Ridge Regression Based on Koropov Sequence Initialized Archimedes Optimization Algorithm
2022 IEEE 12th International Conference on Electronics Information and Emergency Communication (ICEIEC)(2022)
摘要
Ridge regression is a biased estimation regression method specially used for collinear data analysis. It is in essence an improved least square estimation method by abandoning the unbiased nature of least square method, the regression coefficient can be obtained at the cost of losing some information and reducing accuracy. It is more practical and reliable, for it can fit of ill conditioned data better than the least square method. The main idea of ridge regression is to use L2 regularization to lose some information and reduce the accuracy, so as to obtain the best parameters. In order to better optimize the loss function to find the best parameters, gradient descent (GD) or stochastic gradient descent (SGD) method are usually used to find the most appropriate solution. However, the gradient dependent methods have two significant disadvantages: (1) it is easy to fall into local minimum; (2) with the progress of iteration, the closer it is to the optimal solution, the more prone it is to sawtooth effect. In order to improve the performance of global convergence in the process of optimization, in this paper, a Koropov sequence initialized Archimedes optimization algorithm (KSAOA) has been proposed to replace the GD and SGD in solving the loss function of ridge regression, and its advantage in overcoming the disadvantage of gradient dependent methods and its effectiveness is verified by numerical experiments.
更多查看译文
关键词
ridge regression,gradient descent (GD),Archimede optimization algorithm,least square method,Koropov sequence
AI 理解论文
溯源树
样例
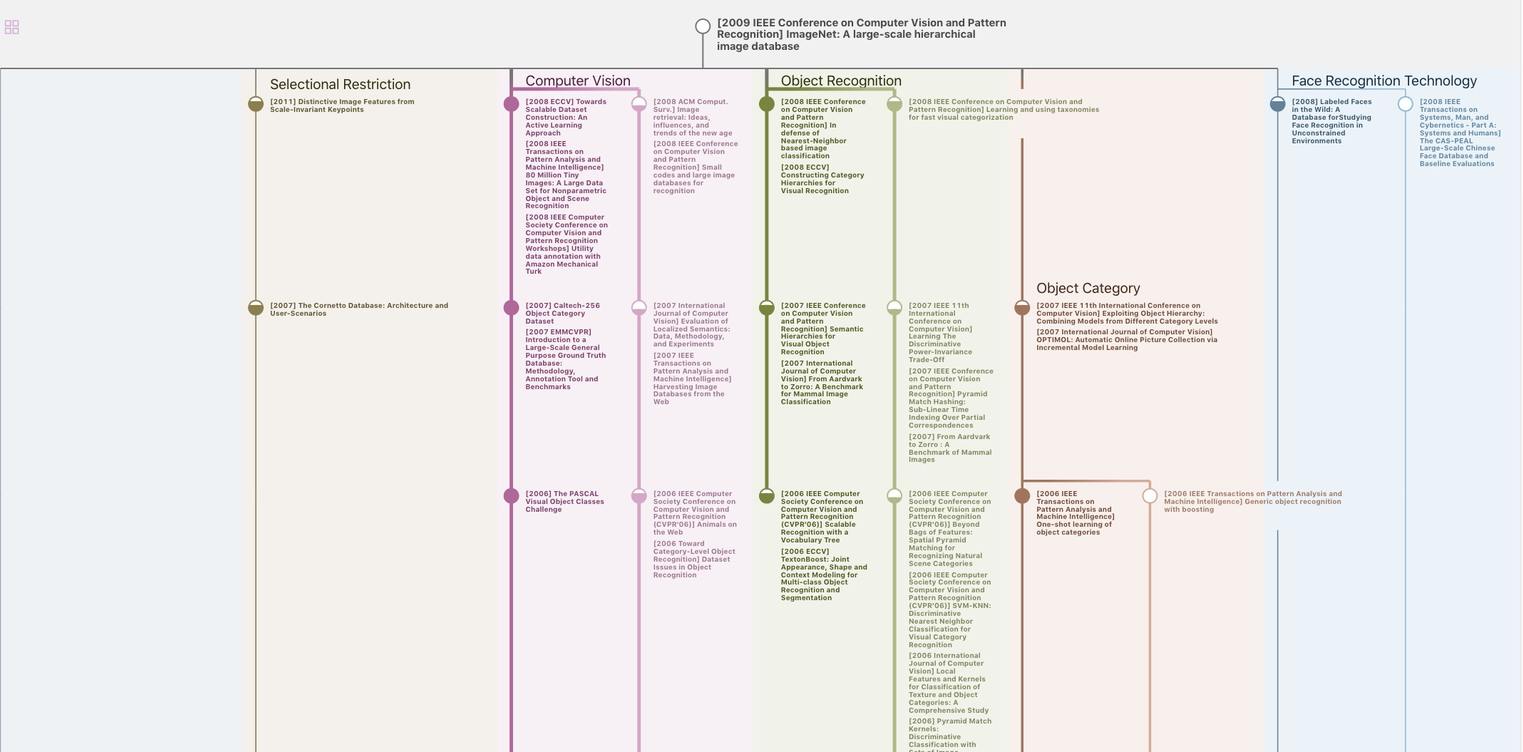
生成溯源树,研究论文发展脉络
Chat Paper
正在生成论文摘要