Fast, Hierarchical, and Adaptive Algorithm for Metropolis Monte Carlo Simulations of Long-Range Interacting Systems
PHYSICAL REVIEW X(2023)
摘要
We present a fast, hierarchical, and adaptive algorithm for Metropolis Monte Carlo simulations of systems with long-range interactions that reproduces the dynamics of a standard implementation exactly, i.e., the generated configurations and consequently all measured observables are identical, allowing in particular for nonequilibrium studies. The method is demonstrated for the power-law interacting long-range Ising and XY spin models with nonconserved order parameter and a Lennard-Jones particle system, all in two dimensions. The measured run times support an average complexity O(N log N), where N is the number of spins or particles. Importantly, prefactors of this scaling behavior are small, which in practice manifests in speedup factors larger than 104. The method is general and will allow the treatment of large systems that were out of reach before, likely enabling a more detailed understanding of physical phenomena rooted in long-range interactions.
更多查看译文
关键词
metropolis monte carlo simulations,adaptive algorithm,long-range
AI 理解论文
溯源树
样例
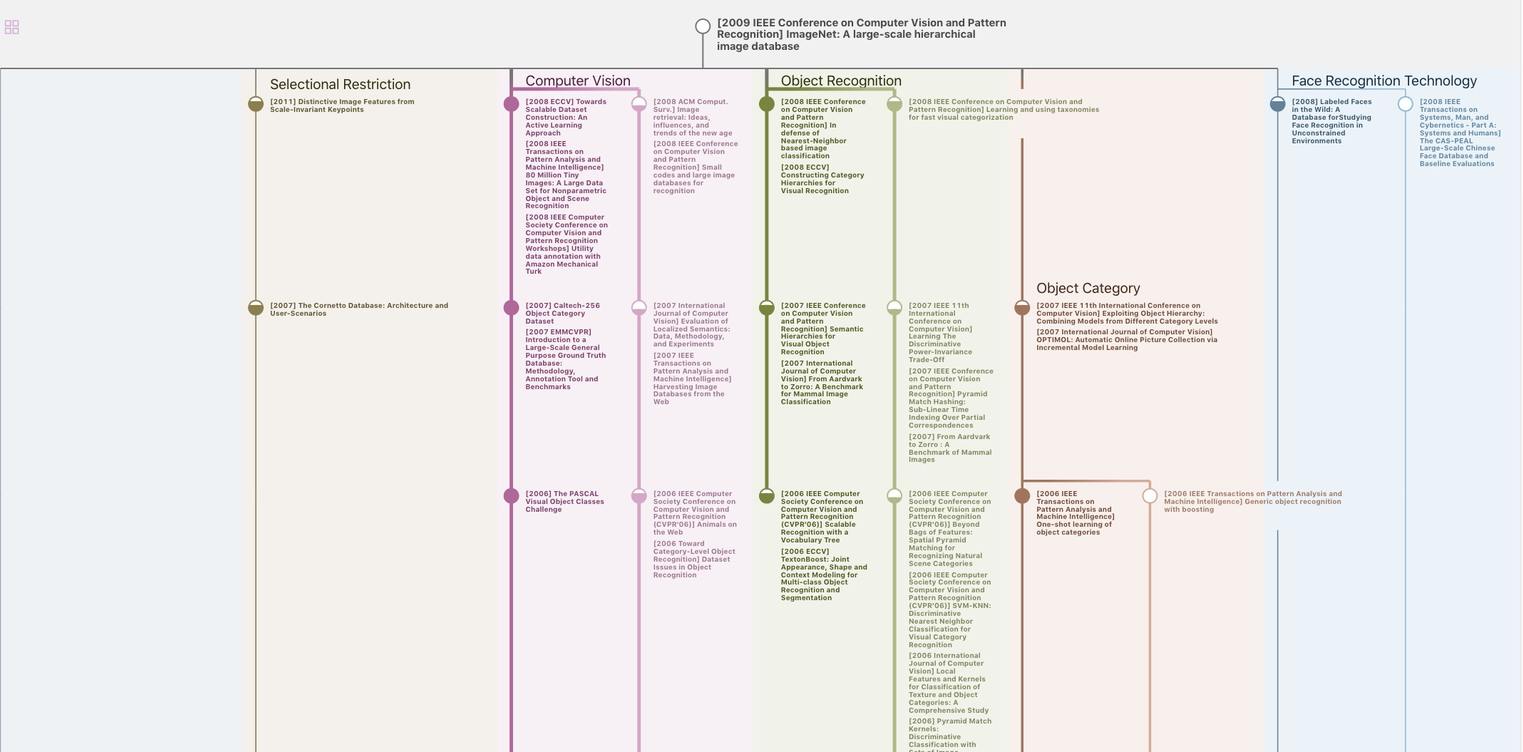
生成溯源树,研究论文发展脉络
Chat Paper
正在生成论文摘要