Four-Class Classification of Neuropsychiatric Disorders by Use of Functional Near-Infrared Spectroscopy Derived Biomarkers
SENSORS(2022)
摘要
Diagnosis of most neuropsychiatric disorders relies on subjective measures, which makes the reliability of final clinical decisions questionable. The aim of this study was to propose a machine learning-based classification approach for objective diagnosis of three disorders of neuropsychiatric or neurological origin with functional near-infrared spectroscopy (fNIRS) derived biomarkers. Thirteen healthy adolescents and sixty-seven patients who were clinically diagnosed with migraine, obsessive compulsive disorder, or schizophrenia performed a Stroop task, while prefrontal cortex hemodynamics were monitored with fNIRS. Hemodynamic and cognitive features were extracted for training three supervised learning algorithms (naive bayes (NB), linear discriminant analysis (LDA), and support vector machines (SVM)). The performance of each algorithm in correctly predicting the class of each participant across the four classes was tested with ten runs of a ten-fold cross-validation procedure. All algorithms achieved four-class classification performances with accuracies above 81% and specificities above 94%. SVM had the highest performance in terms of accuracy (85.1 +/- 1.77%), sensitivity (84 +/- 1.7%), specificity (95 +/- 0.5%), precision (86 +/- 1.6%), and F1-score (85 +/- 1.7%). fNIRS-derived features have no subjective report bias when used for automated classification purposes. The presented methodology might have significant potential for assisting in the objective diagnosis of neuropsychiatric disorders associated with frontal lobe dysfunction.
更多查看译文
关键词
fNIRS, BCI, classification, schizophrenia, obsessive compulsive disorder, migraine, Stroop test
AI 理解论文
溯源树
样例
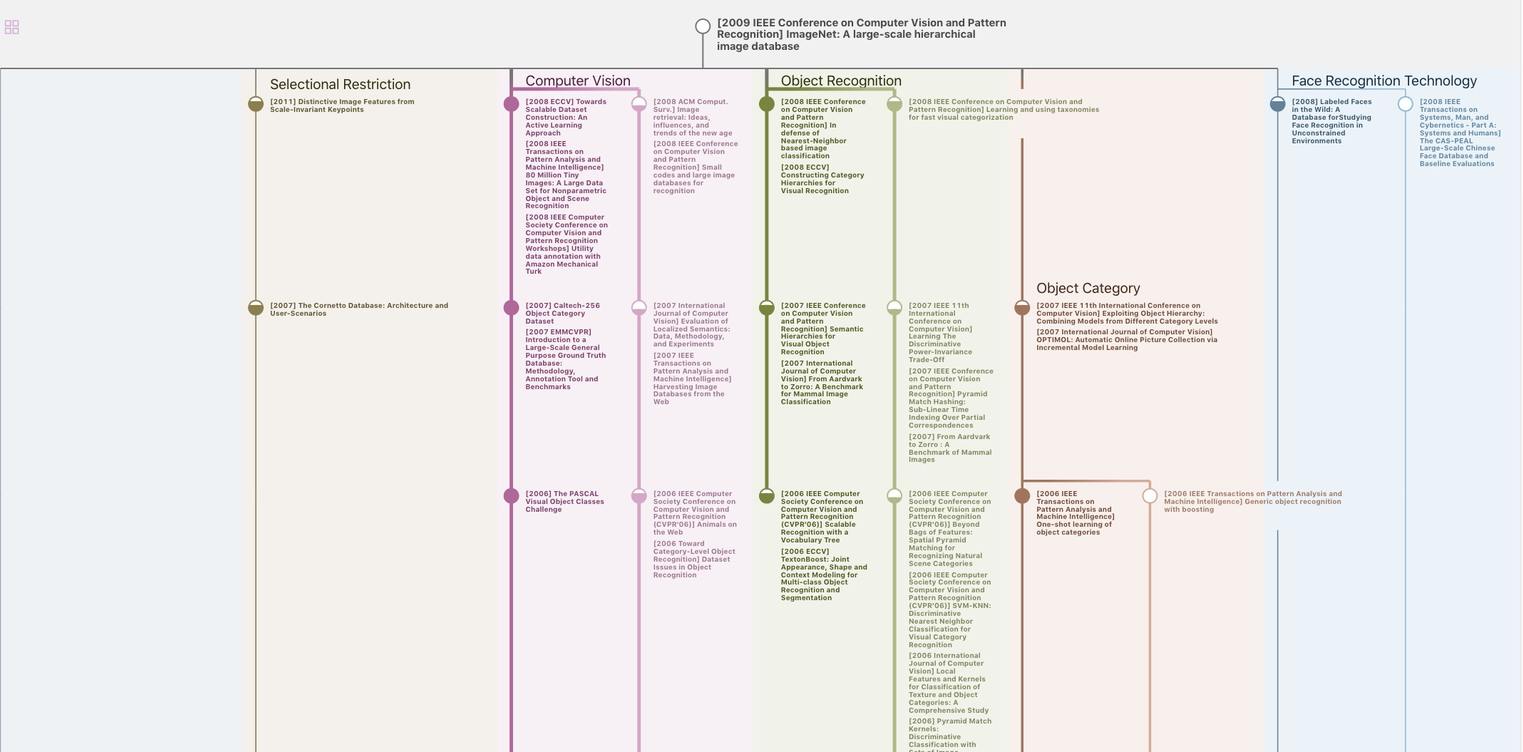
生成溯源树,研究论文发展脉络
Chat Paper
正在生成论文摘要