Eliminating the Effects of Jump Phenomenon for WiFi Driving Behavior Recognition
2022 IEEE 12th International Conference on Electronics Information and Emergency Communication (ICEIEC)(2022)
摘要
Feature extraction-based methods are frequently used for driving behavior recognition systems using the fine-grained CSI from the PHY layer. However, we discover a jump phenomenon: there are switches of frequency-selected fading in random individual time slots, called jump points. Moreover, the jump phenomenon causes the extracted feature values to be inaccurate, resulting in erroneous behavior classification. In this paper, to eliminate the effects of the jump phenomenon, the Pearson correlation coefficient is introduced to detect the position of jump points. Meanwhile, by transforming this problem as an optimization problem, we propose a similarity calculation model and introduce a nested switch-mode firefly algorithm to correct the jump point. The method is tested by the experiments conducted in three indoor scenarios. The results indicate that the proposed method can effectively solve the problem caused by the jump phenomenon and improve the stability of eigenvalues.
更多查看译文
关键词
feature extraction,jump phenomenon,pearson correlation coefficient,similarity calculation,firefly algorithm
AI 理解论文
溯源树
样例
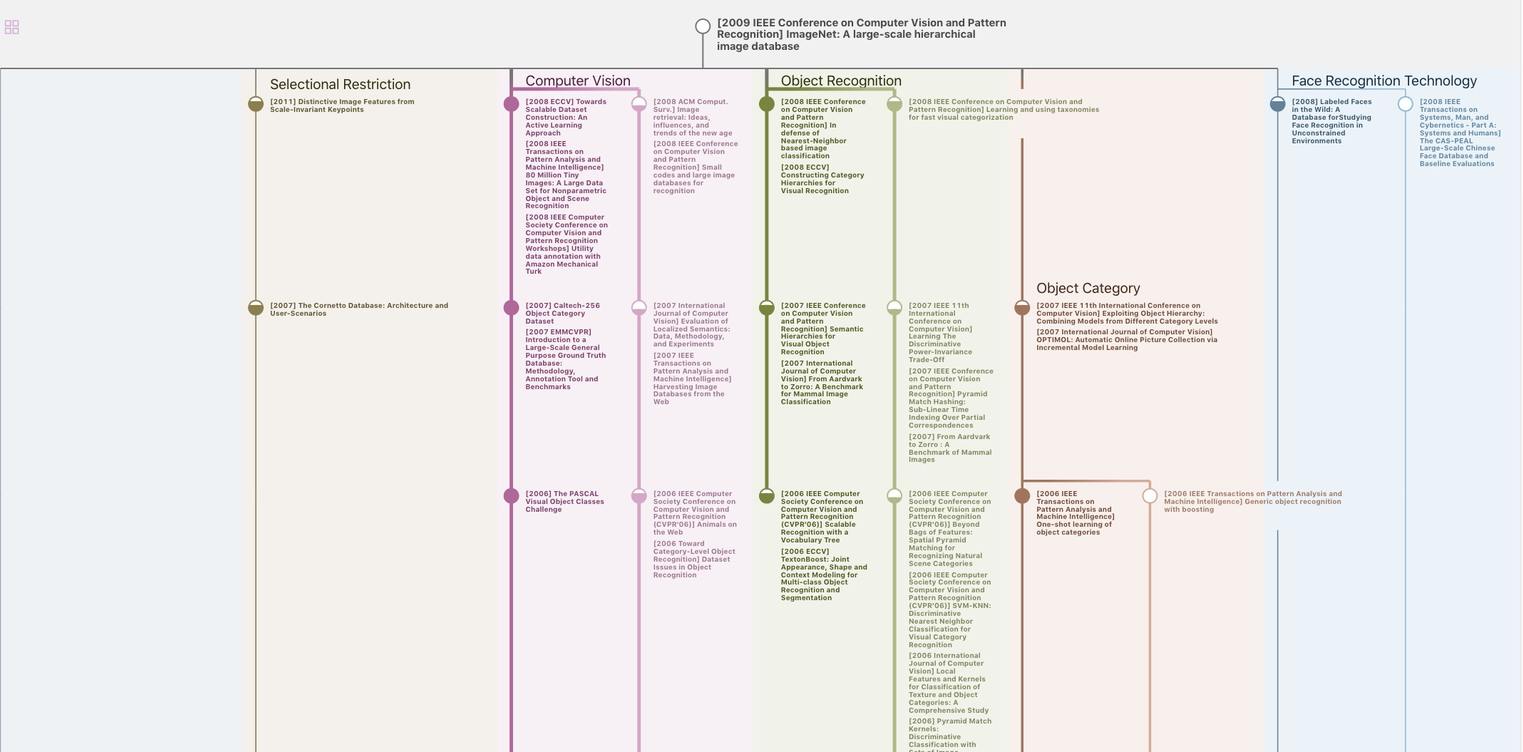
生成溯源树,研究论文发展脉络
Chat Paper
正在生成论文摘要