Development of Deep Learning Architectures for Forecasting Distillation Columns Dynamic Behavior of Biobutanol Purification
Computer Aided Chemical Engineering 32nd European Symposium on Computer Aided Process Engineering(2022)
Abstract
In recent years, Artificial Neural Networks (ANNs) have received special attention to a widespread application in the field of engineering, biology, energy, and finance. Within the ANN design, several factors play a major role in the correct prediction of the process, such as the number of internal layers, the number of neurons, the number of used features, the training algorithm, the activation function, the number of epochs, among many others. Considering datasets of an intensified distillation column generated by Aspen Plus Dynamics at different operation conditions, here we bring clarity in the field with different architectures of ANNs to abstract the dynamics of both an intensified and conventional distillation process that separates an effluent coming from fermentation producing acetone, butanol, and ethanol (ABE) for spark-ignition purposes. Our results highlight that a one-layer neural network can represent the dynamics of an intensified column to forecast the concentration of acetone, butanol, and ethanol. Remarkably, the linear activation function overperforms the tangent hyperbolic as activation functions. Ultimately, we found that the reflux ratio and reboiler duty are key features to reconstruct the full dynamics of the intensified column.
MoreTranslated text
Key words
distillation columns dynamic behavior,deep learning,purification,deep learning architectures,forecasting
AI Read Science
Must-Reading Tree
Example
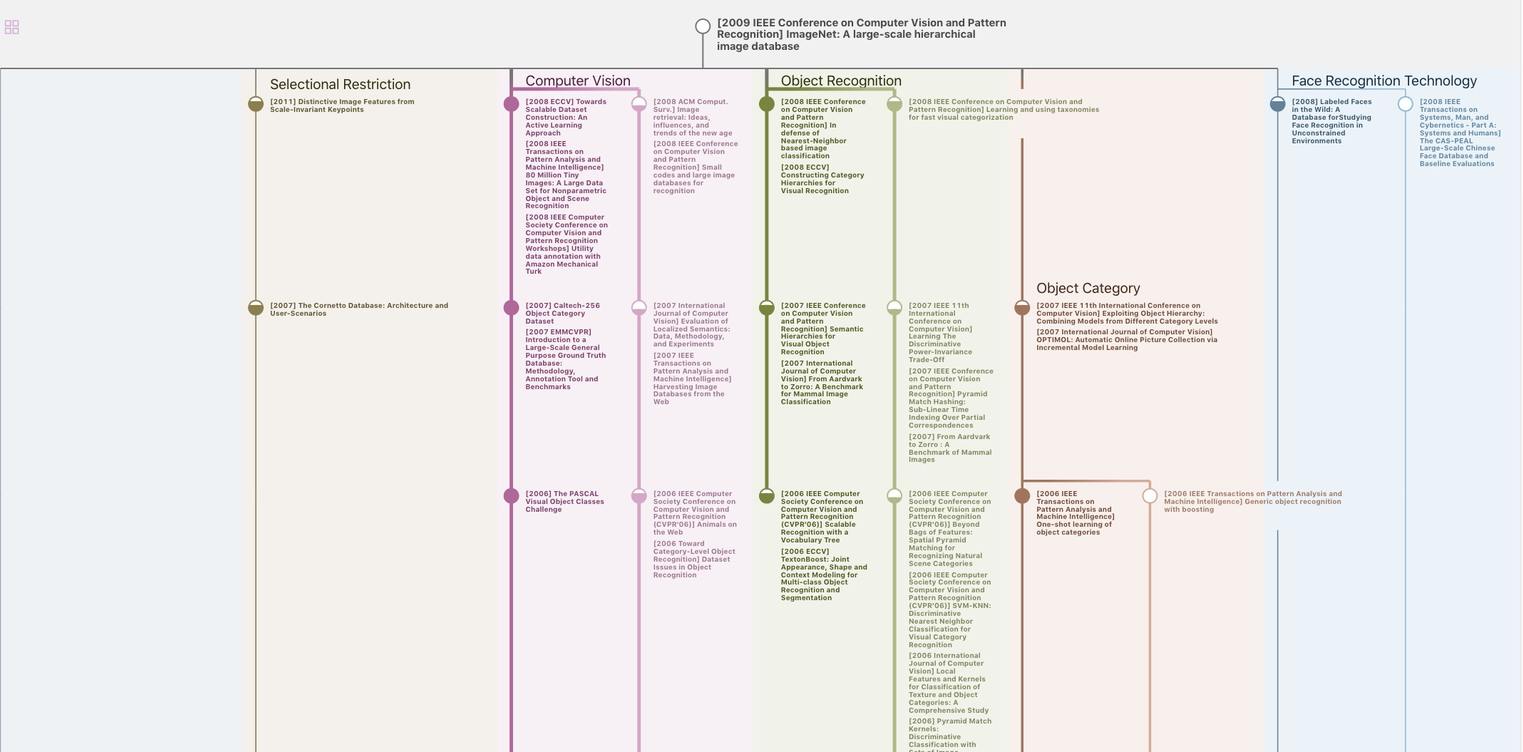
Generate MRT to find the research sequence of this paper
Chat Paper
Summary is being generated by the instructions you defined