On Missing Labels, Long-tails and Propensities in Extreme Multi-label Classification
KDD '22: Proceedings of the 28th ACM SIGKDD Conference on Knowledge Discovery and Data Mining(2022)
摘要
The propensity model introduced by Jain et al has become a standard approach for dealing with missing and long-tail labels in extreme multi-label classification (XMLC). In this paper, we critically revise this approach showing that despite its theoretical soundness, its application in contemporary XMLC works is debatable. We exhaustively discuss the flaws of the propensity-based approach, and present several recipes, some of them related to solutions used in search engines and recommender systems, that we believe constitute promising alternatives to be followed in XMLC.
更多查看译文
关键词
missing labels,classification,long-tails,multi-label
AI 理解论文
溯源树
样例
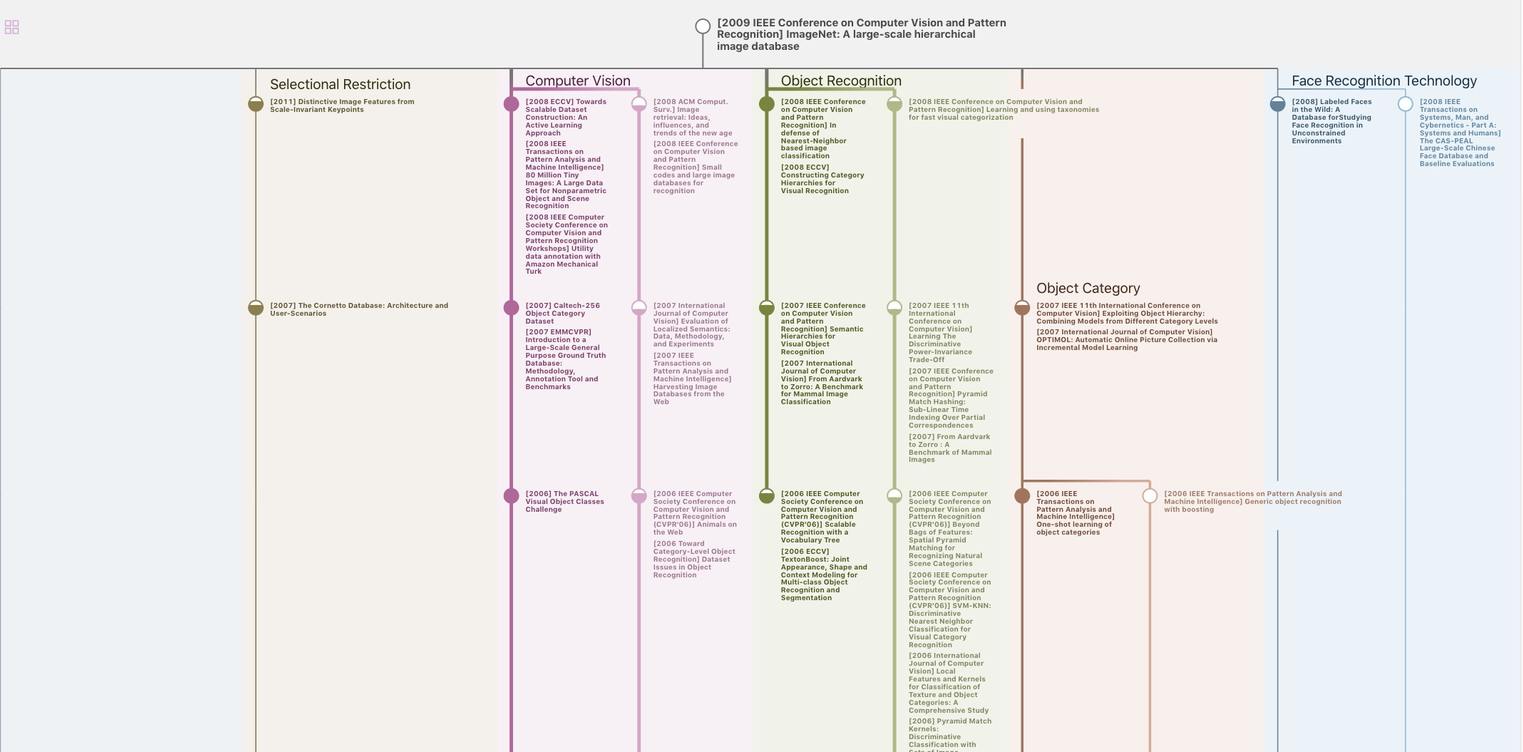
生成溯源树,研究论文发展脉络
Chat Paper
正在生成论文摘要