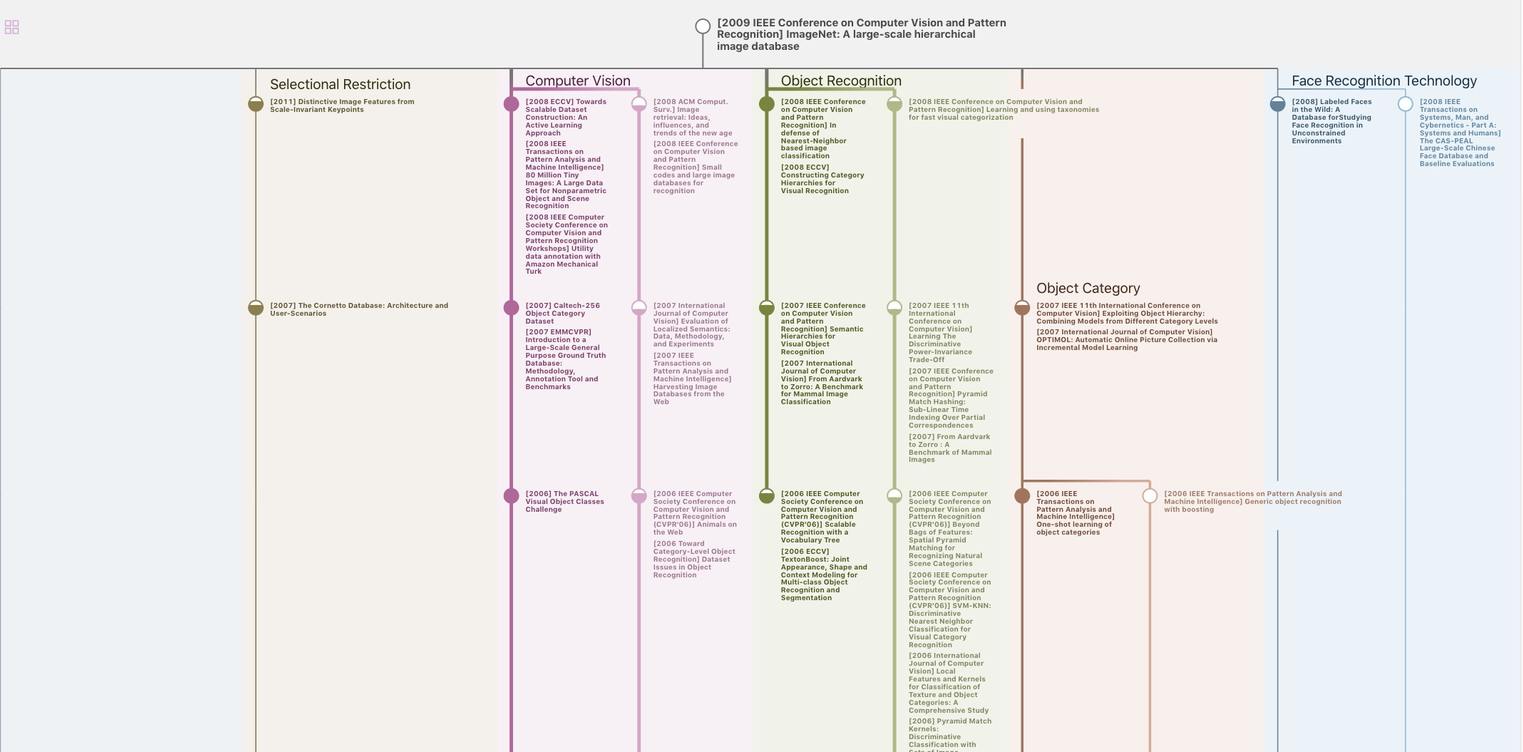
生成溯源树,研究论文发展脉络
Chat Paper
正在生成论文摘要