Bi-Directional BILSTM-Attention Short-Term Load Forecasting based on Correlation Weight
2022 IEEE 2nd International Conference on Electronic Technology, Communication and Information (ICETCI)(2022)
Abstract
In order to improve the short-term electric load forecasting accuracy, a Bi-directional Long Short-Term Memory (BILSTM) time series forecasting method combining similar day selection and correlation weights of the days to be forecasted is proposed. Firstly, K-means++ clustering is used to select the coarse set of similar days, and then gray correlation analysis is performed on the coarse set to determine the training set that is suitable with the day to be tested. Then, BILSTM-attention is used to perform cross-sectional prediction and longitudinal prediction respectively, and the weight of each collection point is calculated using the minimum variance method to derive the final prediction results. The anterior attention mechanism assigns the information weights so that the time-series data are emphasized according to the degree of performance, and the bidirectional long- and short-term memory neural network ensures that the data information is fully utilized. The periodicity of the data is investigated using the minimum variance method with full consideration of the influence of meteorological factors. The method is verified to improve the prediction accuracy by analyzing the electric load data collected in a domestic area.
MoreTranslated text
Key words
Short-term load forecasting,Correlation,Weight distribution,Similar day,The neural network
AI Read Science
Must-Reading Tree
Example
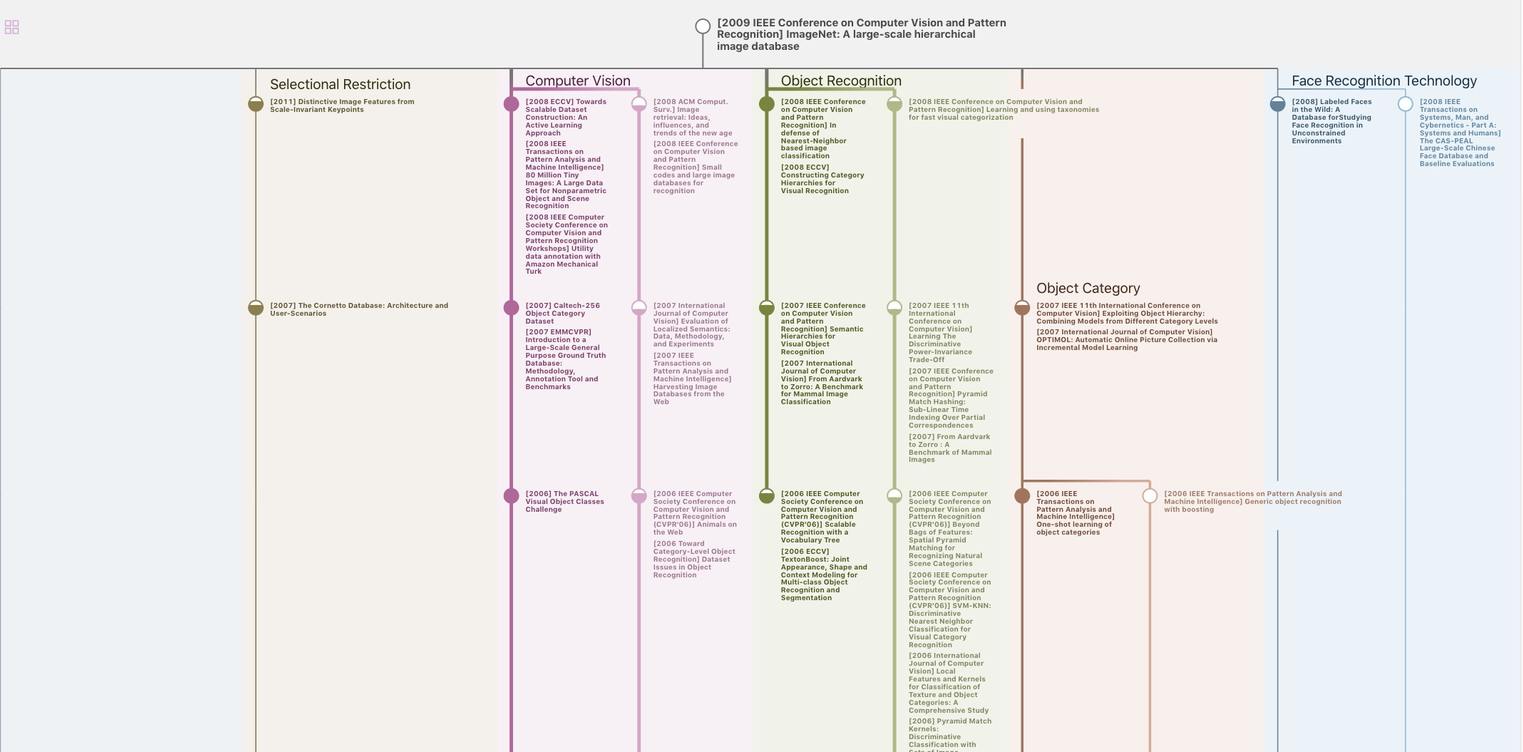
Generate MRT to find the research sequence of this paper
Chat Paper
Summary is being generated by the instructions you defined