Machine-Learning Inspired Clustering of Distributed Energy Resources
arxiv(2022)
摘要
With the increasing penetration of distributed energy resources (DERs) in distribution grids, their impact on grid operations can no longer be ignored. However, the individual control of these increasingly ubiquitous devices remains a challenge due to their numbers. One solution is to control them in groups via virtual power plants. Previous work has typically focused on optimally dispatching a fixed set of DERs, eschewing methods for optimally determining set membership. Intuitively, these fixed sets may not be optimal from a control perspective. Here, we propose a method to cluster the DERs based on a proxy for their covariances, with the goal of minimising the maximum variance across all DER clusters. This method does not require the enumeration and evaluation of all DER combinations, which are required for brute force techniques. Simulation results show that while there is typically a loss in optimality, it is generally small. More importantly, computational tractability is greatly improved when compared to other methods, which require some form of enumeration and evaluation of the DER combinations.
更多查看译文
关键词
clustering,machine-learning machine-learning,energy
AI 理解论文
溯源树
样例
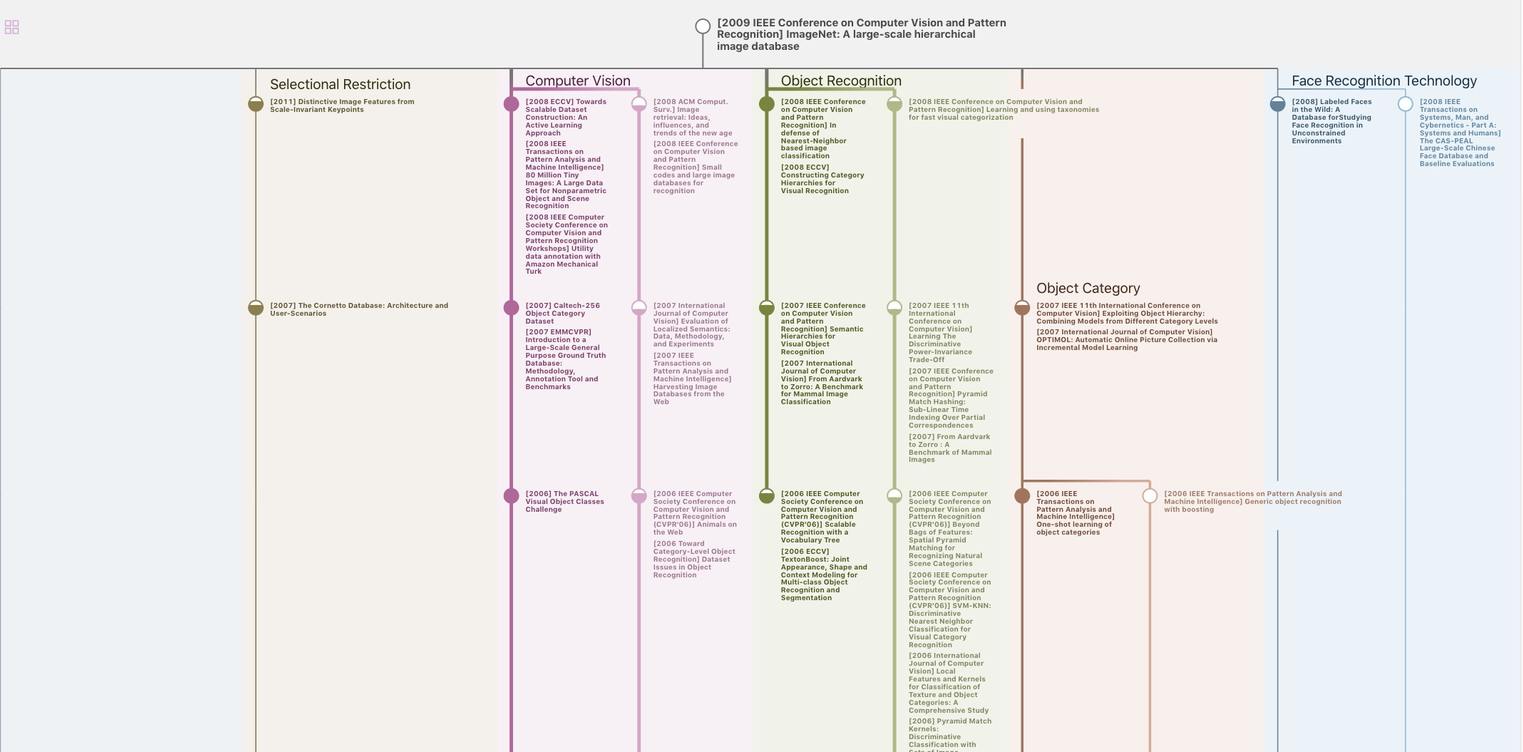
生成溯源树,研究论文发展脉络
Chat Paper
正在生成论文摘要