Unsupervised Hebbian Learning on Point Sets in StarCraft II
2022 International Joint Conference on Neural Networks (IJCNN)(2022)
摘要
Learning the evolution of real-time strategy (RTS) game is a challenging problem in artificial intelligent (AI) system. In this paper, we present a novel Hebbian learning method to extract the global feature of a point set in StarCraft II game units, and its application to predict the movement of the points. Our model includes encoder, LSTM, and decoder, and we train the encoder with the unsupervised learning method. We introduce the concept of neuron activity aware learning combined with k-Winner-Takes-All. The optimal value of neuron activity is mathematically derived, and experiments support the effectiveness of the concept over the downstream task. Our Hebbian learning rule benefits the prediction with lower loss compared to self-supervised learning. Also, our model significantly saves the computational cost such as activations and FLOPs compared to a frame-based approach.
更多查看译文
关键词
Point Set,Hebbian Learning,Winner-Takes-All,Long Short-Term Memory,Game AI
AI 理解论文
溯源树
样例
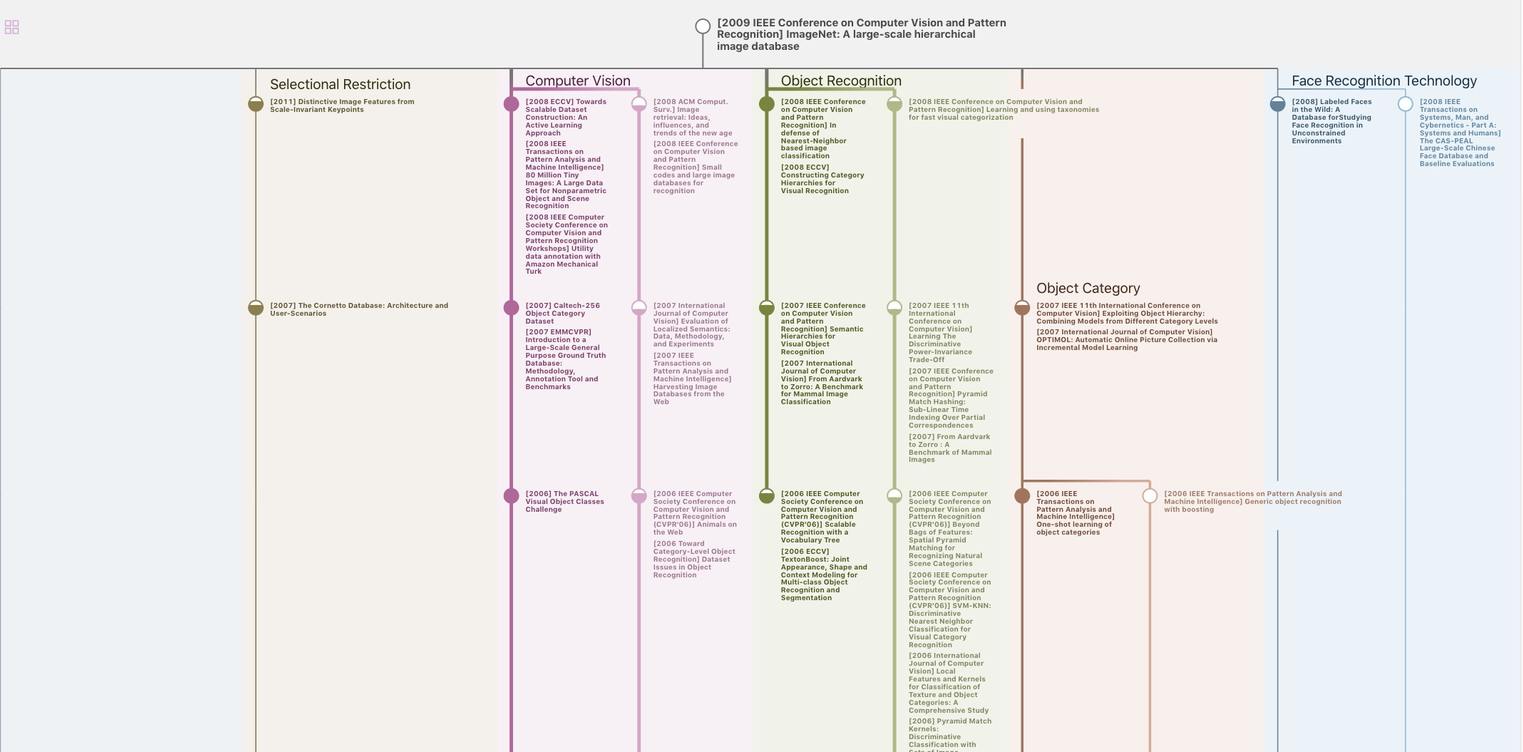
生成溯源树,研究论文发展脉络
Chat Paper
正在生成论文摘要