Offline Reinforcement Learning at Multiple Frequencies
CoRL(2022)
摘要
Leveraging many sources of offline robot data requires grappling with the heterogeneity of such data. In this paper, we focus on one particular aspect of heterogeneity: learning from offline data collected at different control frequencies. Across labs, the discretization of controllers, sampling rates of sensors, and demands of a task of interest may differ, giving rise to a mixture of frequencies in an aggregated dataset. We study how well offline reinforcement learning (RL) algorithms can accommodate data with a mixture of frequencies during training. We observe that the $Q$-value propagates at different rates for different discretizations, leading to a number of learning challenges for off-the-shelf offline RL. We present a simple yet effective solution that enforces consistency in the rate of $Q$-value updates to stabilize learning. By scaling the value of $N$ in $N$-step returns with the discretization size, we effectively balance $Q$-value propagation, leading to more stable convergence. On three simulated robotic control problems, we empirically find that this simple approach outperforms na\"ive mixing by 50% on average.
更多查看译文
关键词
reinforcement,multiple frequencies,learning
AI 理解论文
溯源树
样例
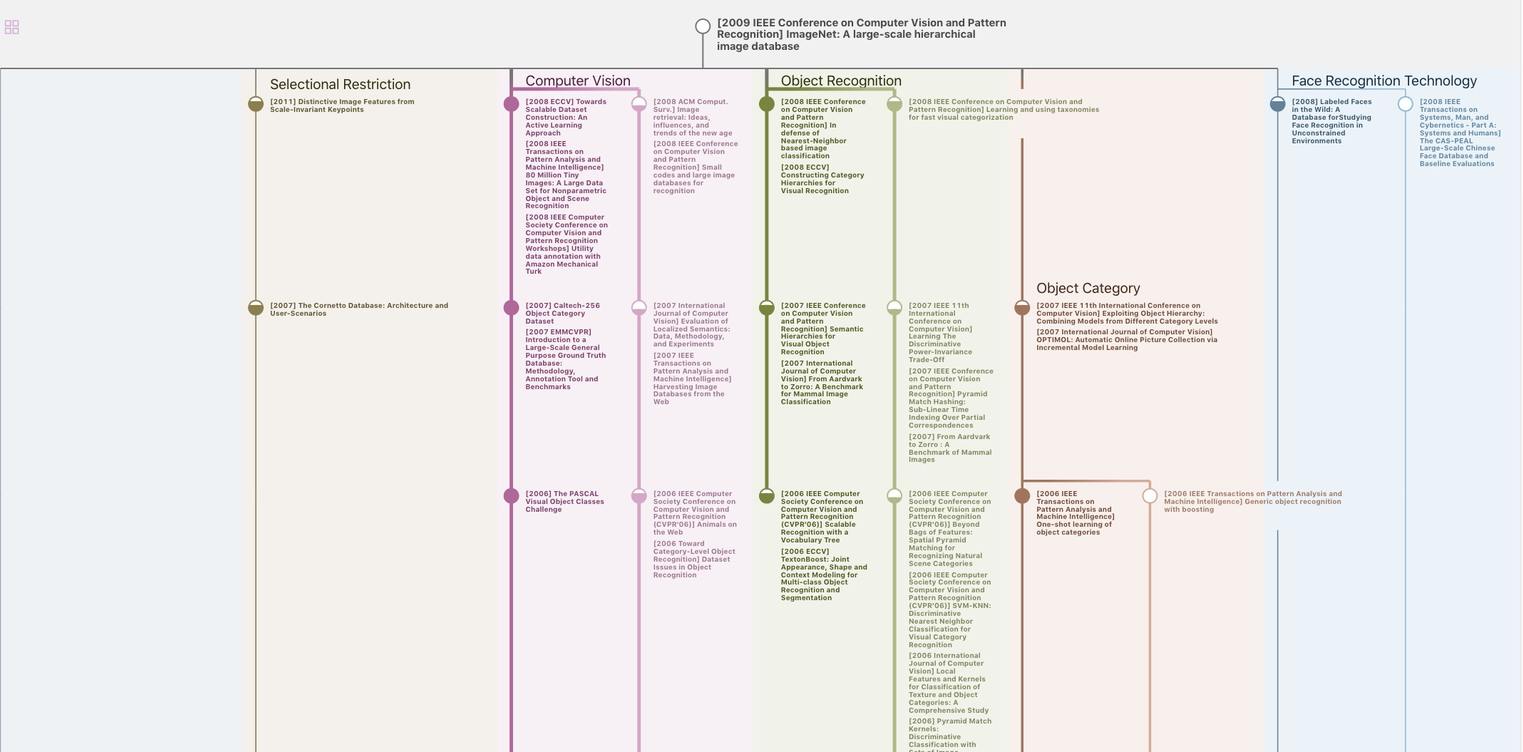
生成溯源树,研究论文发展脉络
Chat Paper
正在生成论文摘要