Parameter Optimization of VLSI Placement Through Deep Reinforcement Learning
IEEE Transactions on Computer-Aided Design of Integrated Circuits and Systems(2023)
摘要
Critical to achieving power-performance-area goals, a human engineer typically spends a considerable amount of time tuning the multiple settings of a commercial placer. This article proposes a deep reinforcement learning (RL) framework to optimize the placement parameters of a commercial electronic design automation (EDA) tool. We build an autonomous agent that learns to tune parameters without human intervention and domain knowledge, trained solely by RL from self-search. To generalize to unseen netlists, we use a mixture of handcrafted features from graph topology theory and graph embeddings generated using unsupervised graph neural networks. Our RL algorithms are chosen to overcome the sparsity of data and latency of placement runs. As a result, our trained RL agent achieves up to 11% and 2.5% wire length improvements on unseen netlists compared with a human engineer and a state-ofthe-art tool auto-tuner in just one placement iteration (20x and 50x fewer iterations). In addition, the success of the RL agent is measured using a statistical test with theoretical guarantees and an optimized sample size.
更多查看译文
关键词
Deep learning,physical design,very-large-scale integration (VLSI) placement
AI 理解论文
溯源树
样例
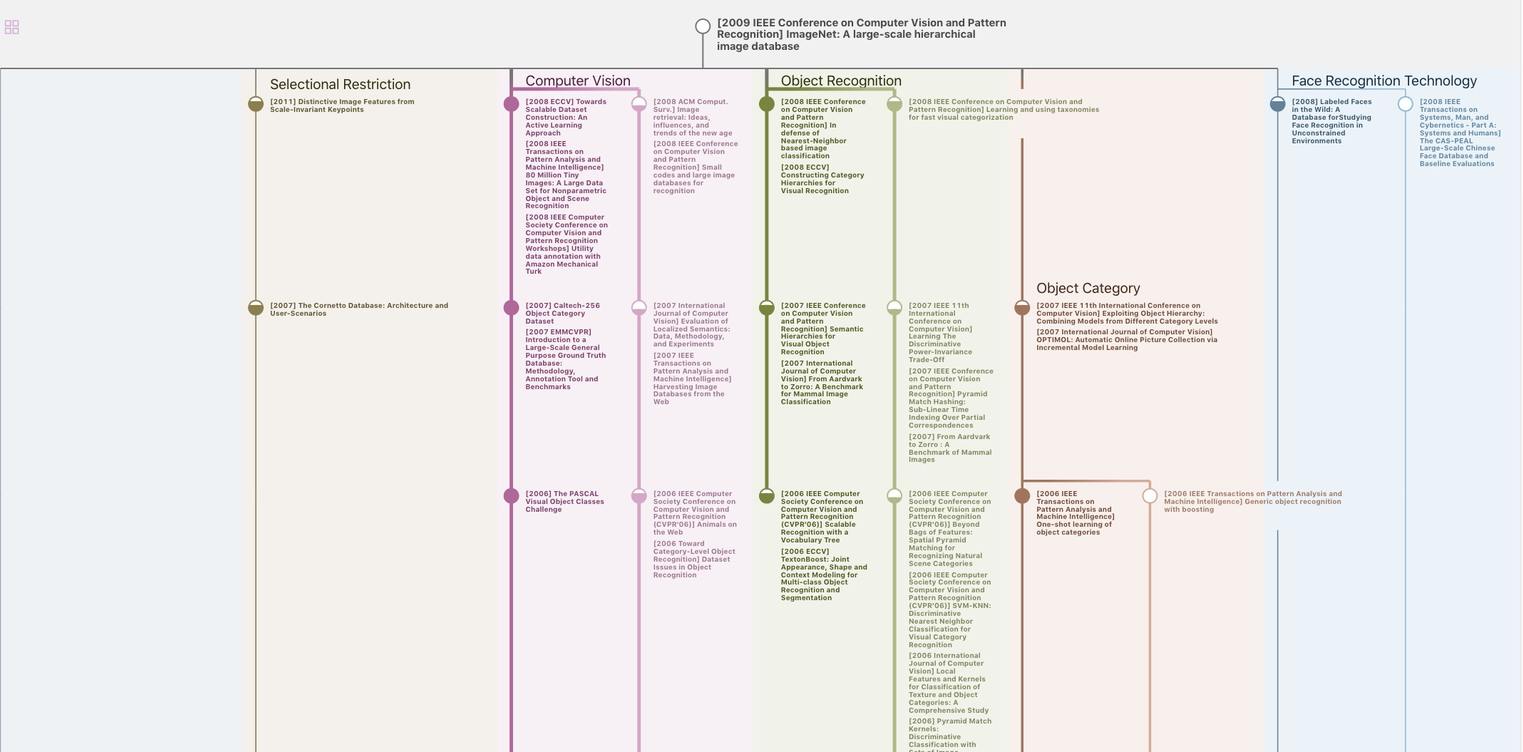
生成溯源树,研究论文发展脉络
Chat Paper
正在生成论文摘要