Privacy-Preserving Design of Scalar LQG Control
ENTROPY(2022)
摘要
This paper studies the agent identity privacy problem in the scalar linear quadratic Gaussian (LQG) control system. The agent identity is a binary hypothesis: Agent A or Agent B. An eavesdropper is assumed to make a hypothesis testing the agent identity based on the intercepted environment state sequence. The privacy risk is measured by the Kullback-Leibler divergence between the probability distributions of state sequences under two hypotheses. By taking into account both the accumulative control reward and privacy risk, an optimization problem of the policy of Agent B is formulated. This paper shows that the optimal deterministic privacy-preserving LQG policy of Agent B is a linear mapping. A sufficient condition is given to guarantee that the optimal deterministic privacy-preserving policy is time-invariant in the asymptotic regime. It is also shown that adding an independent Gaussian random process noise to the linear mapping of the optimal deterministic privacy-preserving policy cannot improve the performance of Agent B. The numerical experiments justify the theoretic results and illustrate the reward-privacy trade-off.
更多查看译文
关键词
control-privacy trade-off, hypothesis testing, Kullback-Leibler divergence, optimal control policy, privacy risk analysis
AI 理解论文
溯源树
样例
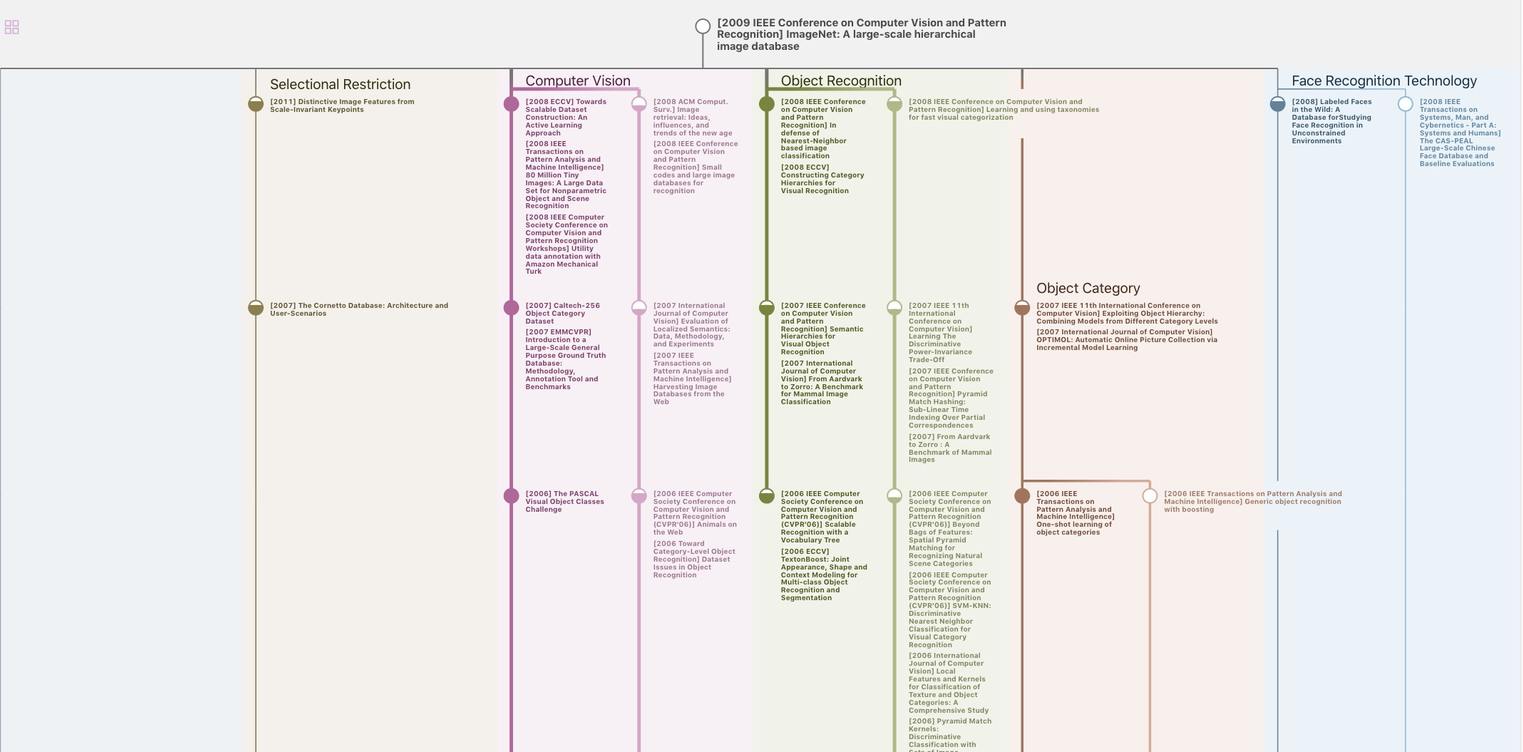
生成溯源树,研究论文发展脉络
Chat Paper
正在生成论文摘要