Nondestructive evaluation of Zn content in rape leaves using MSSAE and hyperspectral imaging.
Spectrochimica acta. Part A, Molecular and biomolecular spectroscopy(2022)
摘要
Zinc (Zn) content plays a decisive role in plant growth. Accurate management of Zn fertilizer application can promote high-quality development of the oilseed rape industry. This study adopted a deep learning (DL) method to predict the Zn content of oilseed rape leaves using hyperspectral imaging (HSI). The dropout mechanism was introduced to improve the stacked sparse autoencoder (SSAE) and named modified SSAE (MSSAE). MSSAE extracted deep spectral features of samples based on pixel-level spectral information (the wavelength range of the spectrum is 431-962 nm). Subsequently, the deep spectral features were applied as the inputs for support vector regression (SVR) and least squares support vector regression (LSSVR) to predict the Zn content in oilseed rape leaves. In addition, the successive projections algorithm (SPA) and the variable iterative space shrinkage approach (VISSA) were investigated as wavelength selection algorithms for comparison. The results showed that the MSSAE-LSSVR model had the best prediction performance (the coefficient of determination (R2) and root mean square error (RMSE) of the prediction set were 0.9566 and 1.0240 mg/kg, respectively). The overall results showed that the MSSAE was able to extract the deep features of HSI data and validated the possibility of HSI combined with a DL method for nondestructive testing of Zn content in oilseed rape leaves.
更多查看译文
AI 理解论文
溯源树
样例
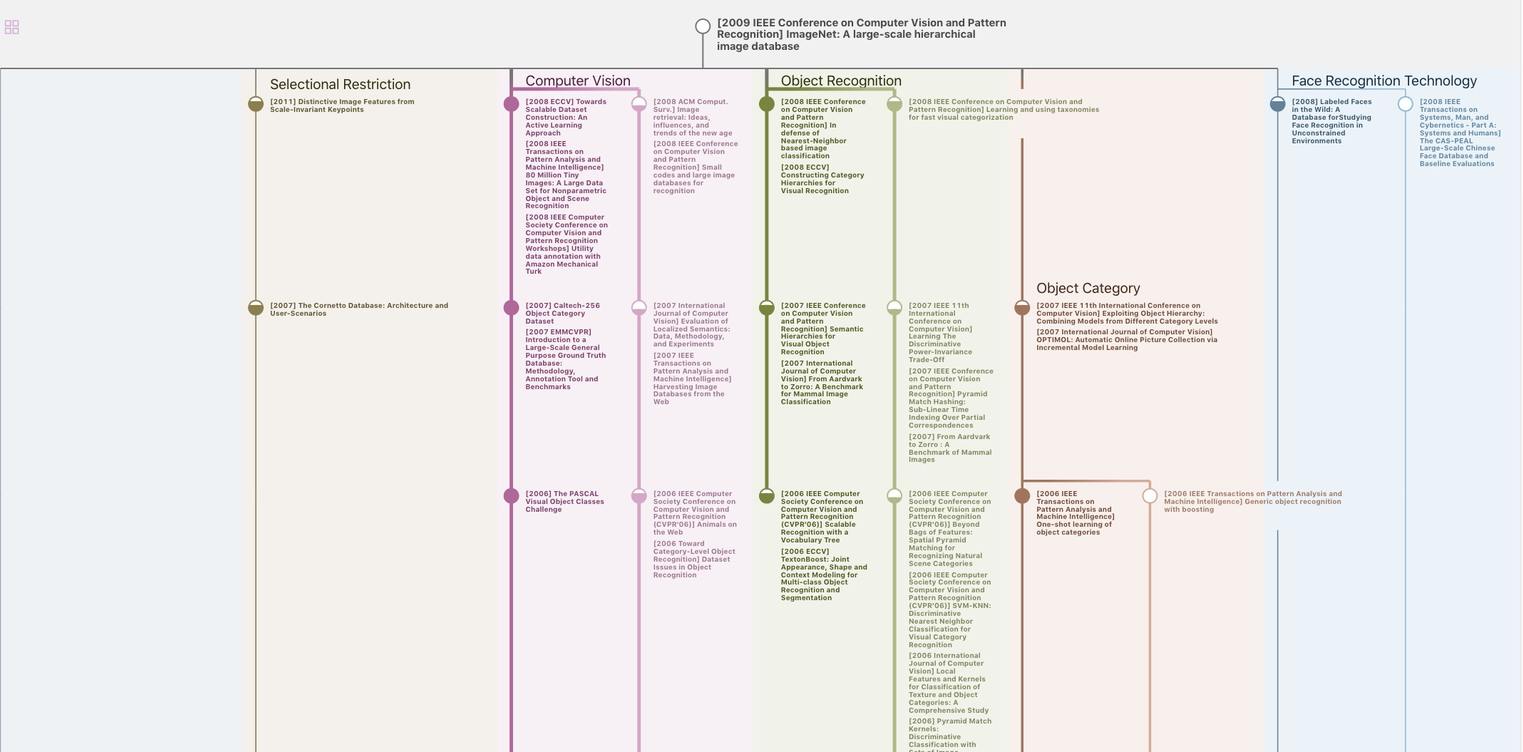
生成溯源树,研究论文发展脉络
Chat Paper
正在生成论文摘要