A novel deep convolutional neural network algorithm for surface defect detection
JOURNAL OF COMPUTATIONAL DESIGN AND ENGINEERING(2022)
摘要
The surface defect detection (SDD) problem is one of the crucial techniques during production process, so it has become a key research area to control the quality of industrial products, which has been increasingly of greater interest to the researchers especially with the rapid development of artificial neural networks technology in recent years. Therefore, this paper proposes a novel deep convolutional neural network algorithm aiming at SDD. Firstly, a dense cross-stage partial Darknet backbone network is designed for feature extraction by optimizing cross-stage partial Darknet through the idea of dense connections, which can, not only enhance feature reuse but also greatly alleviate the overfitting issue. Secondly, a new cross-stage hierarchy module is presented combining the cross-stage feature fusion strategy and depthwise separable convolution technique for each node of the path aggregated feature pyramid network (PAN). Finally, an efficient channel attention (ECA) mechanism is introduced in PAN to construct a novel ECA PAN. The experimental results on three surface defect datasets show that the mean average precision of this network is 2.63, 5.48, and 1.16% which is higher than that of the baseline network, respectively. The proposed network outperforms not only the classical models but state-of-the-art models, which indicates the proposed algorithm can achieve higher accuracy and speed with fewer calculation parameters. And what is more, the proposed algorithm also has outstanding generalization ability.
更多查看译文
关键词
surface defect detection,deep convolutional neural network,densely connected convolutional network,attention mechanism,cross-stage feature fusion
AI 理解论文
溯源树
样例
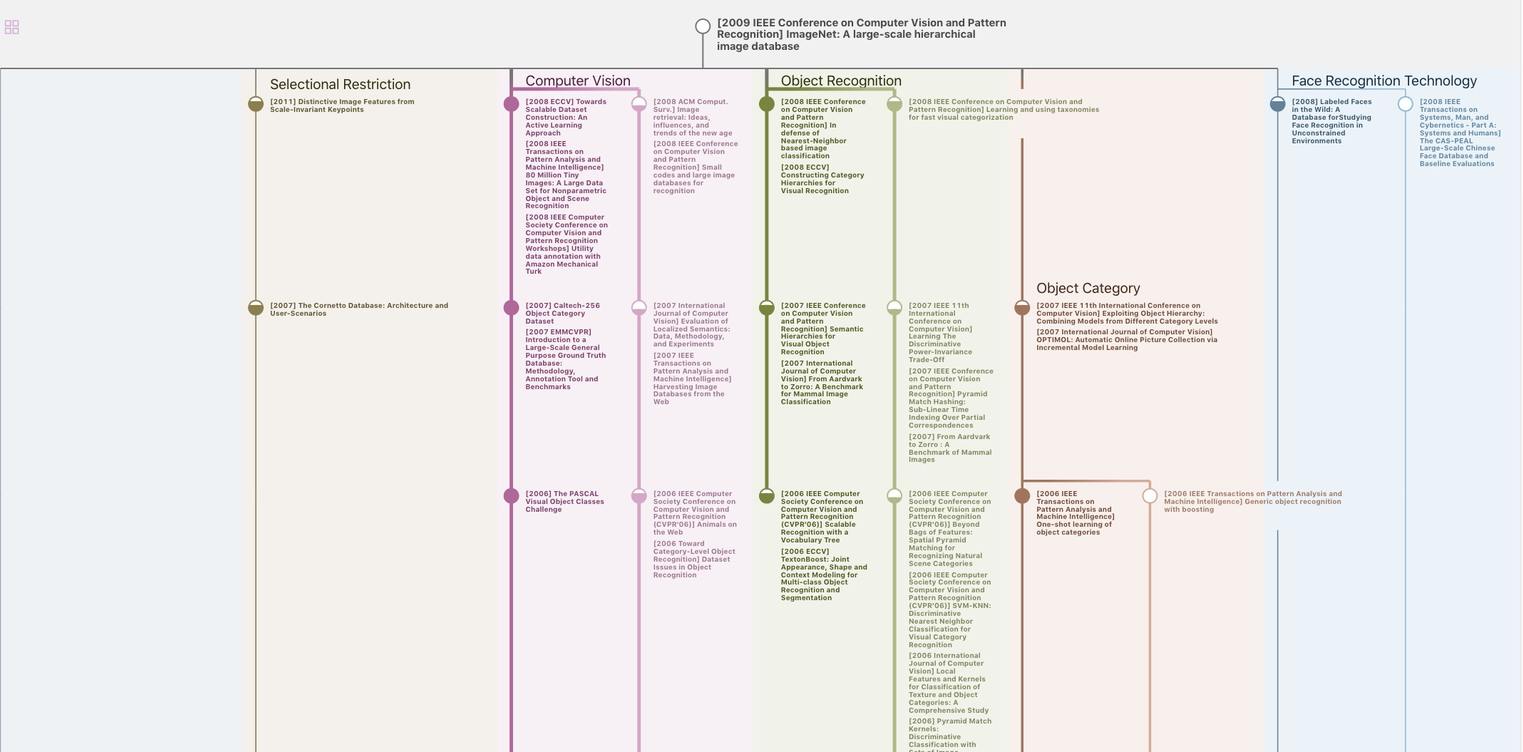
生成溯源树,研究论文发展脉络
Chat Paper
正在生成论文摘要