An Enhanced Multiple Sclerosis Disease Diagnosis via an Ensemble Approach
DIAGNOSTICS(2022)
摘要
Multiple Sclerosis (MS) is a disease attacking the central nervous system. According to MS Atlas's most recent statistics, there are more than 2.8 million people worldwide diagnosed with MS. Recently, studies started to explore machine learning techniques to predict MS using various data. The objective of this paper is to develop an ensemble approach for diagnosis of MS using gene expression profiles, while handling the class imbalance problem associated with the data. A hierarchical ensemble approach employing voting and boosting techniques is proposed. This approach adopts a heterogeneous voting approach using two base learners, random forest and support vector machine. Experiments show that our approach outperforms state-of-the-art methods, with the highest recorded accuracy being 92.81% and 93.5% with BoostFS and DEGs for feature selection, respectively. Conclusively, the proposed approach is able to efficiently diagnose MS using the gene expression profiles that are more relevant to the disease. The approach is not merely an ensemble classifier outperforming previous work; it also identifies differentially expressed genes between normal samples and patients with multiple sclerosis using a genome-wide expression microarray. The results obtained show that the proposed approach is an efficient diagnostic tool for MS.
更多查看译文
关键词
ensemble learning, multiple sclerosis, diagnosis, gene expression, differentially expressed genes
AI 理解论文
溯源树
样例
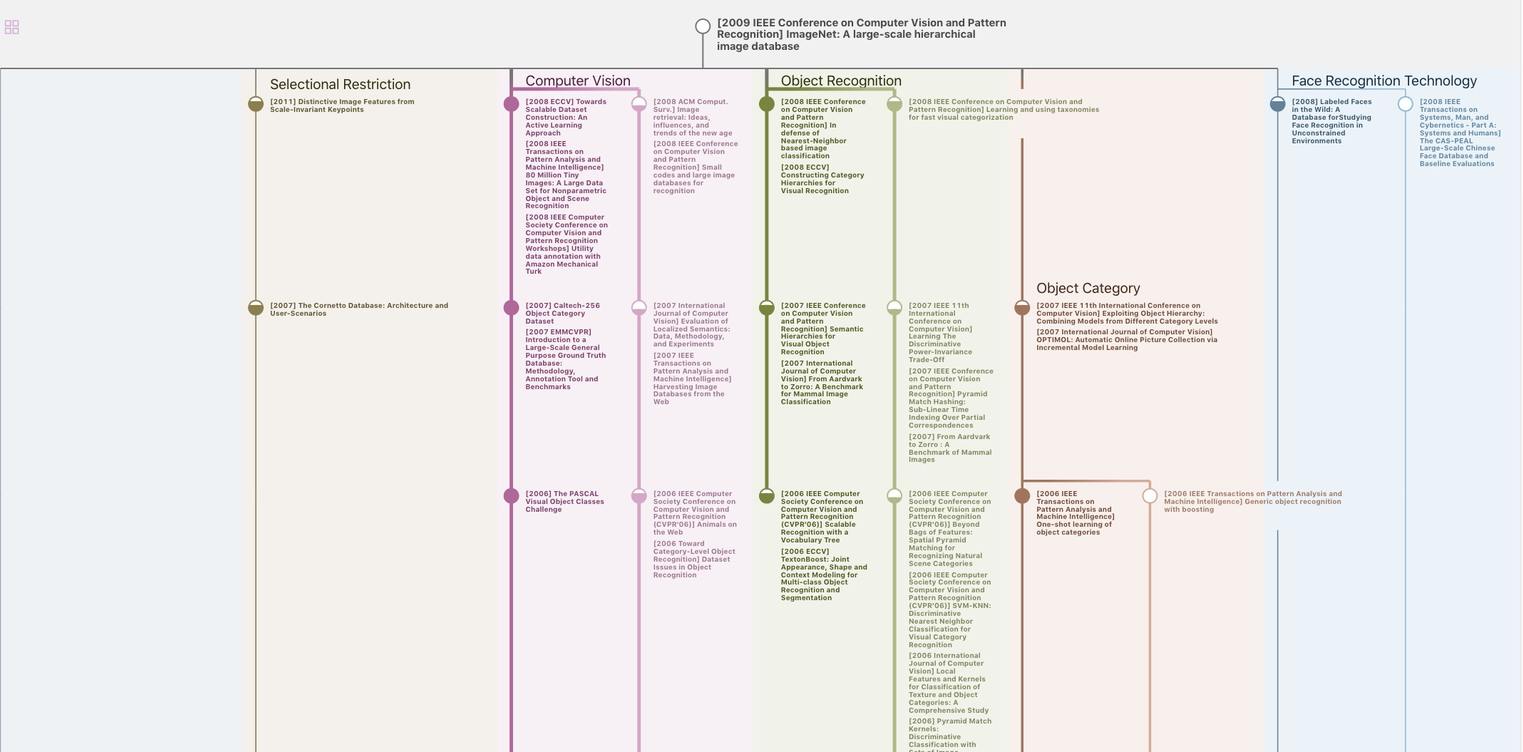
生成溯源树,研究论文发展脉络
Chat Paper
正在生成论文摘要