Leveraging greater relations for improving multi-choice reading comprehension
NEURAL COMPUTING & APPLICATIONS(2022)
摘要
Remarkable success has been achieved in the last few years on machine reading comprehension tasks. In previous works, long-range dependencies were captured by explicitly attending to all the tokens and modeling the relations between the question and each sentence. However, a great deal of important information regarding token-level and sentence-level relations in the passage, which are useful to infer the answer, were ignored in these works. We observed that the contextual information between the token-level and sentence-level in the same passage plays a vital role in reading comprehension tasks. To address this problem, we proposed a multi-stage maximization attention (MMA) network, which is used to capture the important relations in the passage from different levels of granularity at its hierarchical nature. By utilizing MMA as a module, we integrated two sentence-level question-aware matching mechanisms to infer the answer: (1) Co-matching is used to match the passage with the question and the candidate answer. (2) Sentence-level hierarchical attention is used to identify the importance of sentences conditioned on the question and the option. In addition, inspired by how humans solve multi-choice reading comprehension questions, the passage sentence selection strategy is fused into our model to select the most salient sentences to guide the model to infer the answer. The proposed model is evaluated on three multi-choice reading comprehension datasets RACE, Dream and MultiRC. Significance tests demonstrated the improvement of existing MRC models. A series of analyses were also conducted to interpret the effectiveness of the proposed model.
更多查看译文
关键词
Multi-choice reading comprehension,Multi-stage maximization attention,Passage sentence selection,Contextual modeling
AI 理解论文
溯源树
样例
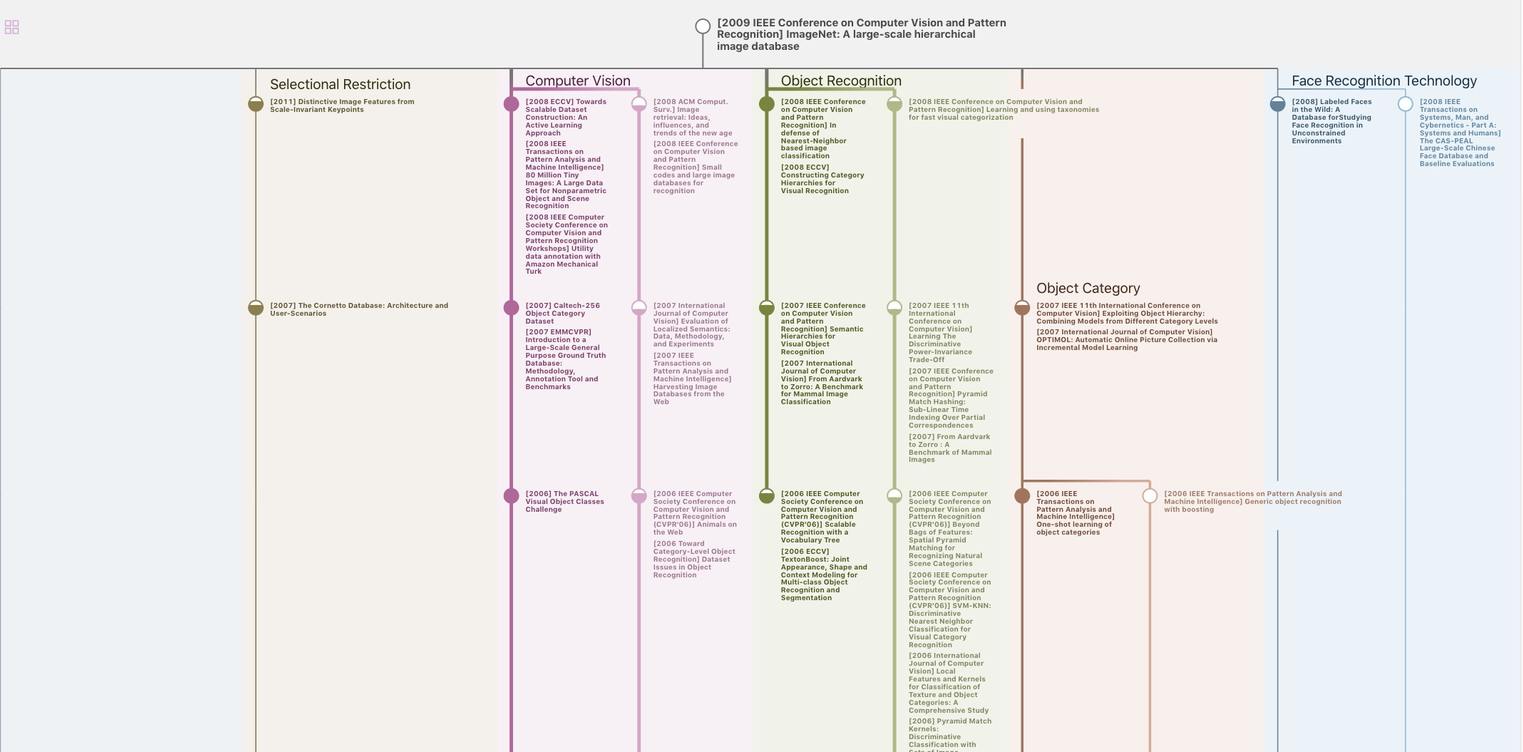
生成溯源树,研究论文发展脉络
Chat Paper
正在生成论文摘要