Multiscale transunet + + : dense hybrid U-Net with transformer for medical image segmentation
Signal, Image and Video Processing(2022)
摘要
Automatic medical image segmentation as assistance to doctors is important for diagnosis and treatment of various diseases. TransUNet that integrates the advantages of transformer and CNN has achieved success in medical image segmentation tasks. However, TransUNet simply combines feature maps between encoder and decoder via skip connections at the same resolution, which leads to be an unnecessarily restrictive fusion design. Moreover, the positional encoding and input tokens in standard transformer blocks of TransUNet have a fixed scale, which are not suitable for dense prediction. To alleviate the above problems, in this paper, we propose a novel architecture named multiscale TransUNet + + (MS-TransUNet + +), which employs a multiscale and flexible feature fusion scheme between encoder and decoder at different levels. The novel skip connections densely bridge the extracted feature representations with different resolutions, and the hybrid CNN-Transformer encoder with long-range dependencies directly passes the high-level features to each stage of decoder. Besides, in order to obtain more effective feature representations, an efficient multi-scale visual transformer is introduced for feature encoder. More importantly, we employ a weighted loss function composed of focal, multiscale structure similarity and Jaccard index to penalize the training error of medical image segmentation, jointly realizing pixel-level, patch-level and map-level optimization. Extensive experimental results demonstrate that our proposed multiscale TransUNet + + can achieve competitive performance for prostate MR and liver CT image segmentation.
更多查看译文
关键词
Medical image segmentation, CNN, Transformer, Weighted loss function
AI 理解论文
溯源树
样例
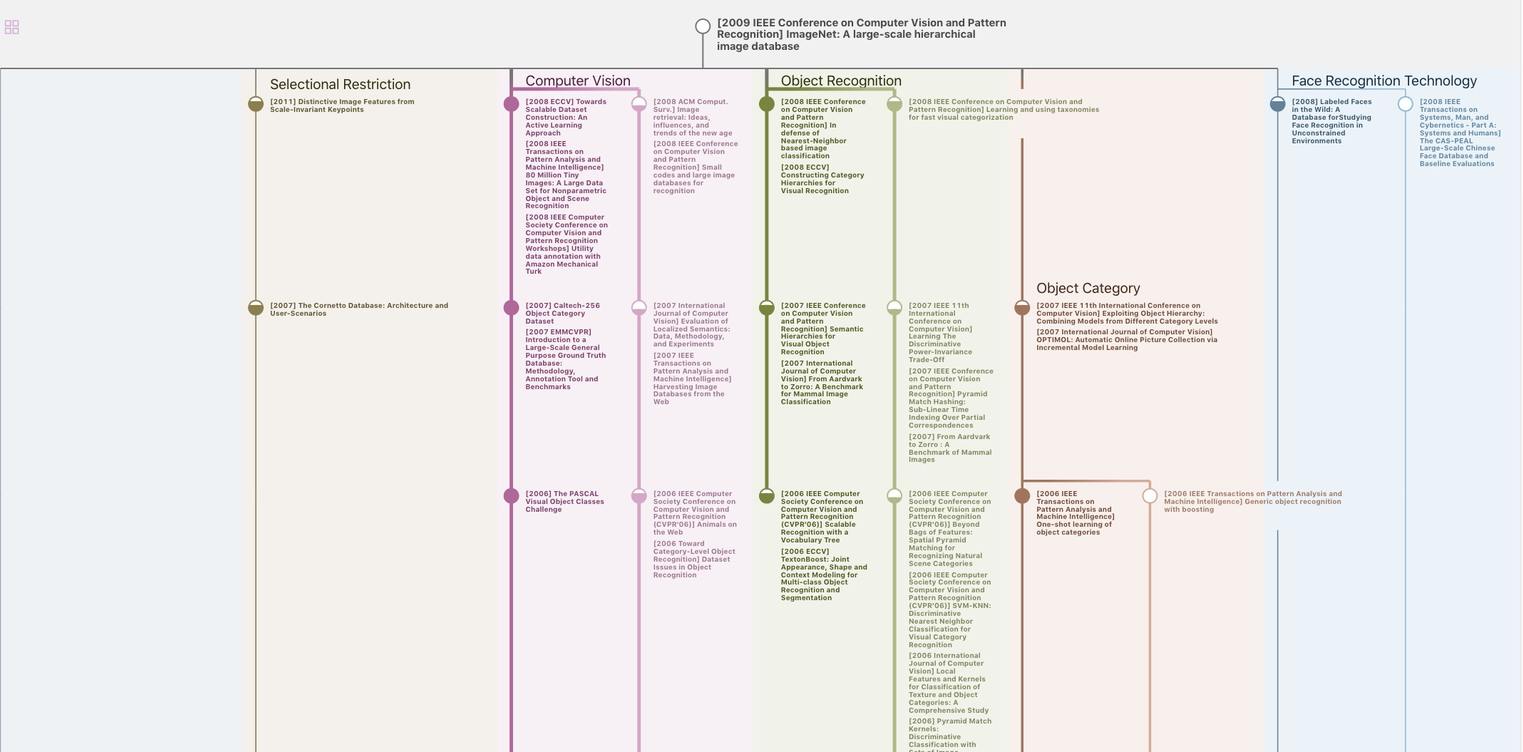
生成溯源树,研究论文发展脉络
Chat Paper
正在生成论文摘要