BeCAPTCHA-Type: Biometric Keystroke Data Generation for Improved Bot Detection
arxiv(2023)
摘要
This work proposes a data driven learning model for the synthesis of keystroke biometric data. The proposed method is compared with two statistical approaches based on Universal and User-dependent models. These approaches are validated on the bot detection task, using the keystroke synthetic data to improve the training process of keystroke-based bot detection systems. Our experimental framework considers a dataset with 136 million keystroke events from 168 thousand subjects. We have analyzed the performance of the three synthesis approaches through qualitative and quantitative experiments. Different bot detectors are considered based on several supervised classifiers (Support Vector Machine, Random Forest, Gaussian Naive Bayes and a Long Short-Term Memory network) and a learning framework including human and synthetic samples. The experiments demonstrate the realism of the synthetic samples. The classification results suggest that in scenarios with large labeled data, these synthetic samples can be detected with high accuracy. However, in few-shot learning scenarios it represents an important challenge. Furthermore, these results show the great potential of the presented models.
更多查看译文
关键词
BeCAPTCHA-type,biometric keystroke data generation,bot detectors,experimental framework,Gaussian Naive Bayes,keystroke biometric data,keystroke events,keystroke synthetic data,keystroke-based bot detection systems,learning framework,learning model,long short-term memory network,massive data,quantitative experiments,statistical approaches,support vector machine,synthesis approaches,synthetic samples,training process,User-dependent models
AI 理解论文
溯源树
样例
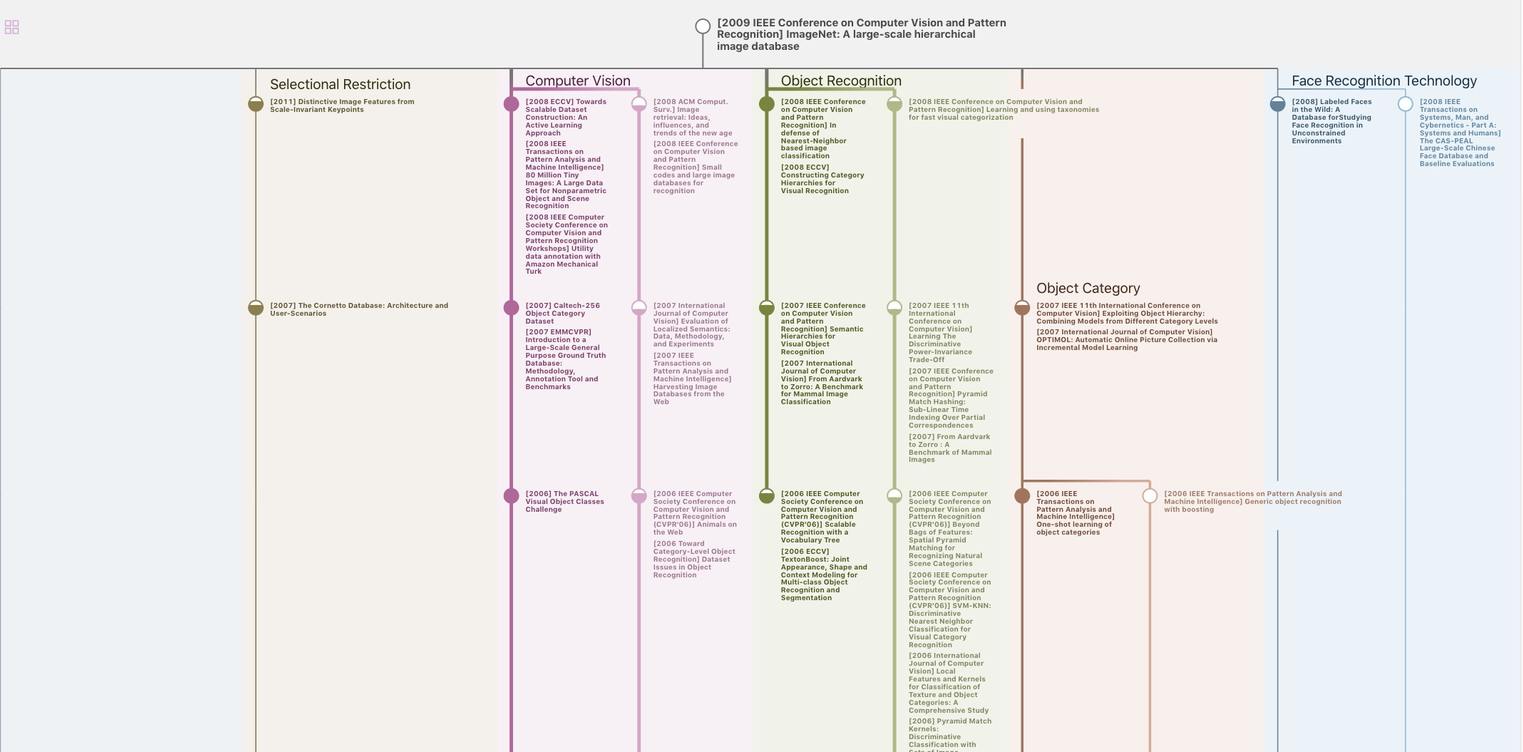
生成溯源树,研究论文发展脉络
Chat Paper
正在生成论文摘要