Frameworks and Results in Distributionally Robust Optimization
Open Journal of Mathematical Optimization(2022)
Abstract
The concepts of risk aversion, chance-constrained optimization, and robust optimization have developed significantly over the last decade. The statistical learning community has also witnessed a rapid theoretical and applied growth by relying on these concepts. A modeling framework, called distributionally robust optimization (DRO), has recently received significant attention in both the operations research and statistical learning communities. This paper surveys main concepts and contributions to DRO, and relationships with robust optimization, risk aversion, chance-constrained optimization, and function regularization. Various approaches to model the distributional ambiguity and their calibrations are discussed. The paper also describes the main solution techniques used to the solve the resulting optimization problems.
MoreTranslated text
Key words
90C15,90C22,90C25,90C30,90C34,90C90,68T37,68T05,Distributionally robust optimization, Robust optimization, Stochastic optimization, Risk-averse optimization, Chance-constrained optimization, Statistical learning
AI Read Science
Must-Reading Tree
Example
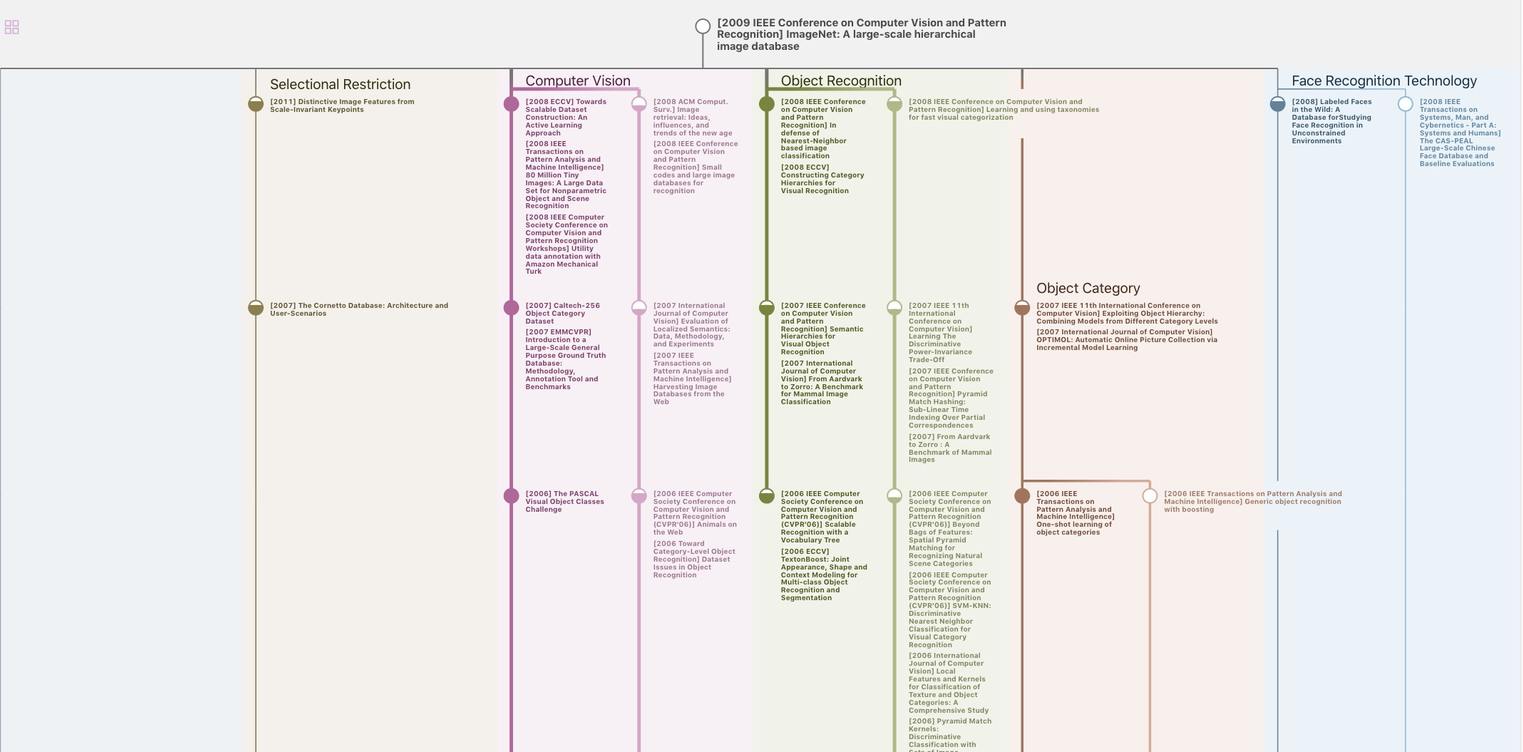
Generate MRT to find the research sequence of this paper
Chat Paper
Summary is being generated by the instructions you defined