Towards Large-Scale Small Object Detection: Survey and Benchmarks
arxiv(2023)
摘要
With the rise of deep convolutional neural networks, object detection has achieved prominent advances in past years. However, such prosperity could not camouflage the unsatisfactory situation of Small Object Detection (SOD), one of the notoriously challenging tasks in computer vision, owing to the poor visual appearance and noisy representation caused by the intrinsic structure of small targets. In addition, large-scale dataset for benchmarking small object detection methods remains a bottleneck. In this paper, we first conduct a thorough review of small object detection. Then, to catalyze the development of SOD, we construct two large-scale Small Object Detection dAtasets (SODA), SODA-D and SODA-A, which focus on the Driving and Aerial scenarios respectively. SODA-D includes 24828 high-quality traffic images and 278433 instances of nine categories. For SODA-A, we harvest 2513 high resolution aerial images and annotate 872069 instances over nine classes. The proposed datasets, as we know, are the first-ever attempt to large-scale benchmarks with a vast collection of exhaustively annotated instances tailored for multi-category SOD. Finally, we evaluate the performance of mainstream methods on SODA. We expect the released benchmarks could facilitate the development of SOD and spawn more breakthroughs in this field.
更多查看译文
关键词
Object detection,Surveys,Feature extraction,Benchmark testing,Deep learning,Task analysis,Pedestrians,Benchmark,convolutional neural networks,deep learning,object detection,small object detection
AI 理解论文
溯源树
样例
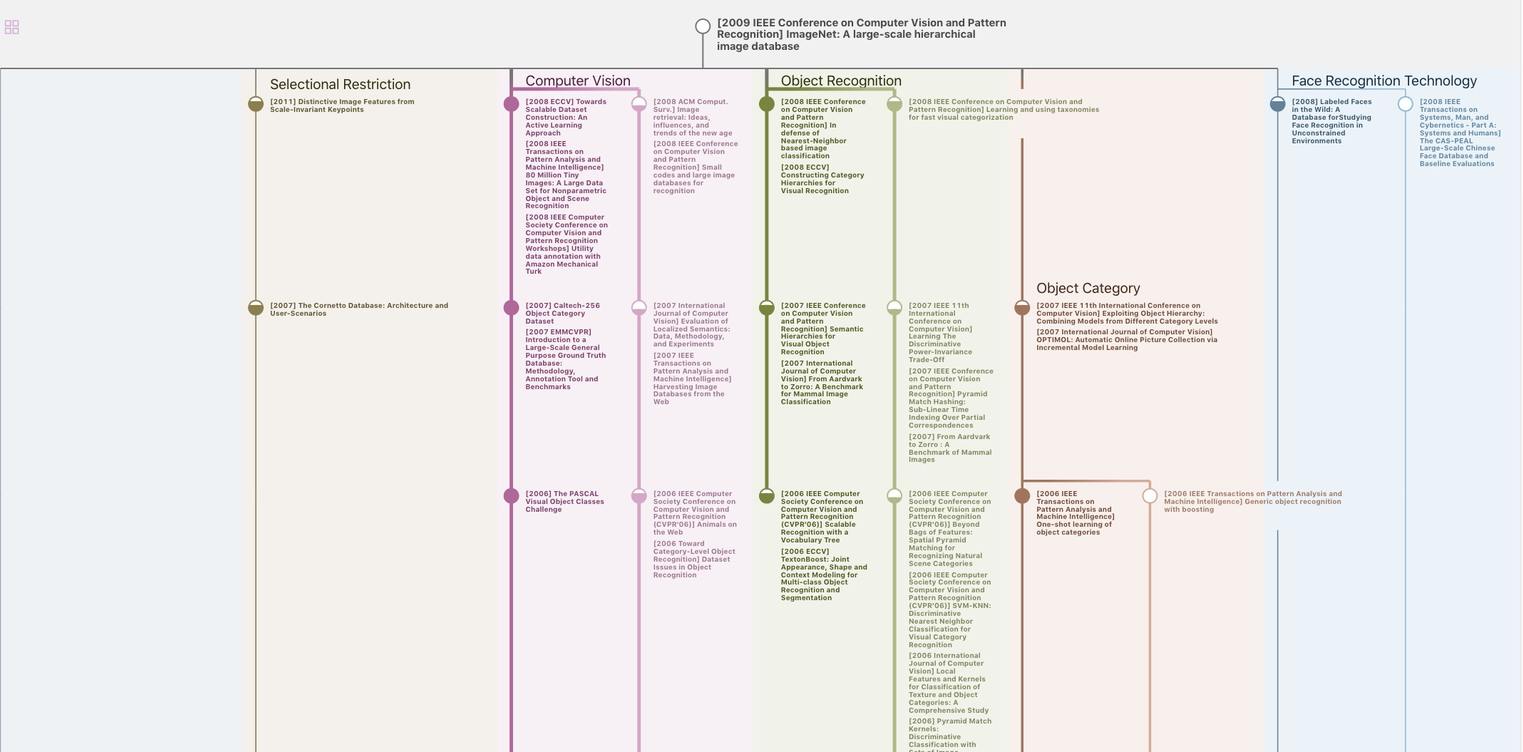
生成溯源树,研究论文发展脉络
Chat Paper
正在生成论文摘要