Plausibility and Faithfulness of Feature Attribution-Based Explanations in Automated Short Answer Scoring
ARTIFICIAL INTELLIGENCE IN EDUCATION, PT I(2022)
Abstract
Automated short answer scoring (SAS) is the task of automatically assigning a score as output to a given input answer. In this work, we tackle the challenging task of outputting the basis (i.e., justification cues) as an explanation for scoring given a defined rubric for assigning a score. In previous studies, researchers explored the performance of scoring via justification identification and constructed their own datasets, but their studies focused solely on limited experiments. On the basis of previous studies, we consider justification identification as an explanation by feature attribution methods for explainable artificial intelligence research. We conduct a comprehensive experiment consisting of multiple explanation generation methods, supervised learning of explanations, and evaluating on two axes, plausibility and faithfulness, which are important for automatic SAS. Our results indicate that we can improve the plausibility of gradient-based methods by supervised learning. However, methods with high plausibility and high faithfulness are still different methods, so it is crucial to select an appropriate method depending on which perspective of explainability is essential.
MoreTranslated text
Key words
Short answer scoring,Explainability,Feature attribution
AI Read Science
Must-Reading Tree
Example
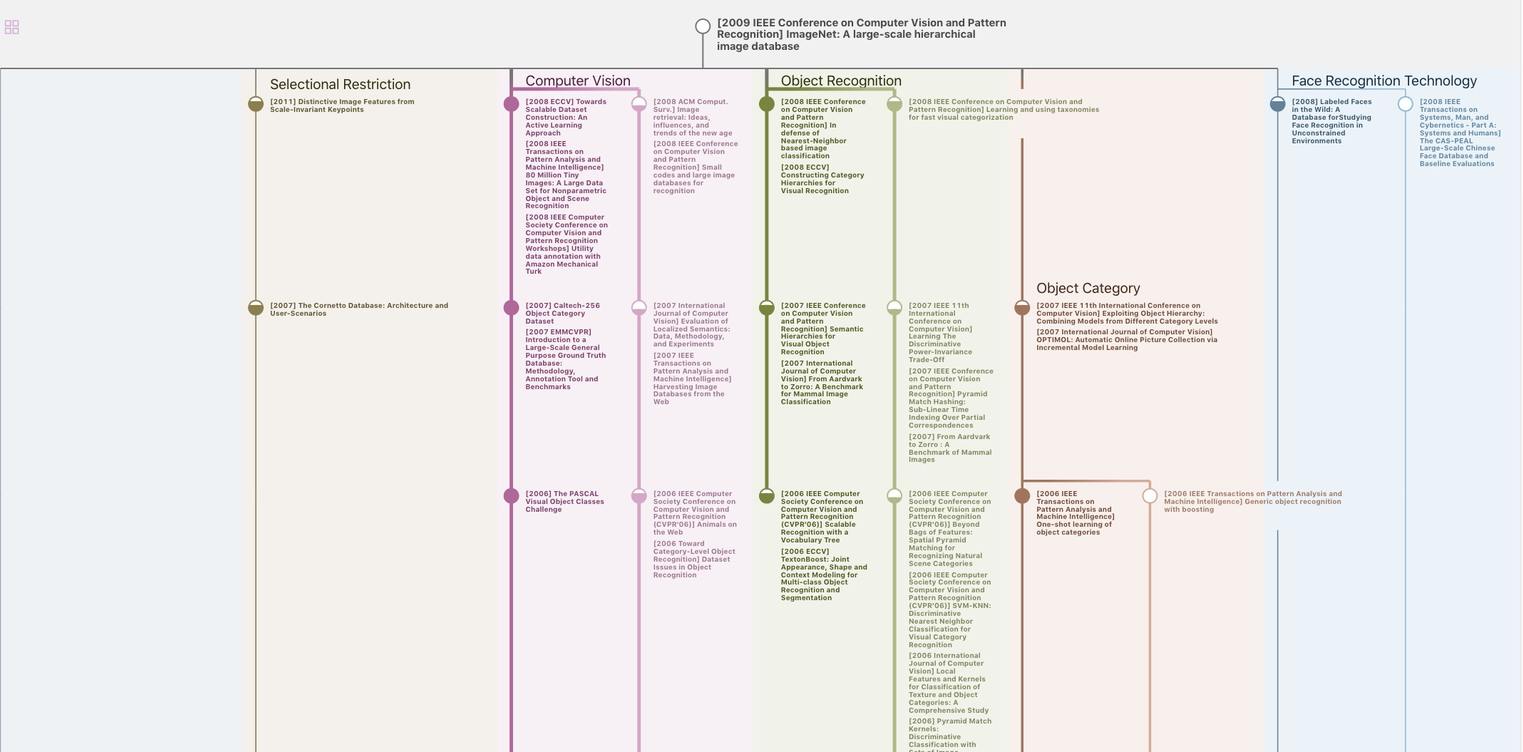
Generate MRT to find the research sequence of this paper
Chat Paper
Summary is being generated by the instructions you defined