Toward Fairness in Speech Recognition: Discovery and mitigation of performance disparities
Conference of the International Speech Communication Association (INTERSPEECH)(2022)
摘要
As for other forms of AI, speech recognition has recently been examined with respect to performance disparities across different user cohorts. One approach to achieve fairness in speech recognition is to (1) identify speaker cohorts that suffer from subpar performance and (2) apply fairness mitigation measures targeting the cohorts discovered. In this paper, we report on initial findings with both discovery and mitigation of performance disparities using data from a product-scale AI assistant speech recognition system. We compare cohort discovery based on geographic and demographic information to a more scalable method that groups speakers without human labels, using speaker embedding technology. For fairness mitigation, we find that oversampling of underrepresented cohorts, as well as modeling speaker cohort membership by additional input variables, reduces the gap between top- and bottom-performing cohorts, without deteriorating overall recognition accuracy.
更多查看译文
关键词
speech recognition, performance fairness, cohort discovery
AI 理解论文
溯源树
样例
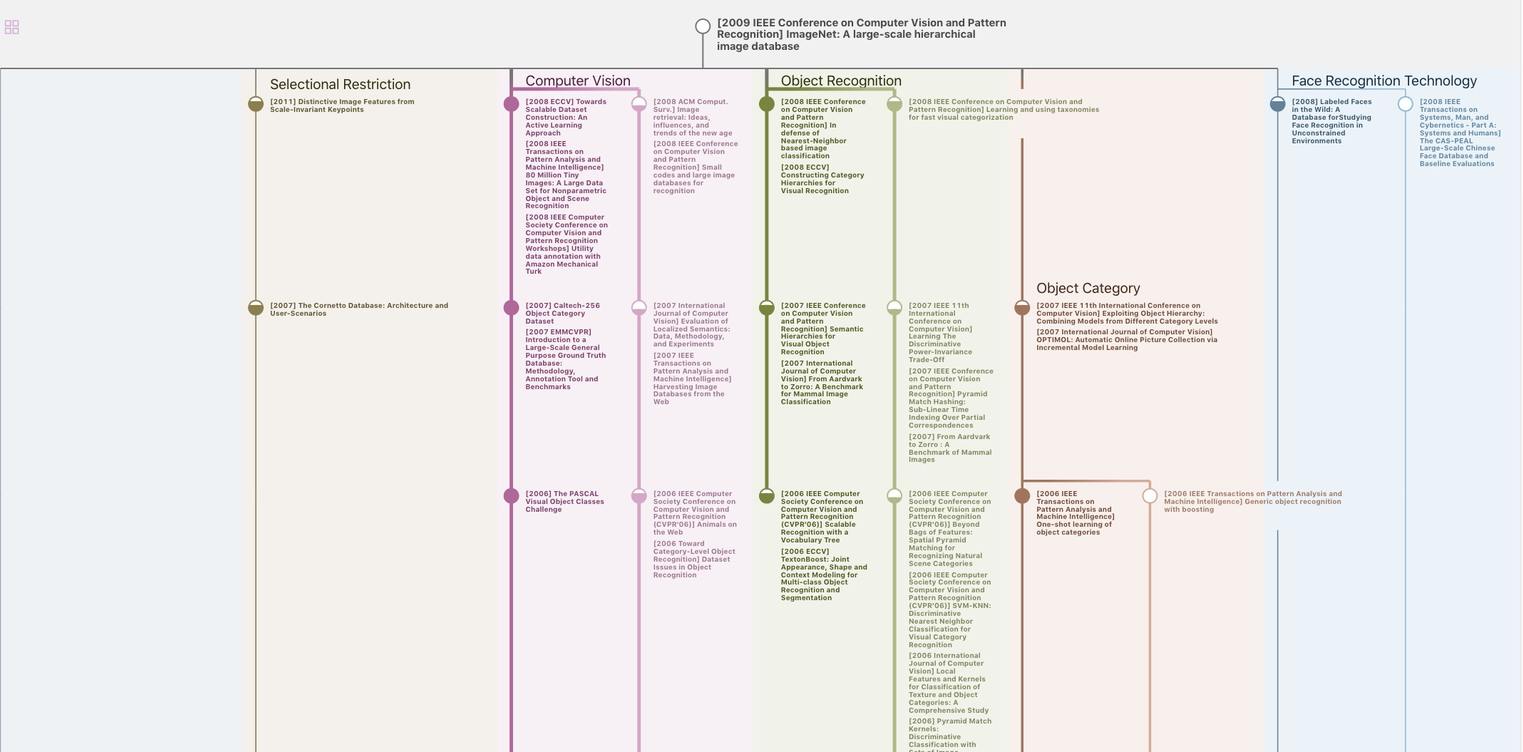
生成溯源树,研究论文发展脉络
Chat Paper
正在生成论文摘要