Bayesian Sub-Pixel Mapping of Hyperspectral Imagery via Discrete Endmember Variability Mixture Model and Markov Random Field
IEEE Journal of Selected Topics in Applied Earth Observations and Remote Sensing(2022)
摘要
Although Bayesian methods have been very effective for spatial-spectral analysis of hyperspectral imagery (HSI), they had not been fully explored for enhanced subpixel mapping (SPM) by simultaneously considering several key issues, i.e., endmemher variability, the discrete nature of subpixel class labels, and the spatial information in HSI. Therefore, we propose a new Bayesian SPM method based on the discrete endmember variability mixture model (DEMM) and Markov random field (MRF), which has three main characteristics. First, DENIM allows the advanced SPM by completely accounting for the endmember-abundance patterns of each pixel to accommodate the endmember variability, the discrete hidden class label field of subpixels, while taking into account the noise heterogeneity effect. Second, the discrete class label fields modeled by MRF together with the DEMM, which can be integrated into a novel Bayesian model to better exploit the spatial contextual and spectral information. Third, the resulting Bayesian model can he efficiently solved by a designed expectation-maximization iteration, where B-step estimates the suhpixel class label field using a simulated annealing algorithm and M-step estimates the endmembers for each pixel in HSI using the alternating non-negative least squares approach. The experimental results on three HSI datasets demonstrate that the proposed approach outperforms previously available SPM techniques.
更多查看译文
关键词
Bayesian subpixel mapping (SPM), discrete endmember variability mixture model (DEMM), expectation-maximization (EM) iteration, hyperspectral imagery (HSI), Markov random field (MRF)
AI 理解论文
溯源树
样例
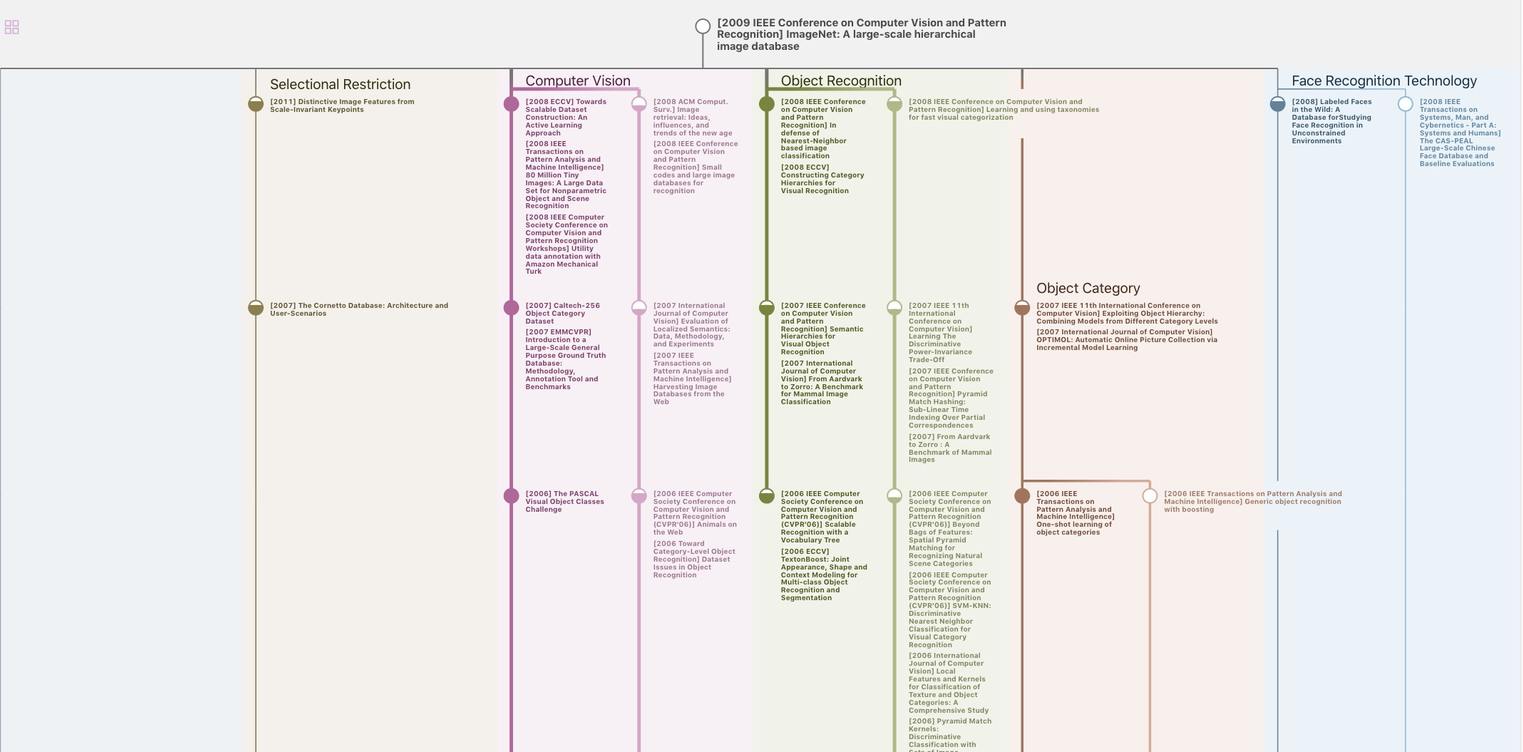
生成溯源树,研究论文发展脉络
Chat Paper
正在生成论文摘要