Marker and source-marker reprogramming of Most Permissive Boolean networks and ensembles with BoNesis
arxiv(2023)
摘要
Boolean networks (BNs) are discrete dynamical systems with applications to the modeling of cellular behaviors. In this paper, we demonstrate how the software BoNesis can be employed to exhaustively identify combinations of perturbations which enforce properties on their fixed points and attractors. We consider marker properties, which specify that some components are fixed to a specific value. We study 4 variants of the marker reprogramming problem: the reprogramming of fixed points, of minimal trap spaces, and of fixed points and minimal trap spaces reachable from a given initial configuration with the most permissive update mode. The perturbations consist of fixing a set of components to a fixed value. They can destroy and create new attractors. In each case, we give an upper bound on their theoretical computational complexity, and give an implementation of the resolution using the BoNesis Python framework. Finally, we lift the reprogramming problems to ensembles of BNs, as supported by BoNesis, bringing insight on possible and universal reprogramming strategies. This paper can be executed and modified interactively.
更多查看译文
关键词
most permissive boolean networks,ensembles,source-marker
AI 理解论文
溯源树
样例
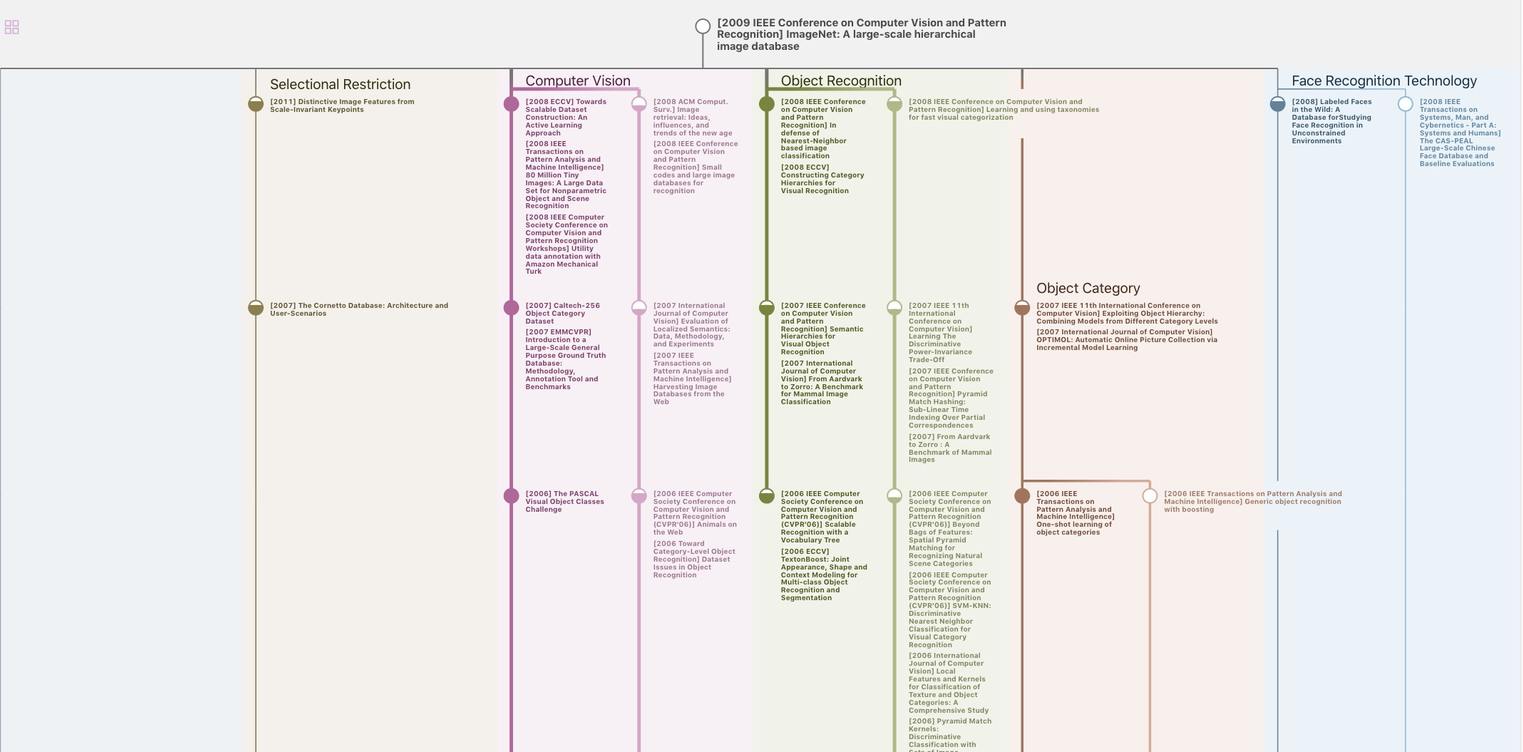
生成溯源树,研究论文发展脉络
Chat Paper
正在生成论文摘要