Evolving Error Feedback Fuzzy Model for Improved Robustness Under Measurement Noise
IEEE Transactions on Fuzzy Systems(2023)
摘要
In this article, we propose a new variant of evolving fuzzy model for regression problems, which is based on error feedback integration in order to compensate measurement noise and to achieve more robust predictions; thus, it is termed as evolving error feedback fuzzy model. Thereby, we define an autoregressive-based noise model, localized per rule for being able to model different possible noise behaviors in different parts of the input space. Its predictions are added to the real fuzzy model predictions for noise correction purposes. This additive aspect leads to an extended version of the recursive fuzzily weighted least squares estimator (RFWLS-N = RFWLS with noise compensation), inheriting all its favorable convergence properties. For rule antecedent learning, we perform an evolving clustering process with the integration of a new forgetting strategy to be able to achieve more flexible updates in order to account for possible drifts. Therefore, a specific sample weighting concept is designed, with which different forgetting types can be achieved, ranging from (slow) logarithmic forgetting to (uniformly) linear forgetting and to (fast) exponential forgetting. The integration of the weights into the updates of rule centers and inverse covariance matrices is underlined with a theoretical analysis of their sample-wise contributions in the updates. Results on several noise-affected real-world datasets and system identification problems showed significantly improved model performances, compared to related evolving fuzzy methods, to the conventional RFWLS estimator and to the recursive correntropy approach. Antecedent forgetting helped us to improve the error trends in the case of abrupt drifts, while it never worsened the trends in the case of no drift.
更多查看译文
关键词
Predictive models,Data models,Noise measurement,Measurement uncertainty,Adaptation models,Load modeling,Convergence,Antecedent forgetting,error feedback integrat- ion,evolving clustering,evolving fuzzy systems (EFSs),measur- ement noise,online data streams
AI 理解论文
溯源树
样例
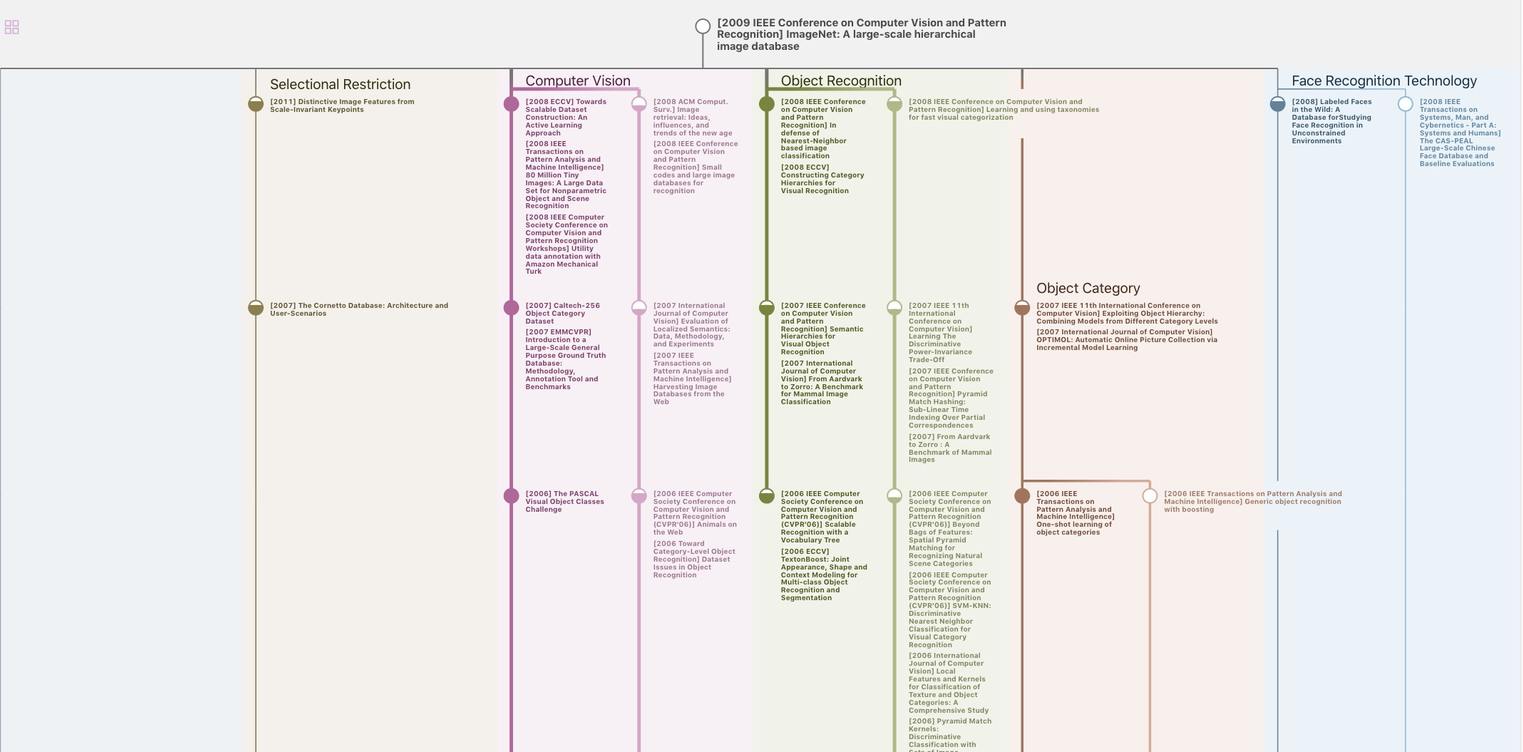
生成溯源树,研究论文发展脉络
Chat Paper
正在生成论文摘要