Design and Analysis of a Novel Distributed Gradient Neural Network for Solving Consensus Problems in a Predefined Time
IEEE TRANSACTIONS ON NEURAL NETWORKS AND LEARNING SYSTEMS(2024)
摘要
In this article, a novel distributed gradient neural network (DGNN) with predefined-time convergence (PTC) is proposed to solve consensus problems widely existing in multiagent systems (MASs). Compared with previous gradient neural networks (GNNs) for optimization and computation, the proposed DGNN model works in a nonfully connected way, in which each neuron only needs the information of neighbor neurons to converge to the equilibrium point. The convergence and asymptotic stability of the DGNN model are proved according to the Lyapunov theory. In addition, based on a relatively loose condition, three novel nonlinear activation functions are designed to speedup the DGNN model to PTC, which is proved by rigorous theory. Computer numerical results further verify the effectiveness, especially the PTC, of the proposed nonlinearly activated DGNN model to solve various consensus problems of MASs. Finally, a practical case of the directional consensus is presented to show the feasibility of the DGNN model and a corresponding connectivity-testing example is given to verify the influence on the convergence speed.
更多查看译文
关键词
Convergence,Laplace equations,Asymptotic stability,Adaptation models,Mathematical models,Consensus protocol,Computational modeling,Asymptotic stability,consensus,gradient neural network (GNN),predefined-time convergence (PTC)
AI 理解论文
溯源树
样例
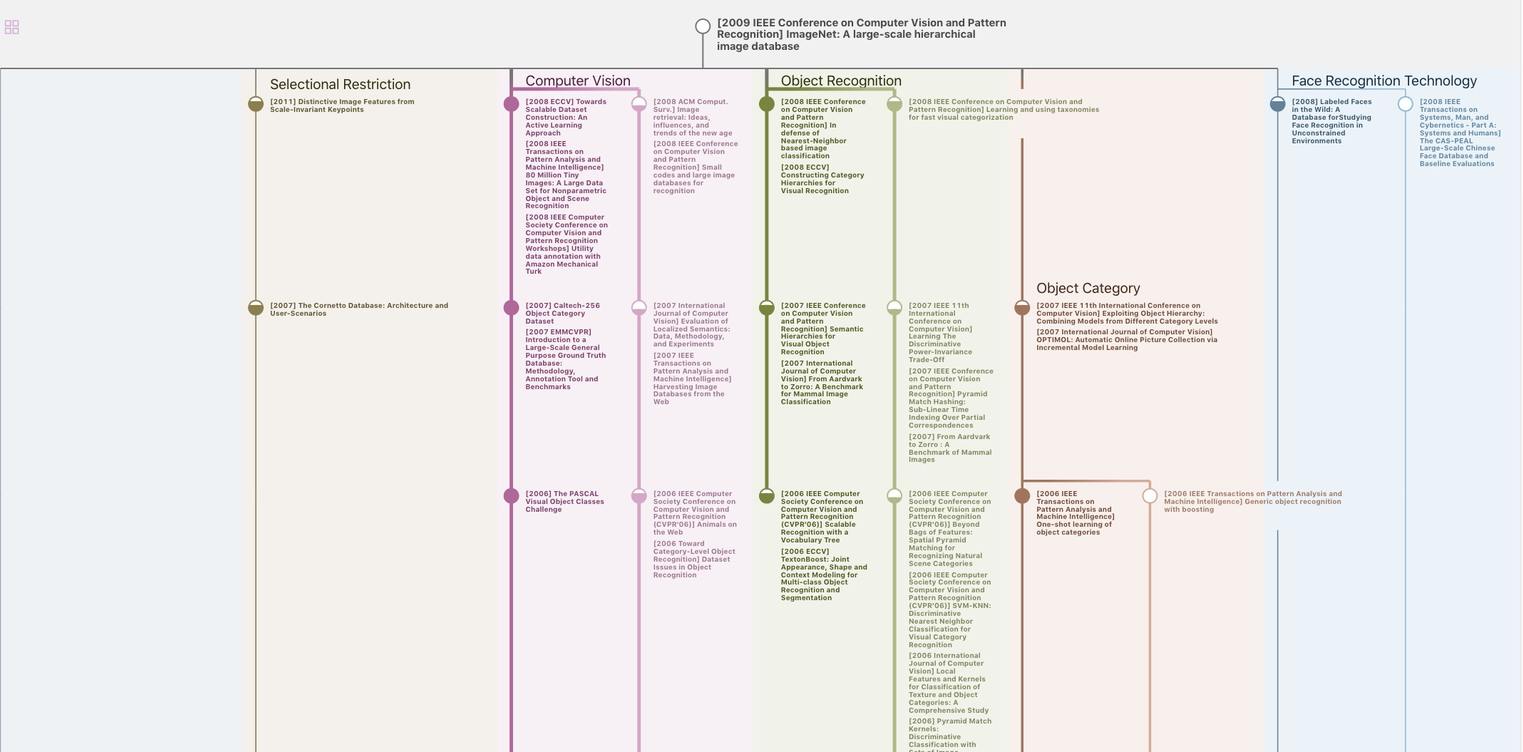
生成溯源树,研究论文发展脉络
Chat Paper
正在生成论文摘要